Social network analysis of the mental health sub-topic on the MedlinePlus subject directory
Yifan Zhu, and Jin Zhang.
Introduction. A subject directory plays an important role in a Web portal and it helps users effectively navigate the portal. This study examines a subject directory system related to Mental Health in the MedlinePlus portal and provides suggestions of optimisation to enhance the subject directory system.
Method. A mixed research method combining social network analysis and inferential statistics was applied.
Analysis. A structural and a semantic social network were built regarding the selected health topics related to mental health in the MedlinePlus portal. The two networks were compared and the outcomes were evaluated by domain experts.
Results. Among the ninety-nine collected health topics related to mental health, three themes were identified through the visualisation analysis regarding grouped health topics. Patterns and characteristics of each theme group were discussed. As a result, fifty-five bidirectional and twenty-three unidirectional edges were identified and recommended to be added to the corresponding health topic pages. The recommended results indicate that the subject directory of specific mental health related topics is well constructed, while health consumer groups related topics might need more improvements. The optimised subject directory has significantly stronger semantic connection, and the results of the recommendations are consistent with the evaluation outcome of two domain experts.
Conclusions. The findings of this study can provide ideas of optimising and enhancing the subject directory system to the public health portal creators and health professionals, and benefit health consumers for seeking health information online. The methodologies applied in this study may provide a novel way to investigate and enhance subject directories in general.
DOI: https://doi.org/10.47989/irpaper876
Introduction
A Web portal refers to a site 'that provides a number of services useful to a particular interest group or purpose' (Schleyer and Spallek, 2002). Such services might vary due to different sources or targeted user groups while many common features such as content aggregation, customisation, and portal administration and maintenance functions, have been shared among the majority of Web portals (Wege, 2002). Meanwhile, some researchers believe that a Web portal with unique structural features can significantly impact user behaviour such as information searching and decision making (Baird et al., 2012). In this study, only those public portals focusing on providing services and information useful to a community were discussed.
The trend of searching health information online has long been indicated by the rapidly growing amount of online public health portals since the beginning of the 21st century (Evers, 2006). Among those comprehensive sites that are regarded as online health portals is MedlinePlus. Launched in 1998, it was the first primary initiative for providing online health information to the public from the National Library of Medicine (Miller et al., 2000).
Today, when people become aware of a health problem, the Internet is a common starting point to seek information. The subject directory of MedlinePlus offers Internet searchers a browsing environment where they could start from a broad term and refine their search terms to meet their real information needs, thus resulting in a better information search. In fact, the Medical Library Association lists MedlinePlus as one of the nine 'top health Websites' for offering general health information (World Health Organization, n.d.). Mental health is of particular concern to the general public due to the pandemic caused by Covid-19. For those novice users who are not familiar with relevant domain knowledge, the MedlinePlus's directory can be of great assistance and lead the portal to adapt to a more general population. Therefore, it is necessary to investigate if the portal is providing a consistently organised format of health information to its users.
A Web portal like MedlinePlus contains dynamic, rich, and comprehensive information. The portal usually covers a variety of information. To better serve its users, the information has to be organised effectively and provide its users with search mechanisms. An internal search engine and a subject directory are common means for this purpose. Unlike search engines which are normally maintained by Web crawlers and robots, subject directories commonly depend on human editors. Information included in subject directories gets reviewed and annotated in most cases (Johnston, 2006).
Query search allows users to find relevant information to a specific information need while browsing enables users to discover and explore relevant information to a non-specific information need. Subject directories are important because users often begin their search in a too general way (Taylor, 1967) due to the lack of prior knowledge toward their search tasks (Pang et al., 2016), hence a subject directory may suggest narrower terms that can better fit the information needs of the users. This process is normally regarded as a process of refinement, and indicates that users find real information need by association; they start from a wrong term, then go through browsing directories, and finally identify relevant information (Bates, 1989; Demelo et al., 2017).
