How does customer's product expertise moderate the usefulness of information recommendation agents in online stores?
Hyung Jun Ahn
School of Business, Hongik University, Seoul, Korea
Sangmoon Park
Department of Business Administration, Kangwon National University, Chuncheon city, Gangwon-do, Korea
Introduction
With the wide acceptance of online shopping, many Internet businesses have developed and deployed customer information aid tools often called recommendation agents (hereafter 'agents'). Agents are software programs that are usually embedded in Internet stores to help customers search and find products. Considering that there are a huge number of choices available on the Internet and that real-time human assistance is not usually available at online stores, agents are now regarded as an essential tool for making the purchasing process efficient and effective (Adomavicius and Tuzhilin 2005; Ahn 2006; Burke 2002; Kwon et al. 2009; Wang and Doong 2010; Xiao and Benbasat 2007).
Most agents consist of two key components: eliciting user preference and matching the preference with product profiles. For example, in a recommendation system used at Amazon.com, user preferences are implicitly collected whenever a user clicks on or purchases a product (Linden et al. 2003). This information is passed to an internal mechanism that finds other products that are most likely to be preferred and purchased by the user. Users may also explicitly enter preferences regarding, for example, desired price ranges, authors or key words.
There have been many studies about agents from various perspectives. Among them, technical studies have developed algorithms or mechanisms of recommendation that can more precisely reflect user preferences (Ahn 2008; Adomavicius and Tuzhilin 2005; Ahn 2006; Burke 2002). On the other hand, behavioural researchers in the information systems or marketing fields have studied factors that affect the adoption or effectiveness of recommendation agents. Studies of this type often conduct empirical investigation involving experiments with real or virtual Internet stores and questionnaires (Cohen and Fan 2000; Greco et al. 2004; Wang and Benbasat 2005; Xiao and Benbasat 2007). The present study falls into the second category.
Although previous studies have found some common factors influencing the usefulness or acceptance of agents, there have been relatively few studies about how the relationships can be moderated by user characteristics. The goal of this study is to investigate the relationship between the user evaluation of agents and its antecedents, particularly focusing on the moderating effect of customers' product expertise. This is believed to be a significant issue since the existence of such a moderating effect can require Internet stores to develop and apply different personalisation approaches to distinct types of customers. In other words, the result of this research can help businesses to better customise agents or use different agents for heterogeneous types of customers for more effective information recommendation. For the investigation, an experimental shopping mall for digital cameras was built and used for shopping experiments and data collection. The collected data were analysed using the partial least squares method.
The paper is organized as follows. The next section provides theoretical background of the research and explains the hypotheses. The third section introduces the experiments and data collection. The fourth section presents findings from the analysis. The final section concludes with a summary, implications for businesses, and limitations of the study.
Theoretical background and hypotheses development
Studies on recommendation agents
Similar to the definitions of recommendation agents found in literature (Adomavicius and Tuzhilin 2005; Burke 2002; Xiao and Benbasat 2007), this paper defines an agent as a software program embedded in an Internet store to help customers find products by providing guidance and/or recommending products. In this sense, previous studies have regarded agents as decision support systems for customers (Grenci and Todd 2002; Haubl and Trifts 2000; Kim et al. 2010).
Many studies have shown that agents help customers to make better, more efficient, purchasing decisions (Hostler et al. 2004; Pedersen 2000). Agents can significantly improve both decision process and decision outcome, especially when the choice set is huge and customers have limited ability and time in evaluating all the alternatives. For these reasons, agents are also believed to help businesses by enabling up-selling and cross-selling of products. Hence, agents are increasingly being regarded as an essential tool by many Internet stores.There have been several different classifications of agents. The most common classification is based on whether recommendation is made using content similarities (content-based filtering) or user similarities (collaborative filtering) (Cohen and Fan 2000; Greco et al. 2004; Kim and Kim 2001; Li et al. 2005). There are many variations to these recommendation methods such as item-based collaborative filtering where item similarity is used instead of user similarity. Another popular scheme of classification is based on whether the inputs to an agent are at the feature level or the needs level (Felix et al. 2001; Komiak and Benbasat 2006; Stolze and Nart 2004). The feature-based agents require selection of product features by customers to generate recommendations; the needs-based agents produce recommendations based on the specific type of needs chosen by a user such as, for example, a camera for family or a camera for sports. This paper adopts the latter typology and provides the two different types of agent for experimental shopping. Still more classifications of agents are available in literature (Adomavicius and Tuzhilin 2005; Burke 2002; Xiao and Benbasat 2007).
Hypotheses development
Despite the abundance of literature on the factors that determine the users' acceptance or evaluation of agents, there have not been many studies investigating the moderating role of personal characteristics, especially customers' product expertise. Based on a literature review, this sub-section develops the hypotheses for our research goal. We introduce the antecedents, the moderating variables, and the dependent variables for our suggested model of users' evaluation of agents. The hypotheses are largely based on empirical findings in related studies as well as theories such as task-technology fit, technology acceptance model and consumer decision-making.
