Mapping open science research using a keyword bibliographic coupling analysis network
Jae Yun Lee, and EunKyung Chung
Introduction: The open science movement has grown rapidly since the mid-2010s, and research has been conducted in various disciplines such as public health, medicine, education, and computer science. Research results have mainly been published in the journals of information science, computer science, and multidisciplinary fields.
Method: To identify the intellectual structure of open science, we constructed a keyword bibliographic coupling analysis network. We examined a total of 1,000 articles on open science from the Web of Science, extracting and analysing 4,645 keywords. Then, we implemented and visualised the keyword bibliographic coupling network by constructing a keyword dataset and a reference dataset for each keyword.
Results: By analysing the backbone keywords and clusters in the network, the study revealed that the most prominent keywords were open access, open data, and reproducibility. The analysis also uncovered nine clusters in open science research: open access, reproducibility, data sharing, preregistrations and registered reports, research data, open peer review, tools and platforms for reproducible research, open innovation, science policy, and preprints. These results indicated that open science research focuses on transparency and reproducibility. Additionally, it is noteworthy that this study revealed a considerable focus on the open innovation and science policy areas, which have not received much attention in previous studies.
Conclusions: The findings can help to understand the landscape of open science research and may guide research funding institutes and research policymakers to design their policies to improve the open science scholarly environment.
DOI: https://doi.org/10.47989/irpaper949
Introduction
Open science is an important topic in a wide variety of academic communities and is rapidly changing how scholarly communities conduct research. Although there are several schools of thought about the definition and scope of open science, many researchers agree that significant changes are taking place, at least regarding how research is conducted and results are shared. Many researchers also agree that open science has several advantages. There is a shared assumption that promoting open science will accelerate research, improve fairness, widen involvement, increase productivity, and promote innovation (McKiernan et al., 2016). More specifically, the advantages of practicing open science usually are discussed as falling into two categories: quality and sharing. Openness and sharing of data, analysis tools, methodologies, and reports produced during the research process lead to research transparency. The first line of discussion focuses on research transparency by opening up its processes, data, and materials and ultimately improving its quality (Baker, 2016; Nosek et al., 2015; Simmons et al., 2011). A series of studies have thus focused on research’s transparency and reproducibility. Ultimately, open science is linked to improved research quality.
A second line of discussion addresses the dissemination of research (McKiernan et al., 2016; Piwowar et al., 2018; Wilkinson et al., 2016). Sharing research results will allow more researchers to easily utilise data, tools, and methodologies to achieve increasingly diverse research outcomes. This openness and sharing can lead to innovation and even “citizen science”.
However, while many researchers agree about the merits of open science, there are various views on open science’s definition, as it is a complex concept. Open science is an emerging topic of study, and its researchers come from a variety of backgrounds. Nevertheless, as Vicente-Sáez and Martínez-Fuentes (2018) have pointed out, open science should be explored to better understand it, because it presents both opportunities and challenges in scholarly communities. In this regard, there have been various attempts to characterise open science in recent years, with methodologies including systematic reviews and qualitative analyses. The current study examined a dataset of open science articles in which the authors explicitly mention “open science” in the title or as a keyword, employing word co-occurrence analysis and network visualisation. This study’s results are expected to contribute to the literature attempting to describe open science.
Methodologically, using keyword bibliographic coupling, the current study attempted to overcome the limitations of word co-occurrence analysis in the context of sparse word distributions, such as author-generated keywords in articles. By applying both keyword co-occurrence analysis and bibliographic coupling analysis, the similarity of the two keywords is judged based on the number of shared references. Following the collection of author-provided keywords and references from open science articles, keyword bibliographic coupling is analysed and visualised in a network. Keyword bibliographic coupling has at least two advantages over traditional bibliographic coupling and keyword co-occurrence analysis. One is that keyword bibliographic coupling analysis can readily find similarities among a small number of keywords because it does not depend on the frequency of keyword co-occurrence. Second, this analysis is based on the similarity of the keyword unit containing the topic beyond the similarity analysis of the article unit. Through this analysis, we aim to understand how the domain of open science has been constructed over the past several decades. The study of open science is highly important in that it can assess open science’s present state and guide future research.
Related Studies
Open science has been discussed in various research communities, and its topics are noticeably diverse, including frameworks, challenges, how-to’s, research transparency, reproducibility, reliability, sharing, and dissemination. It is difficult to clearly define open science in addressing these diverse topics. Nevertheless, this study aims to divide open science research into two groups. The first group of research articles discusses the domain of open science, with systematic reviews serving as the primary methodology (Fecher & Friesike, (2014); Ogungbeni et al., (2018); Vicente-Sáez & Martínez-Fuentes, (2018)). The second group of articles investigate researchers’ perspectives on open science or analyse its actual practices (Cook et al., (2018); Levin et al., (2016); Maggin, (2021); Nosek et al., (2015); Whyte & Pryor, (2011)).
Several studies have attempted to improve our understanding of open science through systematic reviews. As Fecher and Friesike (2014) have indicated, there are five schools of thought on the meaning of open science: the public, democratic, pragmatic, infrastructure, and measurement. From the public perspective, science should be made available to the public in two ways: (1) making research processes publicly accessible and (2) making science understandable. The democratic school focuses on making science accessible. It is slightly different from the public perspective as it emphasises participation in research processes through publications, data, source materials, digital representations, or multimedia materials. For instance, open data and open access are characteristics of open science instruction according to the democratic perspective. The pragmatic school considers open science as disseminating research more efficiently. The infrastructure school considers open science as emerging through technological infrastructures such as software tools, applications, and networks. Lastly, the measurement school focuses on alternative measurement tools to assess scientific impact in open science environments.
Further, Vicente-Sáez and Martínez-Fuentes (2018) reviewed the field of open science and described it as a disruptive phenomenon. This is because open science creates sociocultural and technological change with respect to how research is designed, conducted, and evaluated. Their systematic review categorised the literature into five groups: open science as knowledge, transparent knowledge, accessible knowledge, shared knowledge, and collaboratively developed knowledge. Hence, they define open science as “transparent and accessible knowledge that is shared and developed through collaborative networks” (Vicente-Sáez & Martínez-Fuentes, (2018), p.435). In a different vein, Ogungbeni et al. (2018) explored the role of academic libraries in open science development by reviewing various research articles on the subject. Analysing 34 journal articles and four books, the researchers identified various definitions and schools of thought on open science and discussed academic libraries’ role therein. Metadata-related activities are important because open science fundamentally promotes collaboration and resource sharing.