A subject directory is a powerful tool to help users navigate the sophisticated Web portal and locate useful information to meet their information needs. Due to its hierarchy, a subject directory supports users to discover relevant information from a broad topic to a narrow topic, from a specific topic to a general topic, and from a topic of interest to more related topics. As a result, these unique characteristics make the subject directory a vital and useful tool in a Web portal.
A subject directory system and its involved health topics in the MedlinePlus portal formed a network where a specific research methodology, social network analysis, is applicable. Social network analysis method examines, measures, and evaluates the characteristics of a network and connections among its actors (or nodes) on a network. Social network analysis focuses on the interactions between the actors. The uniqueness of the social network analysis method is that it can quantify the connections of a node to other nodes on a network from multiple perspectives. In addition, it measures not only the relationships between the node and its directly linked nodes but also the relationships between the node and indirectly linked nodes on the network. This study applied social network analysis to explore the health topic directories and connection patterns among the health topics that comprised the subject directory of the MedlinePlus portal, and identified the influential topics (i.e., those health topics which play more important roles than others in connecting different topics) among the topic network.
Investigating mental health related information in the MedlinePlus portal is necessary for related health consumer groups. Among hundreds of health conditions and diseases, mental disorders are generally characterized by the World Health Organisation as 'some combination of abnormal thoughts, emotions, behaviour and relationships with others' (World Health Organization, 2018). According to the fact sheet published by WHO in 2018, the burden of mental disorders keeps growing 'with significant impacts on health and major social, human rights and economic consequences in all countries of the world'. Moreover, the fact sheet also pointed out that common mental diseases such as depression (264 million), bipolar affective disorder (45 million), and schizophrenia (20 million) have had a wide range of patients globally (World Health Organization, 2018).
The objectives of this study included investigating the Mental Disorder topic and its related topics on MedlinePlus's subject directory through social network analysis and inferential statistical methods, evaluating the relationships among these health topics, and providing constructional recommendations for further improvement. The topic Mental Disorders was selected for two reasons: 1) it is the official term used by the MedlinePlus portal to represent mental related diseases - other related terms such as Mental Illness have been combined into the same topic page; 2) compared with the other candidate topic Mental Health whose page only contains three related health topics (Child Mental Health, Mental Disorders, and Teen Mental Health), the topic page of Mental Disorders includes a wider range of related health topics (nineteen in total). Those topics have covered not only the same topics of Mental Health, but also a group of additional common mental health related topics such as Anxiety and Bipolar Disorder.
Related research
Seeking public health information online and browsing
For patients that are curious about both present and the long-term effect of their or their family's health conditions, information seeking is a “critical mechanism” for exploring answers towards questions raised by or related to the illness (Johnson and Case, 2012). Among various health consumer groups, some were reported to have a high percentage of population seeking health information online, such as patients with irritable bowel syndrome (Halpert et al., 2007) and patients eligible for bariatric surgery (weight loss surgery) (Paolino et al., 2015). In addition, among the general public, online information seeking was claimed to be distinguished by specific demographic characteristics (including sex, health condition level, educational background) and Internet-related factors (such as Web skills) despite the existence of exceptions and inconsistent conclusions in some studies (Rains, 2018).
Generally, health information sought by health consumers online could be classified into two topics: technical information regarding a health condition from a medical perspective; and experiential information that can provide personal experience as well as emotional support among people who are dealing with a specific illness (Fergie et al., 2016). Compared with experiential information, technical information appears more frequently in health portals that are operated by authoritative organisations like the National Institute of Health in the United States since they cover information related to specific conditions and treatments (Rains, 2018). With the integration of Web 2.0, the connection between online Web portals and users has been strengthened (Postigo, 2011). As a result, these portals have become the front door to access to the information needed by online health consumers (Zhang et al., 2016).