Antecedent variables: intention specificity, agent-intention fit, familiarity with agents, and online shopping frequency
Some studies show the influence of the type of agent on the effectiveness or users' evaluation of agents (Felix et al. 2001; Komiak and Benbasat 2006; Stolze and Nart 2004). The general conclusion from the studies is that some agents are preferred or perceived to be more effective by users. For example, the empirical study by Felix et al. (2001) shows that needs-based agents are evaluated to be more effective by the participants of the experiment for purchasing digital cameras. Also, in the review by Xiao and Benbasat (2007), several propositions were presented that claim the superiority of one type of agent over others in terms of users' decision quality and decision effort.
However, the preference for a specific type of agent may vary according to the intention of a user. For example, a user who has a very specific shopping goal may know the desired attributes of the target product very well, in which case a feature-based agent can be more suitable. The user can input values or select options for the features to generate recommendations easily. On the other hand, if the intention of a user is ambiguous or the user is not sure of the specific features of the desired product, a needs-based agent can be more helpful. The task-technology fit theory also supports this idea and many studies have tested the theory in the information systems field (Gebauer and Tang 2008; Klopping and McKinney 2004). For instance, in Klopping and McKinney's study (2004), the theory was found to have a significant effect on the perceived usefulness of an e-commerce system. Therefore, it is proposed that:
H1: Higher perceived fit between a user's shopping intention and the type of agent positively impacts the evaluation of the agent.
In addition to the above, the specificity of a user's intention can alone have an impact on the relationship. When users have a specific goal and know what type of products to search for, they may just browse through the categories in the Internet store to find the products. For example, most online digital camera shops provide browsing categories that correspond to different numbers of pixels, zoom capabilities, brands and price ranges, together with some sorting functionality for each category, making it easy to find products once users know what to search for. Empirical studies on the acceptance of e-commerce or Internet services also support this. For example, in the study by Kim et al. (2008) the acceptance of e-commerce by users is shown to be affected by the equivocality of online tasks. In the work of Jarvelainen (2007), task ambiguity was found to have negative impact on the acceptance of online booking for passenger cruise services. In and experiment by Tam et al. (2006), more specific goals lead users to react more sensitively to advertisements. Therefore, it is proposed that:
H2: Higher perceived specificity of a user's shopping intention has a negative impact on the evaluation of the agent.
We also include variables that have been found often to affect the relationship in the literature on agents: frequency of user's online shopping experience (Castaneda et al. 2007; Jarvelainen 2007; Kim et al. 2008; Klopping and McKinney 2004; Stern et al. 2008) and user's familiarity with agents in general (Komiak and Benbasat 2006; Stern et al. 2008).
H3: Higher frequency of online shopping of a user has positive impact on the evaluation of the agent.
H4: Higher familiarity of a user with agents in general has positive impact on the evaluation of the agent.
Product expertise as a moderator
There have been studies about the effect of customer's product expertise, or category expertise, on the preference of agents or acceptance of recommendation results. As high expertise groups are more capable of selecting products themselves based on their knowledge of the products, they can rely less on recommendations from agents (Kramer 2007; Urban et al. 1999). In addition to this, higher expertise groups have also been found to favour agents that are feature-based, attribute-oriented, or use content-based filtering compared with needs-based, benefit-oriented, or collaborating-filtering methods (Pereira 2000; Rathnam 2005; Xiao and Benbasat 2007; Su et al. 2008). This is because the former types of agent give more control to customers allowing them to specify or choose detailed features of products so that they can pinpoint the desired products. The latter types of agent can be more relevant for low expertise users because they do not need detailed knowledge about product attributes, but can just request recommendations based on their broad needs.
We extend and apply the above insights about product expertise to the other relationships in our model to test its moderating role. For a high product expertise group, agent-intention fit may be less important because they rely less on an agent for shopping; in contrast, for a low product expertise group, agent-intention fit can be more significant because they are more likely to need the help of agent recommendations (Kramer 2007; Urban et al. 1999; Xiao and Benbasat 2007). Similarly, the effect of intention specificity can also be moderated. Given a broad, non-specific shopping goal, a low product expertise group may rely more on recommendations from an agent, whereas a high expertise group need not be so reliant (Kramer 2007; Urban et al. 1999; Xiao and Benbasat 2007). Next, agents can be easier to use for a high expertise group if those users are familiar with agents in general. In contrast, low expertise groups can still find it more difficult because of their limited knowledge of the products. Finally, online shopping frequency can also impact the two groups differently. High expertise groups with enough shopping frequency can find agents unnecessary because they can browse through the categories and find the products without the help of agents. Low expertise groups may nevertheless need the guidance of agents.
Therefore, it is proposed that:
H5: Customer's product expertise moderates the influence of the antecedent variables on the evaluation of an agent.
User's evaluation of an agent and the effectiveness of decisions
In the extensive review by Xiao and Benbasat (2007), it was found that two groups of constructs usually appear as outcome variables of agent use: consumer decision making and user evaluation of agents. The first group represents how the process and result of decision making are affected by use of agents, while the latter represents users' own direct evaluation of agents. This research also uses similar variables as dependent variables.