There have also been attempts to understand open science by examining researchers’ perspectives and related practices. Levin et al. (2016) identified seven core themes of open science based on in-depth interviews with UK biomedical researchers: timely contribution of and access to research components, standards for the format and quality of research components, metadata & annotation, collaboration and cooperation with peers and communities, freedom to choose venues and strategies for disseminating research components, transparent peer review systems, and access to research components in non-Western and/or nonacademic contexts. Maggin (2021) investigated the perspectives of 140 journal editors and associate editors on open science in special education, school psychology, and related disciplines. The respondents considered research reproducibility a concern and viewed open science as improving research credibility through familiar practices. Nosek et al. (2015) presented eight standards and implementation steps to promote open science in journals, which include citation standards, data transparency, analytic method (code) transparency, research material transparency, design and analysis transparency, preregistration of studies, preregistration of the analysis plan, and replication. For instance, regarding data transparency standards, if the journal simply encourages or makes no mention of data sharing, it is rated at Level 0. After that, the levels are gradually elevated from 1 to 3. Level 1 articles state whether the data are available and provide a link to it if available. Articles are classified as Level 2 if the data are to be deposited into trusted repositories. Level 3 ensures that the data are deposited in trusted repositories and that the analysis reported in the article can be independently reproduced prior to publication. Nosek et al. (2015) consider practices such as data sharing, code sharing, research material sharing, results reproduction, preregistration, registered reports, and preprints to be characteristic of open science.
On the other hand, Whyte and Pryor (2011) interviewed 18 researchers in astronomy, bioinformatics, chemistry, epidemiology, language technology, and neuroimaging to identify a typology of the degree of openness. As the interviewees revealed, open science is not binary; rather, it is a continuous process. The degree of open science includes private management, collaborative sharing, peer exchange, transparent government, community sharing, and public sharing. Cook et al. (2018) have pointed out that conducting open science can improve transparency, openness, and reproducibility in special education and contribute to improving the trustworthiness of evidence. In this context, Cook et al. (2018) see open science as an emerging science reform that incorporates preprints, data and materials sharing, preregistration of studies and analysis plans, and registered reports.
As discussed above, Table 1 presents a list of studies concerning open science topics. All six studies discuss two topics: openness to the public and sharing. All cover the topic of transparency except Fecher and Friesike (2014). The topic of collaboration is identified as the domain of open science in Fecher and Friesike (2014), Vicente-Sáez and Martínez-Fuentes (2018), and Levin et al. (2016), but not in the remaining studies. The topic of standards and application appears in Fecher and Friesike (2014) and Levin et al. (2016). Other topics included are measurement in Fecher and Friesike (2014), knowledge in Vicente-Sáez and Martínez-Fuentes (2018), and private management in Whyte and Pryor (2011).
Topic | Fecher and Friesike (2014) | Vicente-Sáez and Martínez-Fuentes (2018) | Levin, Leonelli, Weckowska, Castle, and Dupré (2016) | Nosek et al. (2015) | Whyte and Pryor (2011) | Cook et al. (2018) |
---|---|---|---|---|---|---|
Openness to public | Public | Shared knowledge | - Access to research components in non-Western and/or nonacademic contexts | - Preprints | - Public sharing | - Preprints |
Sharing | Democratic | Accessible knowledge | - Timely contribution of and access to research components | - Data sharing - Code sharing - Research material sharing |
- Collaborative sharing - Peer exchange - Community sharing |
- Data and materials sharing |
Transparency | Transparent knowledge | -Transparent peer review systems | - Results reproduction - Preregistration - Registered reports |
- Transparent government | - Preregistration of studies and analysis plans - Registered reports | |
Collaboration | Pragmatic | Collaboratively developed knowledge | - Collaboration and cooperation with peers and communities | |||
Standards and application | Infrastructure | - Standards for format and quality of research components - Metadata and annotation |
||||
Other | Measurement | Knowledge | - Freedom to choose venues and strategies for disseminating research components | - Private management |
Research Methods
Data collection
Articles on open science were retrieved from the Web of Science on May 5, 2021. We performed a search with “open science” in the title or the author-generated keywords. The query was refined by document type as “ARTICLE *OR* EDITORIAL MATERIAL *OR* REVIEW *OR* EARLY ACCESS” with no time period limit. This query retrieved 1,007 articles in the field of open science.
We first processed the data in three steps and divided them into two datasets. In the first step, we reviewed the initial 1,007 articles, among which we found and deleted seven duplicate articles. Therefore, a total of 1,000 articles remained for the analysis. In the second step, we extracted author-generated keywords from the articles and constructed a keyword dataset. Because there are different ways to express the same word (e.g., “open access” and “OA,” “big data” and “big-data”), we cleaned the data according to the authority control rules. In the keyword dataset, 4,645 keywords appeared, of which 2,266 were unique. Table 2 shows the top 20 keywords according to their frequency of appearance. Except for “open science” used as a search term, the top keywords included “open access,” “reproducibility,” “open data,” “data sharing,” and “transparency.” “Open access” and “reproducibility” appeared most frequently (105 times and 95 times, respectively), appearing nearly twice as frequently as the subsequent keywords.
No. | Keyword | Freq. |
---|---|---|
1 | open science | 683 |
2 | open access | 105 |
3 | reproducibility | 95 |
4 | open data | 62 |
5 | data sharing | 55 |
6 | transparency | 51 |
7 | scholarly communication | 50 |
8 | replication | 43 |
9 | preregistration | 35 |
10 | scholarly publishing | 33 |
11 | data management | 29 |
12 | replicability | 22 |
13 | research methods | 20 |
14 | peer review | 19 |
15 | reproducible research | 18 |
16 | FAIR | 18 |
17 | COVID-19 | 17 |
18 | big data | 17 |
19 | citizen science | 17 |
20 | collaboration | 17 |
The third step extracted and constructed the reference dataset from entire articles for individual keywords. As there is diversity in the way reference data is expressed, the data cleaning process was performed according to the authority control rules. This process yielded the top 20 references presented in Table 3.