For information searching purposes, there are mainly two means: the browsing applied by a subject directory, and the query search applied by a search engine (Chung and Noh, 2003; Zhang et al., 2016; Zhang, Zhai, Stevenson et al., 2016). Browsing search had raised much attention from researchers since the occurrence of Web-based information seeking and a lot of models were developed in previous literature such as Choo et al. (2000). Studies from researchers like Zhang et al. (2016, 2016), and Chung and Noh (2003), preferred subject directories because they provide an intuitive way of leading users from general to specific information, and the terms in subject directories have been normalised and controlled. However, when it comes to the health field, previous studies also raised concerns such as inconsistent data formats (Yeo et al., 2010).
Besides the discussion regarding the two means of information searching from the theoretical perspective, other researchers also investigated how health consumers seek information online in real life. Some earlier studies regarded browsing search as a different way of getting informed in an increasingly fragmental digital environment and argued that browsing search may provide a deeper engagement to information seekers compared with search engines and social media platforms (Möller et al., 2019). Authors such as Pang et al. (2016) reported that users' health information seeking behaviour might be affected by the various nature of search tasks, and browsing search was found to be connected to serendipity as well as curiosity since browsing search could often bring health information that seekers do not know much about or have not thought of before the search task. This conclusion was further related to the fact that seekers might apply browsing search when they have little knowledge about a specific health topic. Hence, such searching could provide hints for further search directions.
This finding was echoed in a later study conducted by Demelo et al. (2017) where they had identified the lack of domain-specific knowledge and vocabulary as a major barrier for health information seeking. As a result, Demelo et al. (2017) developed an ontology-driven search interface and found that the interface was helpful for addressing knowledge/vocabulary difficulty.
Moreover, evidence was observed in Pang et al.'s (2016) paper in which they obtained the navigation data of visitors to one of the largest public health portal in Australia. Their findings revealed that 'a number of users continue to look for additional information after the first read, by navigating to other pages, browsing the home page, and using the search functions'. To be more specific, significant navigation flows were investigated among categories like conditions and treatments and A-Z health content, which implied the importance of subject directories in assisting health consumers for seeking information. Similar portal transitions of browsing search were also observed in an Eastern European public library's Website (Shevchenko, 2020). The researchers noted that the improvements brought by setting up related topic pages from certain patent subjects had led the average amount of portal page transitions to a 40 per cent level. In addition to public health and library domains, subject directories were also widely utilised in other domains. For instance, a taxonomy containing twelve categories was developed for wayfinding behaviour in the UK (Barker, 2019). However, these studies mostly focused on general health information seeking, few studies were found to shed light on the mental health area.
Previous studies also generated new ideas for improving subject directories, for instance, a text mining technology based on Self-Organizing Map (SOM) and a following Navigational Self-Organizing Map (NaviSOM) in assisting with a navigational structure for online information (Yang and Lee, 2004, 2006). Other studies analysed the transaction logs and made suggestions for optimising obesity and weight control subject directories (Zhang et al., 2008; Zhang and An, 2009).
Mental health diseases and health consumers on the Internet
Earlier studies have revealed the fact that health consumers with poorer health conditions tended to rely on the Internet when collecting information related to both physical and mental health issues (Fox and Rainie, 2000). Following that thought, the standards for sites containing physical and mental health related content were proposed by researchers (Morahan-Martin, 2004). Some scholars compared common mental health disorders (CMHDs) with diabetes and claimed that health consumers suffering from these two types of health conditions shared several similarities such as the requirement of self-management (Sterling et al., 2010), hence they both considered seeking information online as a crucial element (Fergie et al., 2016).
Among various health consumer groups, adolescents and young adults are unique when discussing mental health because almost 50% of lifetime prevalence of DSM-IV (Diagnostic and Statistical Manual of Mental Disorders, 4th Edition) disorders appear by age 14, with three quarters of symptoms occurring by age 24 (Kessler et al., 2005). Although 30.8 per cent of young college students aged between 18 and 24 were found to use the Internet for mental health information and support, questions remained on how specifically they utilised the Internet (Horgan and Sweeney, 2010).