Basically the technology acceptance model was adopted and modified for this study. This model has been used widely in studies on the acceptance of many different types of information systems (Park et al. 2009; Stern et al. 2008; Van Dijk et al. 2008; Wann-Yih and Chia-Ying 2007). Recently, some studies on agents have also adopted variables related to the model (Wang and Benbasat 2005).
The original model has three variables: perceived ease of use, perceived usefulness, and acceptance of the system. Following the approaches in similar studies (Kowatsch and Maass 2010; Wang and Benbasat 2005), these three variables and their relationship were adopted as a whole for users' evaluation of agents. The first two variables, ease of use and usefulness, were directly adopted. Hypothesis 6 is also from the original model, which states the influence of perceived ease of use on perceived usefulness. Next, unlike many studies that use this model in an organizational management information systems context, this study focuses on the purchasing decision of consumers. Hence, rather than using the original variable about the acceptance of a system, we took the approach by Kamis and Davern (2004) to include a variable about the effectiveness of decisions. That is, if an agent is perceived to be easier to use, it can make the purchase decision more effective by allowing better utilisation; similarly, if an agent is perceived to be more useful, it leads to a more effective purchase decision.
H6: The perceived ease of use of an agent has positive impact on the perceived usefulness of the agent.
H7: The perceived ease of use of an agent has positive impact on the perceived effectiveness of purchase decision.
H8: The perceived usefulness of an agent has positive impact on the perceived effectiveness of purchase decision.
See Table 1 for the summary of the constructs.
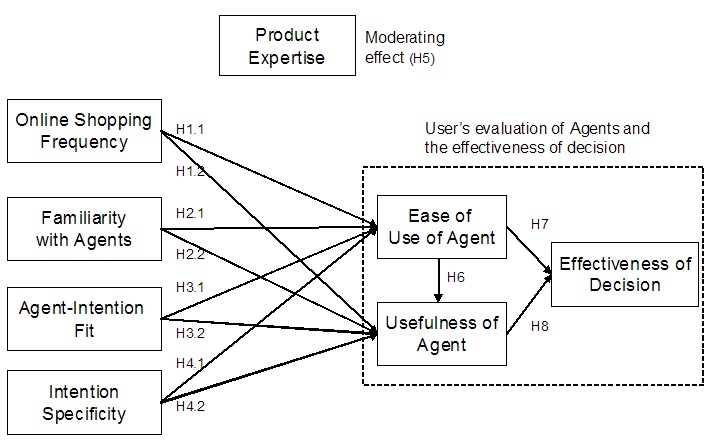
Figure 1: The conceptual model
Research method
Experiment