No. | Reference | Freq. |
---|---|---|
1 | Estimating the reproducibility of psychological science Aarts AA, 2015, SCIENCE, V349, DOI 10.1126/science.aac4716 |
137 |
2 | Promoting an open research culture Nosek BA, 2015, SCIENCE, V348, P1422, DOI 10.1126/science.aab2374 |
126 |
3 | False-positive psychology: Undisclosed flexibility in data collection and analysis allows presenting anything as significant Simmons JP, 2011, Psychological Science, V22, P1359, DOI 10.1177/0956797611417632 |
92 |
4 | The FAIR Guiding Principles for scientific data management and stewardship Wilkinson MD, 2016, SCI DATA, V3, DOI 10.1038/sdata.2016.18 |
85 |
5 | Why most published research findings Are false Ioannidis JPA, 2005, PLOS MED, V2, P696, DOI 10.1371/journal.pmed.0020124 |
75 |
6 | A manifesto for reproducible science Munafo MR, 2017, NAT HUM BEHAV, V1, DOI 10.1038/s41562-016-0021 |
75 |
7 | Measuring the prevalence of questionable research practices with incentives for truth telling John LK, 2012, PSYCHOL SCI, V23, P524, DOI 10.1177/0956797611430953 |
66 |
8 | Badges to acknowledge open practices: A simple, low-cost, effective method for increasing transparency Kidwell MC, 2016, PLOS BIOL, V14, DOI 10.1371/journal.pbio.1002456 |
62 |
9 | How open science helps researchers succeed McKiernan EC, 2016, ELIFE, V5, DOI 10.7554/eLife.16800 |
58 |
10 | The preregistration revolution Nosek BA, 2018, P NATL ACAD SCI USA, V115, P2600, DOI 10.1073/pnas.1708274114 |
53 |
11 | 1,500 scientists lift the lid on reproducibility Baker M, 2016, NATURE, V533, P452, DOI 10.1038/533452a |
52 |
12 | The sociology of science: Theoretical and empirical investigations Merton R.K., 1973. |
52 |
13 | HARKing: Hypothesizing after the results are known Kerr N L, 1998, Pers Soc Psychol Rev, V2, P196, DOI 10.1207/s15327957pspr0203_4 |
51 |
14 | Scientific utopia: II. Restructuring incentives and practices to promote truth over publishability Nosek BA, 2012, PERSPECT PSYCHOL SCI, V7, P615, DOI 10.1177/1745691612459058 |
49 |
15 | Open science: One term, five schools of thought Fecher B, 2014, OPENING SCI EVOLVING, P17, DOI 10.1007/978-3-319-00026-8_2 |
45 |
16 | Power failure: Why small sample size undermines the reliability of neuroscience Button KS, 2013, NAT REV NEUROSCI, V14, P365, DOI 10.1038/nrn3475 |
43 |
17 | Toward a new economics of science Partha D, 1994, RES POLICY, V23, P487, DOI 10.1016/0048-7333(94)01002-1 |
40 |
18 | The file drawer problem and tolerance for null results Rosenthal R, 1979, PSYCHOL BULL, V86, P638, DOI 10.1037/0033-2909.86.3.638 |
38 |
19 | Reinventing discovery: The new era of networked science Nielsen M, 2011. |
35 |
20 | Sharing detailed research data is associated with increased citation rate Piwowar HA, 2007, PLOS ONE, V2, DOI 10.1371/journal.pone.0000308 |
35 |
Data analysis
This study aimed to identify the intellectual structure of the open science domain by analysing research articles. While author, document, and keyword all could serve as units for examining such a structure, we analysed keywords; particularly, the relationship between keywords is based on the number of shared references, not simply the co-occurrence of keywords, as shown in Figure 1. For two keywords A and B, for example, they shared three references.
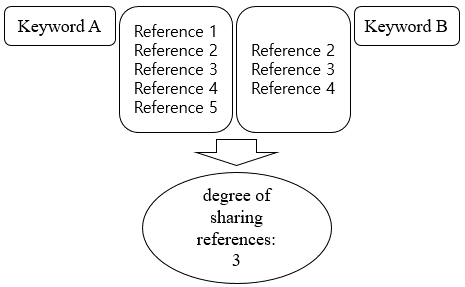
Figure 1: Conceptual diagram of keyword bibliographic coupling analysis (source: Lee and Chung 2022)
This analysis method is similar to the author bibliographic coupling analysis proposed by Lee (2008) and Zhao and Strotmann (2008), which identifies the relationship between authors based on the number of shared references. Hence, to construct the keyword bibliographic coupling network, two datasets—a keyword set and a reference set—were compiled from the 1,000 articles. As mentioned previously, there were 4,645 total keywords, of which 2,266 were unique. In the keyword dataset, 49 keywords with frequencies of 8 or greater were selected for analysis. To determine the sample size, we used the “square root of N plus one method” (Muralimanohar & Jaianand 2011). As the square root of 2,265 is 47.59 (~48), and the sum of the frequencies of the 49 keywords was 1,029, we contend that 49 keywords with frequencies of 8 or greater can sufficiently represent the entire keyword dataset.
On the other hand, 184 references with a frequency of 10 or greater were selected for analysis. The total frequency of all references was 44,871, of which 34,788 were unique. After selecting keywords and references, we constructed two matrices with 49 keywords and 184 references in terms of cosine similarity measures. Using these matrices, we visualised the intellectual structure of the open science domain in the pathfinder network (Schvaneveldt, 1990). Additionally, we identified clusters of keywords using the PNNC clustering algorithm (Kim and Lee, 2008; Lee, 2006). For data visualisation, we employed NodeXL.
Results
Overview
The number of articles on open science has increased, as shown in Figure 2. Because data collection took place in May 2021, articles published in 2021 (n = 95) are not displayed in Figure 2 because they do not represent the entire year. The first article on open science appearing in the Web of Science database was “Open science v. national security” (Ember, 1982). After this article’s publication in 1982, there was a formulating period in the field of open science and a sharp increase followed after the mid-2010s. Thus, research on open science can be considered a relatively new phenomenon that has progressed rapidly since the 2010s. This finding aligns with the results of the study by Zhang et al. (2018) showing that the field of open access of data has increased since 2010.