Echoing that, for young people aged 12 to 25 in Australia, the Internet was used by the majority to connect with other young people on mental health issues (Burns et al., 2010). This phenomenon was echoed by other studies, and young adults were found to have contributed a great amount of mental health related content to various Internet platforms, thus receiving great opportunities for intervention from health professionals (Yonker et al., 2015). Meanwhile, nearly half of the young adults in Australia were reported to search for information about specific mental health problems even if they were not having any. The authors also reported that such information seeking activities varied according to age and sex.
Besides children and teenagers, another important health consumer group that has raised great attention from researchers is the group of older adults. Compared with children and teenagers, older adults were found to encounter more barriers in seeking mental health related information online. According to Conner et al. (2010), 'Stigma associated with having a mental illness has a negative influence on attitudes and intentions toward seeking mental health services among older adults with depression, particularly African American elders'.
Moreover, from the aspect of constructing subject directories regarding online health information, various health conditions were found to be linked to specific health consumer groups. For instance, according to WHO and MedlinePlus portal, several health issues such as diabetes, development issues, and school health are shared among children and teenagers. However, when looking closer at the structure of MedlinePlus portal's subject directory, it is clear that teenagers are faced with more mental illness related conditions since they are more connected to underage drinking, underage driving, sexual health, pregnancy, drug abuse, and depression. On the other hand, according to the constructiond of MedlinePlus's subject directories, older adults are found to be linked to health conditions such as elder abuse, Alzheimer's Disease, stroke, heart problems. These facts indicate some relationships exist among specific health topics in the subject directory systems of many online public health portals.
Social network analysis
The original history of social network analysis could be traced back to the 1930s, but social network analysis was first theorised as a new concept by Barnes (1954), with its roots lying in theories of social action (Coleman, 1986). As a result, it had become an established field within social science by the 1980s (Borgatti. et al., 2009).
According to Otte and Rousseau (2002), social network analysis has been employed to explore many fields within the information science area. Instances include subject classification, citation network and bibliometrics. Previous research in health informatics included information exchanges among health consumers (Mertens et al., 2012), the impact left by culture on health consumers' health information searching (Smith and Christakis, 2008), the feedback of usage from adolescents towards health information (Gray et al., 2005), and the interaction among nurses, patients, and other actors (Pow et al., 2012). Moreover, social network analysis has been proven to work effectively with other research methods such as content analysis methods in the healthcare field, for instance, the breast cancer related health information on Twitter (Kim et al., 2016).
On the other hand, some researchers have brought an interesting idea of employing social network analysis into evaluation of the topic/subject based navigation systems administrated by public health portals like World Health Organisation (Zhang et al., 2016) and the U.S.'s government agriculture portal (Zhang et al., 2016). Their findings suggested that some subjects connected with hyperlinks might not have significant semantic relationships while some other topics sharing a significant number of keywords do not reflect in the structural link relationship.
Summary
The previous literature has shed more light on social network sites and search-based navigation means. Previous studies focused more on health consumers actively seeking specific health information or emotional support while the importance, benefits, and potential development of organised health information for general browsing purposes provided by health professional institutions requires further research.
In addition, mental health related subject directory systems have seldom been noticed during the previous research work. Hence, investigating the section of mental health on a representative health Web portal's subject directory might provide opportunities for improving the effectiveness of the directory system, and offer a new perspective for both health consumers and professionals to understand relevant health information.
Research method
The MedlinePlus portal was selected for this study since it represents reliable and up-to-date health information on a wide range of health professionals and health consumers. The health topics-based subject directory of MedlinePlus is displayed in Figure 1. A health topic refers to a specific health-related issue and has an individual Web page. The Web page has a “related health topics” column located at the right side to help end-users navigate other relevant health topics. In addition, such relationship between a topic and one of its related topics is directional, which provides an ideal condition to apply the social network analysis method.