An experimental online store for digital cameras was built for collecting data to test the proposed model (Figure 2). The shop has one hundred cameras from eleven brands, ranging from low-price compact products to high-end professional cameras. Users can browse the shop according to three attribute categories: brands, number of pixels, and price ranges. Users can find products and put as many products as they want into the shopping cart before they finish the experiment.
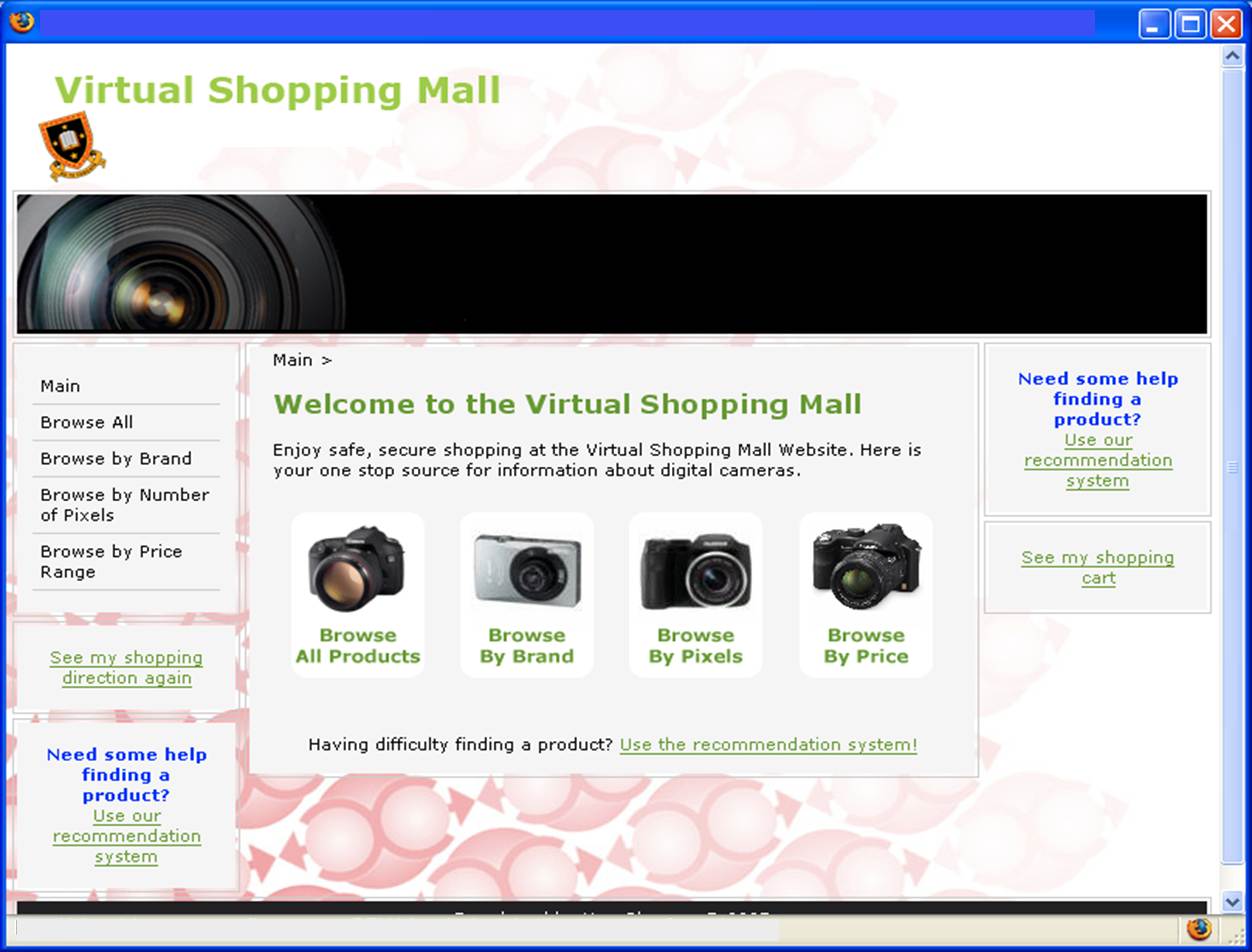
Figure 2: The main screen of the experimental store
The online store has two different types of agent: feature-based and needs-based. The feature-based one, shown on the left-hand side of Figure 3, allows users to select features of a desired camera and recommends cameras that match the selection. The results are ordered by average customer ratings. We used the ratings data available from www.dpreview.com at the time of the experiment for the sorting. The needs-based agent is also shown on the right-hand side of Figure 3. It presents users with a list of typical consumer needs of cameras so that users can select one in the list for the given shopping goal. The list was also adopted from an online shop (www.imaging-resource.com) at the time of the experiment. It also provides a list of three basic features so that users can narrow down their search by selecting the values of the basic features. During the experiment, only one of the two agents is randomly assigned to each participant so that about half the users could use the feature-based one, and the other half the needs-based one.
Each user is also randomly given a shopping task of either buying a camera that is most similar to a specific product, or buying a camera for a family member (Figure 4). The former is intended to provide participants with a specific goal, whereas the latter is somewhat ambiguous, while still providing a realistic shopping purpose.