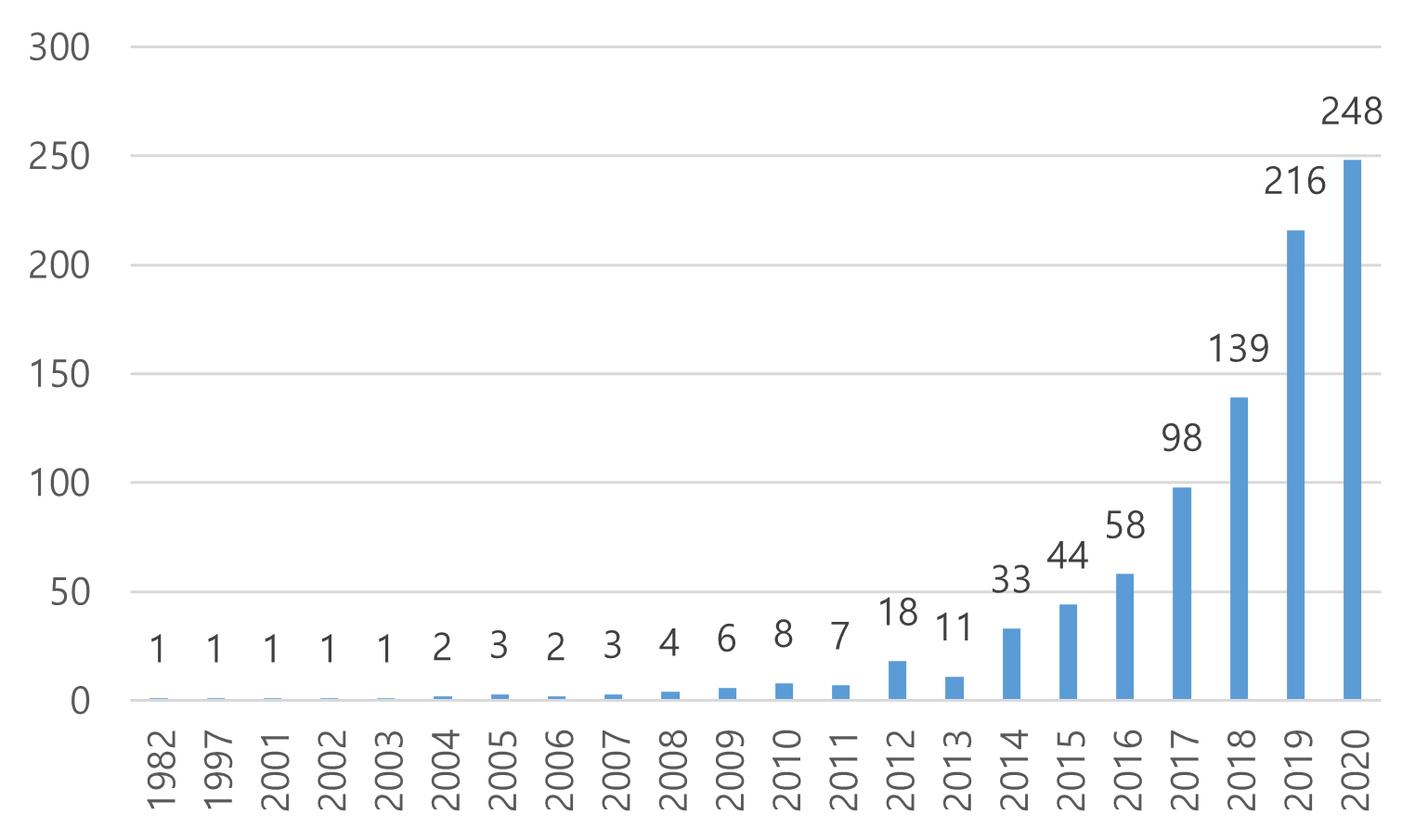
Figure 2: The trend of open science articles
A total of 4,392 unique authors was identified in this dataset. The average number of authors per article was approximately 4.4. The authors are from various disciplines, including medicine, computer science, biology, law, education, and informatics. Additionally, the analysis identified 622 journals in which open science articles were published. Similar to the distribution of authors, the distribution of journals in which open science research has been published is not skewed heavily toward a specific journal. The subject areas of published journal articles on open science were based on the Web of Science subject categories. As there can be multiple subject categories for a single journal, we identified 166 total subject categories. The subject area of Information Science & Library Science dominated with 138 entries. After that, topics such as Multidisciplinary Sciences, Computer Science, Information Systems, Psychology: Multidisciplinary, and Neurosciences appeared 78 times, 56 times, 54 times, and 51 times, respectively.
Primary open science keywords
We visualised the network in the open science field using the keyword-based bibliographic coupling technique (Figure 3). There was a backbone path of keywords including open innovation, science policy, open access, open data, reproducibility, transparency, replicability, preregistration, and replication. Among these keywords, open access, open data, reproducibility, and transparency were substantially recognised because the node size is represented in terms of frequency of appearance. The keyword open access was linked with related terms such as scholarly communication, collaboration, scholarly publishing, and open source. The keyword open data was connected with three others: data management, data sharing, and big data. The keyword reproducibility had six related keywords: COVID-19, research methods, ethics, journal policy, reproducible research, and policies. The keyword transparency was linked to social sciences, meta-research, and meta-science.