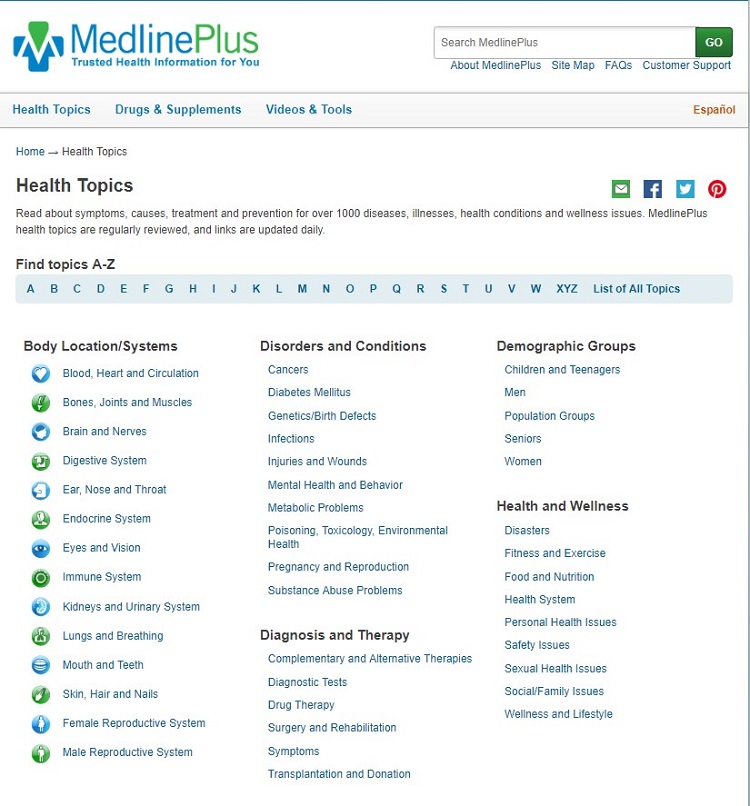
The connections among the health topics are important for the study. They were used to construct the networks. The relationship or connection between two health topics was determined by two methods: the structural connection was determined by embedded links between the two topics while the semantic connection was defined by the similarity of the two topics' Web page content.
To be more specific, in this study, all the connections among these health topics were extracted and generated from two perspectives: structural and semantic. The structural connections refer to the fact that one topic is listed as a “related health topic” to another topic. The structural connections form a structural network. The structural network was manually set up by the portal creators and enables end-users to navigate the Website. The semantic connections refer to the semantic strength between two topics' Web page content. The semantic connections form a semantic network. On a well-designed subject directory, the semantic relationship should serve as the fundamental support for building a structural link network. Theoretically, the two networks should be consistent. Otherwise, end-users might lose vital related information or be guided to inappropriate pages.
Data preparation, processing, and organisation
The selected health topic was Mental Disorders – it is a topic under the section of Disorders and Conditions, and the subcategory Mental Health and Behaviour. This topic and its related topics were collected to form the first level of health topics as Level 1. After that, related health topics were generated from each of the health topic at Level 1 to form the second level as Level 2. Such data collection process repeated until the fourth level was reached. After the process, ninety-nine health topics were included in total. Meanwhile, Web pages of all the involved four levels of health topics were gathered and the text on these Web pages was extracted to form a word list.
This word list was cleaned. First, a stop-word list was applied to filter the word list to remove redundant words. These stop-words mainly included those which only function from the grammar aspect, such as a, an, the, with. Second, synonyms were combined; for instance, anorexia nervosa, binge eating, and bulimia were combined into eating disorders. Third, all the words on the list were kept as their regular form. Different forms were normalised. For example, psychotherapies was converted to psychotherapy.
In the next stage, two matrices were built to represent the structural link network and the semantic network. The first matrix was a topic-topic link matrix (TTLM). It referred to the structural relationship among the collected health topics. In this matrix, t referred to the fact whether a health topic was listed as a related health topic of another health topic, n represented the number of health topics selected from the subject directory (hence n = 99) and the matrix itself was a n×n asymmetrical matrix. The cell value of t within the matrix indicated the relationship between two health topics – if topic i was listed as one of the related health topics of topic j, the cell value tij was assigned as 1, otherwise it was assigned as 0. Here, the matrix is asymmetrical because the fact that topic i is a related health topic of topic j did not ensure that topic j would be a related health topic of topic i (Equation (1)).