This way, each user belongs to one of the four agent, shopping task combinations. Note, however, that this study is not assuming any definite fit between a specific agent and a specific shopping task. The reason for providing two agents and two tasks is mainly to create diversity in the variables so that the participants can have varying levels of perception about such variables as agent-intention fit and intention specificity. Using definite fits for studies like this is very hard because the perceived fit by each user can be different despite being given the same agent and task. Therefore, this study also measures the perceived fit rather than using fixed fits as has been done in previous studies (Larsen et al. 2009; Lin and Huang 2008).
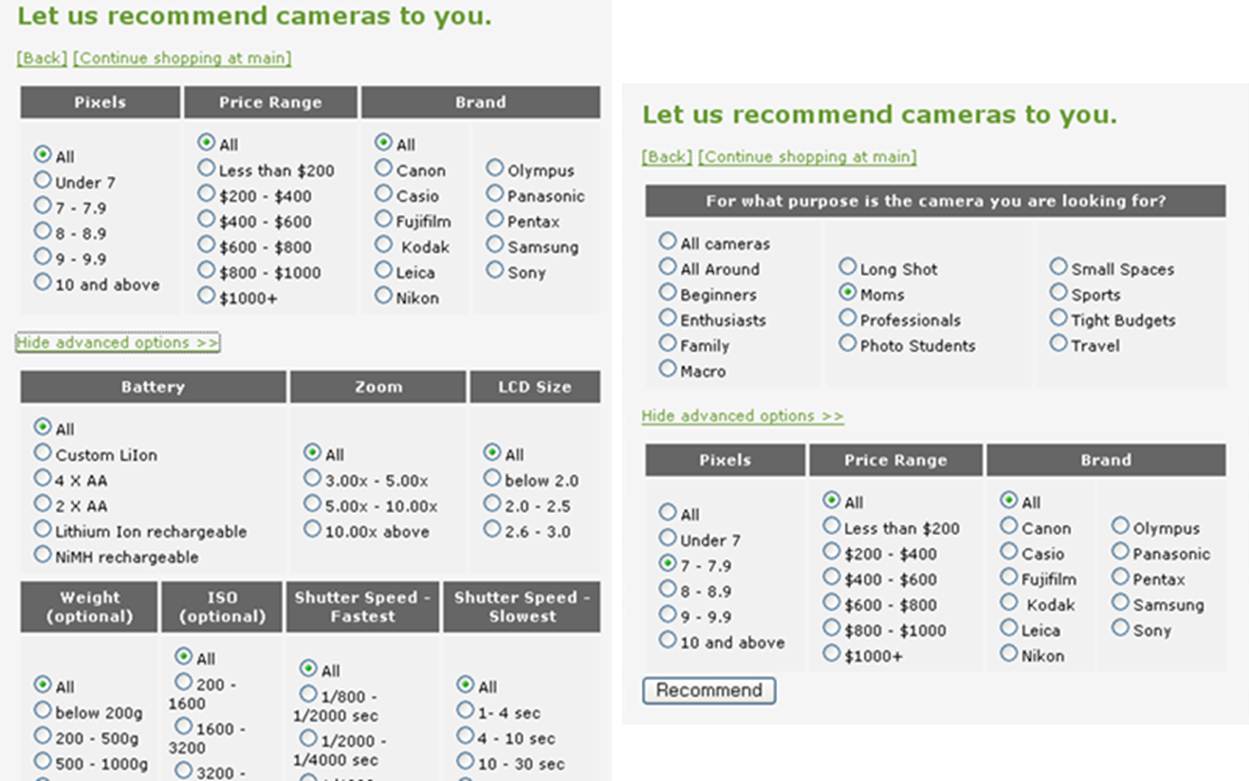
Figure 3: Feature-based (left) versus needs-based (right) agents
Participants were instructed to put as many products as they like into the shopping cart, but they had to choose two as their final choice at the end of the experiment. The reason for asking participants to purchase two products was to encourage them to use and experience the system, and the agents assigned to them, more extensively.
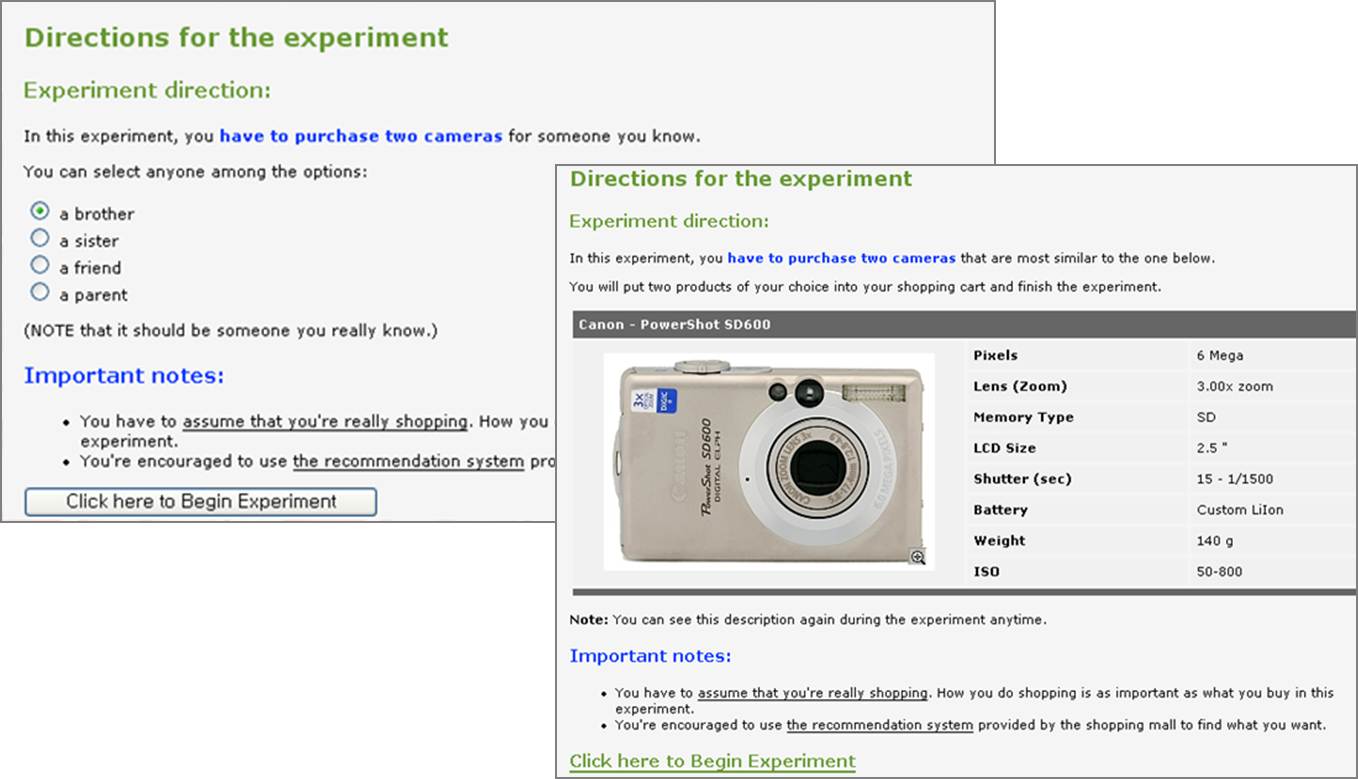
Figure 4: Two different shopping goals given to users randomly
Data from the experiment
Before the experiment, a pilot test was performed involving about twenty graduate and undergraduate students at a management school in an English speaking country to find and fix possible problems of the experiment settings. The actual experiment was performed with 220 undergraduate students at the same school taking a particular compulsory course. A tutor instructed the participants on the overview of the experiment and how to use the system. Any questions from the students regarding the task were also answered prior to the experiments. Students were given marks of about 1 percent of the course for the participation. No other compensation was given to the participants.