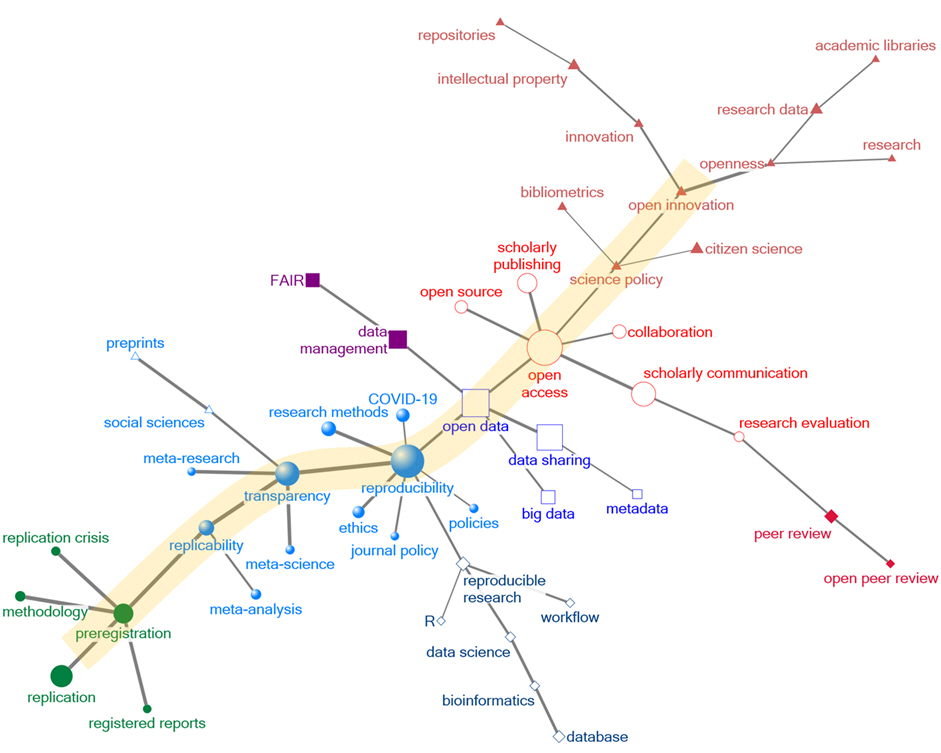
Figure 3: Backbone keywords in the open science network
Open science topics
Table 4 and Figure 4 show that nine topic clusters were revealed in this network using a clustering algorithm. They were C1-open access, C2-reproducibility, C3-data sharing, C4-preregistrations and registered reports, C5-research data, C6-open peer review, C7-tools and platforms for reproducible research, C8-open innovation and science policy, and C9-preprints. The cluster that played the most central role was C2-reproducibility, consisting of 11 keywords: reproducibility, transparency, replicability, COVID-19, ethics, journal policy, meta-analysis, meta-research, meta-science, policies, and research methods. Three primary references—Baker (2016), Nosek (2015), and Aarts (2015)—addressed reproducibility in the context of open science culture. Another major cluster was C1-open access, consisting of five keywords: open-source, scholarly publishing, collaboration, scholarly communication, and research evaluation. The three most common references in articles containing these keywords were Piwowar (2018), Lariviere (2015), and McKiernan (2016). These articles focus mainly on the current status of journal publications, the status of OA, and open science’s impact and advantages. The C3-data sharing cluster connected C2-reproducibility and C1-open access. On the other hand, although the C8-open innovation science policy cluster was located on the outskirts, it was large-scale, consisting of 11 keywords: science policy, bibliometrics, citizen science, open innovation, innovation, openness, intellectual property, repositories, research, research data, and academic libraries. Partha (1994), Fecher (2014), and Chesbrough (2003) were the most common references among articles containing these keywords. These articles mainly address new, innovative, and open science from an economic perspective and attempt to identify the domain of open science.
Clusters that were relatively small and located on the outskirts of this network included C5-research data, C6-open peer review, C9-preprints, C7-tools and platforms for reproducible research, and C4-preregistrations and registered reports. The C5-research data cluster was linked to C3-data sharing; it included the keywords data management and FAIR, and Wilkinson (2016) and Borgman (2012, 2015) were its most common references. These articles mainly address the guidelines and principles of data management. C6-open peer review was connected to C1-open access and included two keywords: peer review and open peer review. The most cited references were Ross-Hellauer (2017a), Ross-Hellauer (2017b), and Tennant (2017), which discuss the fundamental ideas and innovative approaches of peer review and open peer review. The C9-preprints cluster consisted of two keywords: preprints and social sciences. The most common references in this cluster were Bourne (2017), Piwowar (2018), and Ferguson (2012), which address preprints from an open access standpoint. The C7-tools and platforms for reproducible research cluster contained six keywords: reproducible research, R, workflow, data science, bioinformatics, and database. The cluster’s most common references were Peng (2011), Wilkinson (2016), and Sandve (2013), which address issues related to reproducible research in terms of guidelines and data. The C4-preregistrations and registered reports cluster was connected to the C2-reproducibility cluster and attached to the opposite end of the C8 cluster. This cluster contained five keywords: preregistration, replication crisis, methodology, replication, and registered reports. The most common references in the articles of this cluster discuss the credibility of published articles in terms of various methods.
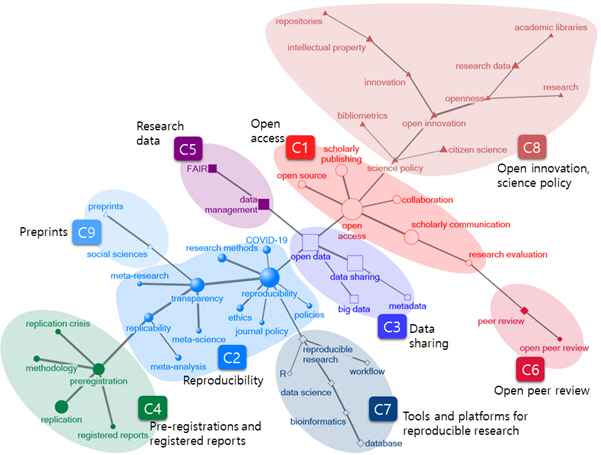
Figure 4: Nine topics in the open science network
Cluster | Topics | Keywords | Primary references |
---|---|---|---|
C1 | Open Access | open access, scholarly communication, collaboration, open source, research evaluation, scholarly publishing | Piwowar (2018), The state of OA: A large-scale analysis of the prevalence and impact of Open Access articles Lariviere (2015), The oligopoly of academic publishers in the digital era McKiernan (2016), How open science helps researchers succeed |
C2 | Reproducibility | reproducibility, transparency, replicability, COVID-19, ethics, journal policy, meta-analysis, meta-research, meta-science, policies, research methods | Baker (2016), 1,500 scientists lift the lid on reproducibility Nosek (2015), Promoting an open research culture Aarts (2015), Estimating the reproducibility of psychological science |
C3 | Data sharing | data sharing, open data, big data, metadata | Tenopir (2011), Data sharing by scientists: Practices and perceptions Wilkinson (2016), The FAIR Guiding Principles for scientific data management and stewardship Piwowar (2011), Who shares? Who doesn’t? Factors associated with openly archiving raw research data |
C4 | Preregistrations and registered reports | preregistration, methodology, registered reports, replication, replication crisis | Simmons (2011), False-positive psychology: Undisclosed flexibility in data collection and analysis allows presenting anything as significant Kerr (1998), HARKing: Hypothesizing after the results are known Nosek (2014), Registered reports: A method to increase the credibility of published result |
C5 | Research data | data management, FAIR | Wilkinson (2016), The FAIR Guiding Principles for scientific data management and stewardship Borgman (2012), The conundrum of sharing research data Borgman (2015), Big data, little data, no data: Scholarship in the networked world |
C6 | Open peer review | open peer review, peer review | Ross-Hellauer (2017a), What is open peer review? A systematic review Ross-Hellauer (2017b), Survey on open peer review: Attitudes and experience amongst editors, authors, and reviewers Tennant (2017), A multidisciplinary perspective on emergent and future innovations in peer review |
C7 | Tools and platforms for reproducible research | bioinformatics, reproducible research, data science, database, R, workflow | Peng (2011), Reproducible research in computational science Wilkinson (2016), The FAIR Guiding Principles for scientific data management and stewardship Sandve (2013), Ten simple rules for reproducible computational research |