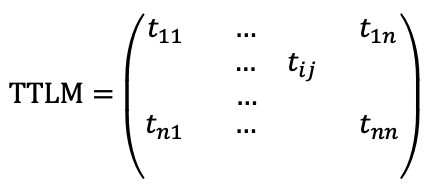
Another equation that could be generated for the topic-topic link matrix is displayed below. It indicates that a health topic could not include itself as a related health topic (Equation (2)).
tii = 0, 1 ≤ i ≤ n
Equation (2)
The other matrix created was a topic-keyword matrix (TKWM). In this matrix, each row referred to a health topic while each column referred to a keyword from the word list generated previously. The cell values, unlike those in the topic-topic link matrix, were representing the degree to which keyword j related to topic i. This degree was determined by the weight, i.e., the term frequency (tf) of the keyword in the corresponding topic's Web page. Equation (3) was built for the topic-keyword matrix and is shown below: h was the frequency number of a keyword that appeared in a health topic's Web page. n was the number of the selected health topics while m was the number of the keywords contained in the word list that were extracted from the health topic Web pages.
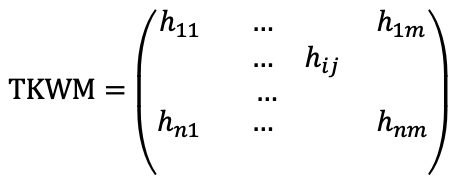
When calculating the term frequency in the topic-keyword matrix, a cut-off point of two was applied to further filter the dataset. For those words holding a cell value of one in the matrix, it indicated that they appeared only in a single health topic's Web page. That means they were not able to provide sufficiently semantic connection between two or more health topics. Hence, a word should at least have a cell value that was equal to or larger than two to be kept in the matrix. Furthermore, among the remaining words, some were eliminated still if they only occurred in a single health topic's Web page no matter how large the cell value was since there would be no semantic connections from other topics.
In the last stage, a topic-semantic matrix was built to represent the semantic network among the selected health topics through similarity measure based on the term frequency data in the topic-keyword matrix. The topic-semantic matrix is displayed in Equation (4). In this Equation, s referred to the similarity value between two health topics and n referred to the number of selected health topics. For similarity measure, the cosine-similarity measure was used in this study and displayed in Equation (5). This Equation aimed to find how similar two health topics' Web pages were based on their textual information. In this Equation, h represented the frequency number of a keyword while n referred to the number of selected health topics. Each cell value s in Equation (4) was calculated based on Equation (5). The cell value was between zero to one, in which zero indicated that there was no similarity between two health topic's Web page content while one indicated that the two Web pages were the same. The cosine-similarity measure has been widely applied to the information retrieval area (Baeza-Yates and Ribeiro-Neto, 1999; Zhang and Rasmussen, 2001) due to the fact that for documents containing the same distributed or proportional weighted keywords, the cosine-similarity measure can be an effective tool in identifying their similarities (Korfhage, 1997).
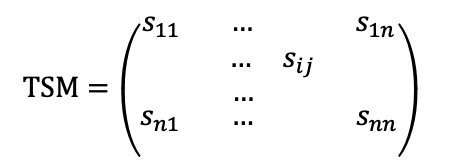
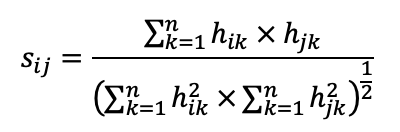
Based on Equation (5), the cell value (similarity) between topic i and topic j should stay the same as that between topic j and topic i. That meant the topic-semantic matrix was a symmetrical matrix. Moreover, the topic-semantic matrix was not directional since two topics share the same similarity in both directions from the semantic aspect.