Although the use of student samples is sometimes questioned, the use of student subjects in this study can be justified as follows. First, online consumers are known to be generally younger and to have a higher education level, which is similar to the characteristics of university students (McKnight et al. 2002). For this reason, many other studies published in the e-commerce field have used students for experiments or survey (Ahuja et al. 2003; Calisir et al. 2010; Choi and Lee 2003; Constantinides et al. 2010; Hasan 2010; McKnight et al. 2002). It has also been reported that research in the e-commerce field shows similar results between student samples and non-student samples (Ahuja et al. 2003). Moreover, the experiment in this study does not require the students to be in an unrealistic or unfamiliar setting. Rather, the participants are just asked to conduct a virtual online shopping in a way very similar to real e-commerce.
Out of the total 220 participants, 138 who used the given agent at least once were selected for the analysis. Others were excluded because they either finished the experiment without the help of agent, just browsing the categories, or submitted unusable values to some of the questions in the questionnaire. The reason why some of the participants did not use the agents is because the experiment did not force all the participants to use the agents, which was to provide a realistic online shopping environment. Otherwise, the experiment could have imposed unrealistic constraints on the participants. Pearson's chi-squared test was conducted on both the included and the excluded samples to see whether a specific task-agent combination caused the users to avoid using the agents or not finish the experiment properly. The results for the 138 included samples showed no significant difference (p=0.224), where the proportion of the participants ranged from 20% to 30% for each of the four combinations. The results for the 82 excluded samples were also similar, showing no significant difference (p=0.278), with the proportion of each combination ranging from 20% to 30%.
Measures
The measures used by this study are listed in Table 1. The existing validated measures were used where available (see Appendix 1 for individual instruments). All the variables were measured using an online questionnaire that followed each individual shopping experiment. The data were split into two groups of high and low product expertise respectively, based on the median value of the item average of the construct. This led to seventy-four users of low expertise and sixty-four of high, where the inequality in the two groups was caused by tie values among some users.
Variable | Definition |
---|---|
1. Intention specificity | The degree to which a user perceives a shopping goal is specific. |
2. Agent-intention fit | The degree to which a user perceives a given agent is suitable for the given shopping intention compared with the other agent. |
3. Familiarity with agents | How familiar a user is with agents in general. |
4. Online shopping frequency | How frequently a user shops online. |
5. Ease-of-use of agents | How easy a user perceives the agent to be to use. |
6. Usefulness of agents | How useful a user perceives the agent to be. |
7. Effectiveness of decision | How a user perceives about the effectiveness of the final purchase decision |
8. Product expertise | How well a user knows about the products |
Method
This study used the partial least squares method for analysing the data. Similar to LISREL (a program for LInear Structural RELations), partial least squares is a popular method for analysing causal relationships and was chosen for the following reasons: first, unlike LISREL, it can be used reliably for relatively small data samples (Barclay et al. 1995). Secondly, it does not require strict normality of data for analysis unlike many other methods (Wold 1985). Thirdly, it can combine regression and factor analysis within the same statistical procedure: this allows the test of a model and the generation of reliability measures simultaneously, which makes it easy to apply the method to complicated causal models (Barclay et al. 1995; Hulland 1999). Finally, it has been widely and successfully used in the e-commerce and management information systems field (Goodhue et al. 2006; Marcoulides et al. 2009), as well as other fields in business studies (Fornell and Bookstein 1982; Hulland 1999).
Measurement results: reliability and validity
In many applications of partial least squares in management studies, reliability is usually checked with the loadings of the measures with their associated constructs (Hulland 1999). It is generally accepted that individual measures are statistically reliable if the loadings of them are larger than 0.7. In other words, loadings of a measure above the threshold are considered to show enough variance is accounted for by the respective constructs. In Table A2 of Appendix 2, it is shown that all the measures have loadings greater than 0.7.
In addition to the reliability of the individual measures, it is important to check whether the measures of each construct together show convergent validity. Two measures of convergent validity are often used: Cronbach's alpha and composite reliability (Hulland 1999). Again the threshold value 0.7 is generally accepted for both alpha and composite reliability. It can be seen from Table A3 of Appendix 2 that all the constructs satisfy these requirements.