C8 | Open innovation, science policy | open innovation, openness, science policy, innovation, intellectual property, research data, academic libraries, bibliometrics, citizen science, repositories, research | Partha (1994), Toward a new economics of science Fecher (2014), Open science: One term, five schools of thought Chesbrough (2003), Open innovation: The new imperative for creating and profiting from technology |
C9 | Preprints | preprints, social sciences | Bourne (2017), Ten simple rules to consider regarding preprint submission Piwowar (2018), The state of OA: A large-scale analysis of the prevalence and impact of Open Access articles Ferguson (2012), A vast graveyard of undead theories: Publication bias and psychological science’s aversion to the null |
Discussion
This study intended to identify the intellectual structure of the open science domain by analysing a total of 1,000 articles and 4,645 keywords using a keyword-based bibliographic coupling network. The results revealed nine subject clusters in the open science domain: C1-open access, C2-reproducibility, C3-data sharing, C4-preregistrations, and registered reports, C5-research data, C6-open peer review, C7-tools and platforms for reproducible research, C8-open innovation and science policy, and C9-preprints.
The nine clusters derived in this study are comparable to the six topics identified in previous studies. In such articles, the topic of openness to the public has been discussed in terms of public knowledge sharing—more specifically, providing access to research constituents in broader contexts such as non-Western and/or nonacademic audiences (Levin et al., 2016). Additionally, preprints have mainly been discussed in relation to sharing knowledge with the public. The current study’s results indicate that the clusters of open access, open innovation and science policy, and preprints (C1, C8, and C9) encompass the topic of openness to the public. This study shows that open access can be understood in the context of changes in the scholarly environment, such as scholarly publishing, research evaluation, and collaboration.
Moreover, as evidenced by the open innovation and science policy cluster, the literature is discussing citizen science in the context of open innovation and science policy. Further, the preprints cluster comprises discussions in the field of social sciences. The topic of sharing has been addressed from the perspective of democratic sharing of data, code, and materials with fellow researchers in related studies. On the other hand, the present study revealed a focus on data— including sharing data, metadata for data sharing, and principles and guidelines (e.g., FAIR) of data management—as evidenced by the C3-data sharing and C5-research data clusters. Transparency also emerged as a primary discussion topic, contributing to four clusters: reproducibility (C2), preregistration and registered reports (C4), open peer review (C6), and tools and platforms for reproducible research (C7). In the relevant studies, transparency encompasses results reproduction, preregistration and registered reports, and transparent peer review systems. As shown by the reproducibility cluster, topics such as research policy, ethics, methods (such as meta-analysis or meta-science) and journal policy are frequently being discussed in the literature. The preregistration and registered reports cluster also demonstrates that preregistration, registered reports and replication are being studied as methodological tools to ameliorate the replication crisis. Additionally, the open peer review cluster demonstrates the prominence of transparent peer review systems as a discussion topic in the literature. Related studies discuss standards and applications in terms of infrastructure, format standards and the quality of research components, and metadata and annotation.
On the other hand, this study shows that tools and platforms focus more on research reproducibility. Notably, the C7-tools and platforms for reproducible research cluster encompassed discussions of tools and platforms to enhance transparency, which are particularly active in bioinformatics. These discussions specifically concern databases, R, workflow, and data science. The topic of collaboration put forth by related studies did not emerge as an individual cluster in this study. Another noteworthy cluster is C8-open innovation and science policy, which previous studies have not addressed in-depth. This cluster concerns innovations in open science and includes subject areas such as science policy, intellectual property, research data, academic libraries, repositories, citizen science and bibliometrics.
Comparing this study with related studies identifying the domain of open science, two key insights can be derived. First, research transparency and reproducibility form large clusters with detailed keywords. Moreover, it is noteworthy that a separate cluster for tools and platforms for research reproducibility emerged. These results show that recent studies on open science have been actively discussing research transparency and reproducibility. Second, a cluster concerning open innovation and science policy emerged in this study, but this again has not been presented clearly in previous studies. This is an important finding in that this is a crucial area addressing the innovative methods and policies necessary for improving open science.
However, this study is not free from methodological limitations in the word co-occurrence analysis. Representing articles’ topics using author-generated keywords could be limited, such as with expressing similar concepts differently and excluding conceptual hierarchical relationships. Nevertheless, this technique addresses limitations of the word co-occurrence analysis technique because it analyses the co-occurrence of words and references simultaneously. More specifically, as suggested by Lee and Chung (2022), by using keyword bibliographic coupling analysis, we could identify a detailed intellectual structure. The keyword co-occurrence technique tends to rely on sparse data because the number of author-provided keywords is typically less than five. Addressing this limitation, the keyword bibliographic coupling analysis establishes a relationship based on the number of references shared by the keywords, not the keywords’ co-occurrence. In this regard, it measures the relationship between the two keywords more comprehensively compared to the keyword co-occurrence analysis. Furthermore, because the intellectual structure of a specific domain is identified as subfields or clusters, and key references can be extracted along with them, the interpretation of the subfields can be improved based on the key references within each field.