Next, it is important to assure that the measures of each construct are different from the measures of the other constructs, which requires the test of discriminant validity. In studies using partial least squares, average variance extracted (i.e., the correlation between a construct and its measures) is often used for the test (Fornell and Bookstein 1982). Simply, the square root of the average variance of each construct needs to be greater than the correlation between the construct and any other. In Table A3 of Appendix 2, it is observed that the square root values (the diagonal elements in the correlation matrix) of all the constructs exceed the correlation values of the other constructs (the off-diagonal elements), showing strong discriminant validity.
Data analysis and results
Full model (hypotheses 1, 2, 3, 4, 6, 7, and 8)
Table 2 summarises the findings of the analysis. First, the analysis of the full model showed partial support for the hypotheses. The effects of online shopping frequency appeared to be non-significant on its dependent variables. Also, agent-intention fit, agent familiarity, and intention specificity all appeared to have no significant influence on the perceived usefulness of agent. While the perceived ease of use turns out to be significant on the decision effectiveness, the perceived usefulness of agent does not.
Full model (n=138) | Moderated models (H5) | Difference in path coefficients between subgroups (t-value) | |||||
---|---|---|---|---|---|---|---|
High expertise group (n=64) | Low expertise group (n=74) | ||||||
Path coefficient | t-value | Path coefficient | t-value | Path coefficient | t-value | ||
[H1.1] Online shopping freq. - usefulness of agent | -0.01 | -0.09 | 0.11 | 1.29 | -0.05 | -0.56 | 0.15 (1.29) |
[H1.2] Online shopping freq. - ease of use of agent | -0.15 | -1.55 | -0.44 | -3.77† | 0.03 | 0.26 | -0.47 (-2.88**) |
[H1.2] Online shopping freq. - ease of use of agent | -0.15 | -1.55 | -0.44 | -3.77† | 0.03 | 0.26 | -0.47 (-2.88**) |
[H2.1] Familiarity with agents - ease of use of agent | 0.26 | 2.99** | 0.37 | 2.97** | 0.20 | 1.45 | 0.17 (0.90) |
[H2.2] Familiarity with agents - usefulness of agent | 0.14 | 2.09* | 0.14 | 1.23 | 0.14 | 1.69 | 0.01 (0.04) |
[H3.1] Agent-intention fit - ease of use of agent | -0.25 | -3.41† | -0.25 | 2.65** | -0.28 | -2.28* | 0.03 (0.17) |
[H3.2] Agent-intention fit - usefulness of agent | -0.06 | -1.01 | -0.02 | 0.14 | -0.08 | -1.06 | 0.07 (0.52) |
[H4.1] Intention specificity - ease of use of agent | 0.28 | 3.67† | .18 | 1.19 | 0.28 | 2.48* | -0.11 (-0.60) |
[H4.2] Intention specificity - usefulness of agent | -0.06 | -1.13 | -0.04 | 0.32 | -0.05 | -0.89 | 0.02 (0.13) |
[H6] Ease of use of agent - usefulness of agent | 0.77 | 12.84† | 0.77 | 7.08† | 0.80 | 10.98† | -0.03 (-0.24) |
[H7] Ease of use of agent - decision effectiveness | 0.59 | 5.14† | 0.61 | 3.13** | 0.46 | 3.44† | 0.17 (0.72) |
[H8] Usefulness of agent - decision effectiveness | 0.06 | 0.52 | -0.10 | -0.52 | 0.28 | 2.09* | -0.38 (-1.64) |
Overall, we can observe that agent familiarity and intention specificity have the most impact on the ease of use, and the ease of use leads to decision effectiveness, while the usefulness of agent may not. Simply put, the results show that when the users perceive their intention as being more specific, and are more familiar with an agent, they find it easier to use and this leads to more effective purchase decisions.
Moderated models (hypothesis 5)
To assess the moderating effect of product expertise on the relationships, further PLS analyses were performed for the two sub-groups of high and low product expertise. The result is also summarised in Table 2 which shows whether the statistical significance of a relationship differs between the two groups. Also, we can check whether the differences in the two path coefficients of a relationship in the two groups are statistically significant (see the t-values in the last column).
Table 2 shows that product expertise does moderate four of the relationships (see the rows that have values in bold in the columns of the moderated models). First, agent familiarity appeared to have significant influence on the ease of use of agent only for the high expertise group. That is the high expertise group appeared to find the agents easier to use as they get more familiar with agents, whereas the low expertise group did not. It can be interpreted that the limited product knowledge of the low expertise group makes it difficult for the group to adapt to the recommendation agents of cameras regardless of their general knowledge about agents. Second, the results show that more specific intentions make the agent easier to use for the low expertise group, while it does not for the other group, which is consistent with what was expected. Third, online shopping frequency appeared to have negative significant influence on the perceived ease of use of agent for the high expertise group, while not being significant in the other group. This is also consistent with the expectation that the high expertise group with better online shopping skills can easily find the desired products without the help of the given agent. In contrast, shopping frequency had no influence on the other group with lower product knowledge. Finally, the relationship between the perceived usefulness of the agents and the decision effectiveness is also being moderated, where it is significant only for the low expertise group. That is, for the high expertise group, a useful agent may not necessarily lead to an improved purchase decision, while it can for the other group. The other relationships appeared not to be moderated by product expertise.
The last column of Table 2 shows the results of the t-tests regarding the significance of the difference between the path coefficients of the two moderated models. Only the fourth relationship showed significant difference, suggesting a stronger moderating effect of product expertise on this relationship.
The above results suggest that product expertise plays an important role in explaining when and what type of users perceive agents to be easy to use and helpful for improving decision effectiveness.
Discussion and conclusion
Summary
The main goal of this study was to investigate the moderating role of product expertise on the relationship between users' evaluation of information recommendation agents and its antecedents. We hypothesised that product expertise moderates the relationship such that high and low expertise groups will perceive the influence of the independent variables differently. An experimental shopping mall of digital cameras was constructed to conduct a virtual shopping experiment for investigating the hypotheses. Two agents and two shopping tasks were prepared to create diversity in the experiment variables. Participants were randomly assigned one of the agents and one of the tasks.
The empirical investigation and analysis of the experiment showed that product expertise does moderate some of the impacts of agent familiarity, intention specificity, and online shopping frequency on the ease of use of agents. Agent familiarity and online shopping frequency influenced the ease of use only in the high expertise group. On the other hand, intention specificity affected the perceived ease of use only for the low expertise group. The influence of agent-intention fit on the perceived ease of use of the agent appeared not to be moderated by product expertise. Also, it was found that the impacts of the independent variables on the perceived usefulness of agents are not moderated by product expertise.
The main contribution of this research can be summarised as follows. First, there have been only a limited number of studies on the role of product expertise in the broad field of research on agents. Thus, the results of this research contribute to the advancement of our understanding of how different users with different product expertise may find the usefulness of various agents. Second, more specifically, no study so far has studied the moderating role of product expertise on the influence of intention specificity and agent-intention fit on users' evaluation of agents. The result of the analysis showed that users with different product expertise can react to agents differently when the specificity of shopping intention varies.
Implications for research and practice
First, we could clearly see that users with different characteristics or intentions perceive the ease of use of the given agents differently. Although this research just used two types of agents, there are many others such as those based on past purchase history or those that recommend products based on clicks. Moreover the research suggests that many of the users may find other methods of searching for products more effective than agents, for example, different listing or categorisation of products. Therefore, businesses need to understand that a single agent cannot make online shopping effective for everyone, and should consider providing multiple customer-aid information functions that can cater for different user characteristics and shopping goals (Ahn 2010).
Secondly, in line with the above, research is also needed on how online stores can effectively provide multiple recommendation functions. At most online stores, it is currently unusual to see many recommendation functions being provided. Academic research on the problem is also hard to find. Therefore, there exists a challenging and novel research problem of finding out how different types of customers can be identified correctly, and how different recommendation functions can be provided to users, distinctively and dynamically, without causing confusion to users. For example, further research is needed to find ways to identify whether a customer is an expert or not in digital cameras, and to lead that customer to the proper information recommendation function so that the whole shopping experience can be improved. The problem can be even more complicated for stores with diverse products because an expert in one product may not be knowledgeable about others.
Thirdly, more research is needed on the moderating relationship between the effectiveness of recommendation agents and its antecedents. There exist more variables for customers and products that may possibly moderate the relationship such as, for example, time spent on research before shopping and product complexity (Wang and Benbasat 2007). Hence, as more studies are conducted, we can expect to develop an integrated framework for online stores that leads to a proper selection and mix of shopping aid agents according to the characteristics of the stores and users.
Limitations of the study
This study has several limitations. First, because there is a huge variety of agents and many different ways of building them, one can not expect to produce exactly the same result from the present model when it is applied to different types of agent or different implementations. Second, we used only perceptive measures for the constructs. Experiments that involve direct measures can possibly yield more precise results. Third, the experiment was conducted with a single product type, digital camera. Therefore, care needs to be taken for the generalisation of the results.
Acknowledgement
This work was supported by 2010 Hongik University Research Fund, together with the Contestable Research Fund of Waikato Management School, New Zealand.
About the authors
Hyung Jun Ahn is an Associate Professor at School of Business, Hongik University, Korea. He received his PhD in Management Information Systems from Korea Advanced Institute of Science and Technology. Before joining Hongik University, he worked as a Senior Lecturer at Waikato Management School, New Zealand. He can be contacted at: hjahn@hongik.ac.kr
Sangmoon Park is an Associate Professor at Department of Business Administration, Kangwon National University, Korea. He received his PhD in Entrepreneurship and Innovation from Korea Advanced Institute of Science and Technology. Before joining Kangwon National University, he served as a manager at Samsung Corporate Research Centre. He can be contacted at: venture@kangwon.ac.kr