Conclusion
This study aimed to describe the domain of open science. In our dataset, the first article on open science was published in 1982, and since the mid-2010s, there has been a rapid increase in the number of such articles. Additionally, we observed that authors from various disciplines, such as public health, medicine, education, and computer science/information science, have published articles on open science. Articles in journals publishing heavily in open science tend to focus on changes in the academic environment, address research policy and come from interdisciplinary fields. We categorised the journals by subject areas, such as information science and library science, multidisciplinary science, computer science, and so on. By utilising the keyword bibliographic coupling analysis network, we identified the prominent keywords of “open access,” “open data,” “reproducibility,” “transparency,” “replicability,” “preregistration,” “replication,” “science policy,” and “open innovation.” While keywords such as “open access,” “open data,” and “reproducibility” are discussed frequently, keywords such as “transparency,” “replicability,” “preregistration,” “replication,” “science policy,” and “open innovation” are relatively less salient. Moreover, we identified a total of nine clusters—C1-open access, C2-reproducibility, C3-data sharing, C4-preregistrations, and registered reports, C5-research data, C6-open peer review, C7-tools and platforms for reproducible research, C8-open innovation and science policy, and C9-preprints—which we compared to the six topics identified in previous studies. The present findings help clarify the current state of open science. It is expected that the development and promotion of desirable research areas will be possible based on this new understanding of open science as a phenomenon. Given that open science has rapidly expanded since the mid-2010s, it would be beneficial to examine these temporal changes in a follow-up study.
About the authors
Dr. Jae Yun Lee is a Professor in the Department of Library and Information Science at Myongji University, Seoul, South Korea. He received his Bachelor’s, Master’s, and Doctoral degrees all in Library and Information Science from Yonsei University. Before joining Myongji University, he served as an assistant and associate professor at Kyonggi University until February 2013. His research interests include network analysis, informetrics, data literacy, and scholarly communication. He can be contacted at memexlee@mju.ac.kr
Dr. EunKyung Chung (Corresponding Author) is a Professor in the Department of Library and Information Science at the Ewha Womans University, Seoul, South Korea. She received her Bachelor’s degree in Library and Information Science from Ewha Womans University, her Master’s degree in Computer Science, and her Ph.D. in Information Science from the University of North Texas. Her research interests include visual information retrieval and network analysis. She can be contacted at echung@ewha.ac.kr
References
- Aarts, A.A., Anderson, J.E., Anderson, C.J., Attridge, P.R., Attwood, A., Axt, J., Babel, M., Bahník, Š., Baranski, E., Barnett-Cowan, M., Bartmess, E., Beer, J., Bell, R., Bentley, H., Beyan, L., Binion, G., Borsboom, D., Bosch, A., Bosco, F.A., ... & Zuni, K. (2015). Estimating the reproducibility of psychological science. Science, 349(6251) https://doi.org/10.1126/science.aac4716
- Baker, M. (2016). 1,500 scientists lift the lid on reproducibility. Nature, 533(7604), 452-454. https://doi.org/10.1038/533452a
- Borgman, C.L. (2012). The conundrum of sharing research data. Journal of the American Society for Information Science and Technology, 63(6), 1059-1078. https://doi.org/10.1002/asi.22634
- Borgman, C.L. (2015). Big data, little data, no data: Scholarship in the networked world. MIT press.
- Bourne, P.E., Polka, J.K., Vale, R.D., & Kiley, R. (2017). Ten simple rules to consider regarding preprint submission. PLOS Computational Biology, 13(5), e1005473. https://doi.org/10.1371/journal.pcbi.1005473
- Chesbrough, H.W. (2003). Open innovation: The new imperative for creating and profiting from technology. Harvard Business Press.
- Cook, B.G., Lloyd, J.W., Mellor, D., Nosek, B.A., & Therrien, W. J. (2018). Promoting open science to increase the trustworthiness of evidence in special education. Exceptional Children, 85(1), 104-118. https://doi.org/10.1177/0014402918793138
- Ember, L.R. (1982). Open Science V. National-Security. Chemistry & Industry. 15, 506.
- Fecher, B., & Friesike, S. (2014). Open science: one term, five schools of thought. In. S. Bartling, and S. Friesike (Eds.), Opening science: The Evolving Guide on How the Internet is Changing Research, Collaboration and Scholarly Publishing (pp. 17-47). Springer. https://doi.org/10.1007/978-3-319-00026-8_2
- Ferguson, C.J., & Heene, M. (2012). A vast graveyard of undead theories: Publication bias and psychological science’s aversion to the null. Perspectives on Psychological Science, 7(6), 555-561. https://doi.org/10.1177/1745691612459059
- Kerr, N.L. (1998). HARKing: Hypothesizing after the results are known. Personality and social psychology review, 2(3), 196-217. https://doi.org/10.1207/s15327957pspr0203_4
- Kim, H., & Lee, J.Y. (2008). Exploring the emerging intellectual structure of archival studies using text mining: 2001-2004. Journal of Information Science, 34(3), 356-369. https://doi.org/10.1177/0165551507086260
- Larivière, V., Haustein, S., & Mongeon, P. (2015). The oligopoly of academic publishers in the digital era. PloS one, 10(6), e0127502. https://doi.org/10.1371/journal.pone.0127502
- Lee, J.Y. (2006). A novel clustering method for examining and analyzing the intellectual structure of a scholarly field. Journal of the Korean Society for Information Management, 23(4), 215-231. http://doi.org/10.3743/KOSIM.2006.23.4.215
- Lee, J.Y. (2008). Bibliographic author coupling analysis: A new methodological approach for identifying research trends. Journal of the Korean Society for Information Management, 25(1), 173-190. https://doi.org/10.3743/KOSIM.2008.25.1.173
- Lee, J.Y., & Chung, E. (2022). Introducing keyword bibliographic coupling analysis(KBCA) for identifying the intellectual structure. Journal of the Korean Society for Information Management, 39(1), 309-330. https://doi.org/10.3743/KOSIM.2022.39.1.309
- Levin, N., Leonelli, S., Weckowska, D., Castle, D., & Dupré, J. (2016). How do scientists define openness? Exploring the relationship between open science policies and research practice. Bulletin of Science, Technology & Society, 36(2). 128-141. https://doi.org/10.1177/0270467616668760
- Maggin, D.M. (2021). Journal Editor and Associate Editor Perspectives on Research Reproducibility and Open Science. Remedial and Special Education, 43(3), 135-146. https://doi.org/10.1177/07419325211017294
- McKiernan, E.C., Bourne, P. E., Brown, C. T., Buck, S., Kenall, A., Lin, J., McDougall, D., Nosek, B.A., Ram, K., Soderberg, C.K., Spies, J.R., Thaney, K. Updegrove, A., Woo, K.H., & Yarkoni, T. (2016). Point of view: How open science helps researchers succeed. elife, 5, e16800. https://doi.org/10.7554/eLife.16800
- Mirowski, P. (2018). The future(s) of open science. Social Studies of Science, 48(2), 171-203. https://doi.org/10.1177/0306312718772086
- Muralimanohar, J., & Jaianand, K. (2011). Determination of Effectiveness of the ‘Square Root of N Plus One’ Rule in Lot Acceptance Sampling Using an Operating Characteristic Curve. The Quality Assurance Journal, 14(1-2), 33-37. https://doi.org/10.1002/qaj.482
- Nosek, B.A., & Lakens, D. (2014). Registered reports: A method to increase the credibility of published results. Social Psychology, 45(3), 137-141. https://doi.org/10.1027/1864-9335/a000192
- Nosek, B.A., Alter, G., Banks, G. C., Borsboom, D., Bowman, S. D., Breckler, S. J., Buck, S., Chambers, C.D., Chin. G., Christensen, G., Contestabilea, M., Dafoe, A. Eich, E., Freeser, J. Glennersterd, R., Goroff, D., Green, D.P., Hesse, B., Humphreys, M.,... & Yarkoni, T. (2015). Promoting an open research culture. Science, 348(6242), 1422-1425. https://doi.org/10.1126/science.aab2374
- Ogungbeni, J.I., Obiamalu, A.R., Ssemambo, S., & Bazibu, C.M. (2018). The roles of academic libraries in propagating open science: A qualitative literature review. Information Development, 34(2), 113-121. https://doi.org/10.1177/0266666916678444
- Partha, D., & David, P.A. (1994). Toward a new economics of science. Research policy, 23(5), 487-521. https://doi.org/10.1016/0048-7333(94)01002-1
- Peng, R.D. (2011). Reproducible research in computational science. Science, 334(6060), 1226-1227. https://doi.org/10.1126/science.1213847
- Piwowar, H.A. (2011). Who shares? Who doesn't? Factors associated with openly archiving raw research data. PloS one, 6(7), e18657. https://doi.org/10.1371/journal.pone.0018657
- Piwowar, H., Priem, J., Larivière, V., Alperin, J. P., Matthias, L., Norlander, B., Farley, A., West, J., & Haustein, S. (2018). The state of OA: a large-scale analysis of the prevalence and impact of Open Access articles. PeerJ, 6, e4375. https://doi.org/10.7717/peerj.4375
- Ross-Hellauer, T. (2017a). What is open peer review? A systematic review. F1000Research, 6. https://doi.org/10.12688/f1000research.11369.2
- Ross-Hellauer, T., Deppe, A., & Schmidt, B. (2017b). Survey on open peer review: Attitudes and experience amongst editors, authors and reviewers. PloS one, 12(12), e0189311. https://doi.org/10.1371/journal.pone.0189311
- Sandve, G.K., Nekrutenko, A., Taylor, J., & Hovig, E. (2013). Ten simple rules for reproducible computational research. PLoS computational biology, 9(10), e1003285. https://doi.org/10.1371/journal.pcbi.1003285
- Schvaneveldt, R.W. (1990). Pathfinder associative networks: Studies in knowledge organizations. Ablex Publishing.
- Simmons, J.P., Nelson, L.D., & Simonsohn, U. (2011). False-Positive psychology: Undisclosed flexibility in data collection and analysis allows presenting anything as significant. Psychological Science, 22(11), 1359-1366. https://doi.org/10.1177/0956797611417632
- Tennant, J.P., Dugan, J.M., Graziotin, D., Jacques, D.C., Waldner, F., Mietchen, D., Elkhatib, Y., Collister, L.B., Pikas, C.K., Crick, T., Masuzzo, P., Caravaggi, A., Berg, D.R., Niemeyer, K.E., Ross-Hellauer, T., Mannheimer, S., Rigling, L., Katz, D.S., Tzovaras, B.G.... & Colomb, J. (2017). A multi-disciplinary perspective on emergent and future innovations in peer review. F1000Research, 6. https://doi.org/10.12688/f1000research.12037.2
- Tenopir, C., Allard, S., Douglass, K., Aydinoglu, A.U., Wu, L., Read, E., Manoff, M., & Frame, M. (2011). Data sharing by scientists: practices and perceptions. PloS one, 6(6), e21101. https://doi.org/10.1371/journal.pone.0021101
- Vicente-Sáez, R., & Martínez-Fuentes, C. (2018). Open Science now: A systematic literature review for an integrated definition. Journal of Business Research, 88, 428-436. https://doi.org/10.1016/j.jbusres.2017.12.043
- Whyte, A., & Pryor, G. (2011). Open science in practice: Researcher perspectives and participation. The International Journal of Digital Curation, 1(6), 199-213. https://doi.org/10.2218/ijdc.v6i1.182
- Wilkinson, M., Dumontier, M., Aalbersberg, I., Appleton, G., Axton, M., Baak, A., Blomberg, N., Boiten, J., Bonino da Silva Santos, L.O., Bourne, P.E., Bouwman, J., Brookes, A.J., Clark, T., Crosas, M., Dillo, I., Dumon, O., Edmunds, S., Evelo, T.E., Finkers, R., ... & Mons, B. (2016). The FAIR Guiding Principles for scientific data management and stewardship. Scientific Data, 3, 160018. https://doi.org/10.1038/sdata.2016.18
- Woelfle, M., Olliaro, P., & Todd, M. H. (2011). Open science is a research accelerator. Nature chemistry, 3(10), 745-748. https://doi.org/10.1038/nchem.1149
- Zhang, Y., Hua, W., & Yuan, S. (2018). Mapping the scientific research on open data: A bibliometric review. Learned Publishing, 31(2), 95-106. https://doi.org/10.1002/leap.1110
- Zhao, D., & Strotmann, A. (2008). Evolution of research activities and intellectual influences in information science 1996–2005: Introducing author bibliographic‐coupling analysis. Journal of the American Society for Information Science and Technology, 59(13), 2070-2086. https://doi.org/10.1002/asi.20910