The topic connection recommendation is described as follows. After the similarities among all the health topics on the semantic network were calculated, each edge on the semantic network had a similarity value. All edges were partitioned into one of 3 edge sets: Edge Set A, Edge Set B, and Edge Set C. Edge Set A contained the edges in which a topic was linked to itself. The similarity value between a topic and itself is always equal to one. Since these edges made no contribution to later analysis, they were excluded. If an edge could find that its corresponding link existed on the structural link network, it was put in Edge Set B. If an edge could not find that its corresponding link existed on the structural link network, it was put in Edge Set C.
The average similarity among those edges in Edge Set B was calculated and it was used as the threshold to choose recommended topic edges for the structural link network. This process is called the optimisation of the structural link network. If the similarity value of an edge in Edge Set C was larger than the threshold, the corresponding edge/link was recommended to add to the structural link network. Moreover, among the recommended edges in Edge Set C, there were two scenarios: 1) there was not any connection between two topics, T1 and T2, on the structural link network; 2) there was only a single connection from topic T1 (T2) to topic T2 (T1) on the structural link network. Both cases were considered. In conclusion, the similarity value of an edge in Edge Set C being larger than the threshold indicates that the recommended edge did not exist on the structural link network, but its similarity was larger than the average similarity value of the edges on the structural link network. Hence, the recommended edge should be added to the structural link network.
As a result, the recommended edges formed a new set Edge Set D. This Edge Set D was a subset of Edge Set C. It is clear that after the process the optimised or finalised structural link network consisted of both Edge Set B and Edge Set D.
Results and analysis
Descriptive information of the health topic directory
The data were gathered in September 2018. Among the ninety-nine selected health topics, level 1 contained twenty topics (including Mental Disorders itself), and level 2 included 11 new health topics. The third level contained twenty health topics while the fourth level had forty-eight topics. The data were processed using UciNet (Version No. 6.669) and SPSS (Version No. 25.0).
Meanwhile, text was extracted from the introduction pages of each of the ninety-nine topics. A validation process was conducted and all the stop-words were removed. Consequently, 2,441 keywords were left. In the next step, two filtering processes followed: those keywords that only appeared once were eliminated, leaving 1,413 keywords (cut-off point =1). For those keywords that appeared more than once, they were ensured to have appeared in more than one topic's page, otherwise they were excluded as well. As a result, 1,211 keywords were found to appear in at least two different health topics' pages. Finally, when the keywords collection was adjusted further in cut-off points 2 and 3 to pursue more precise similarity measurement results, the keyword count was 934 and 762, respectively. The analytical results showed that the similarity data between cut-off points 2 and 3 were extremely close; hence the outcome of cut-off point 2 was selected to involve a wider keyword range.
Based on the topics identified, two networks were built to operate the social network analysis – one of them referred to the structural link network while the other referred to the semantic network. The numbers of the nodes (ninety-nine) for both networks were the same. However, the cell value on the structural link network represented the structural connections while the cell value on the semantic network represented the semantic connections. Hence, the number of edges investigated on these two networks were different – 260 edges were found on the structural link network and 9,700 edges were found on the semantic network.
Visual display of the link network
A visualised figure of the structural link network is displayed in Figure 2. In this figure, some of the edges between two health topics were unidirectional while others were bidirectional. Moreover, the health topics were not evenly distributed on the visualised network. Therefore, the selected ninety-nine health topics could be classified into three groups: the first group was titled specific mental-related disease group and it included topics about specific mental-related diseases and disorders such as Mental Disorders, Post-traumatic Stress Disorder (PTSD). The second group was defined as health consumer-related group since it included topics that were associated with some health consumer groups like Child Health, Teen Health. It is interesting that most topics in this group were related to teenagers and children, which indicated that these two types of health consumers might have a stronger connection to mental health related issues. The last group was labelled daily health element group. This group consisted of several topics that represented components for daily health, for instance, Nutrition, Diets, Calcium. Part of the daily health element group was isolated. There was a sub-group containing the topic Nutrition located at the right side of the figure. Note that a health topic might belong to multiple groups - some topics like Child Nutrition and Teen Mental Health were considered to be included in two groups and such overlapped topics were serving as the bridging topic to link two groups.
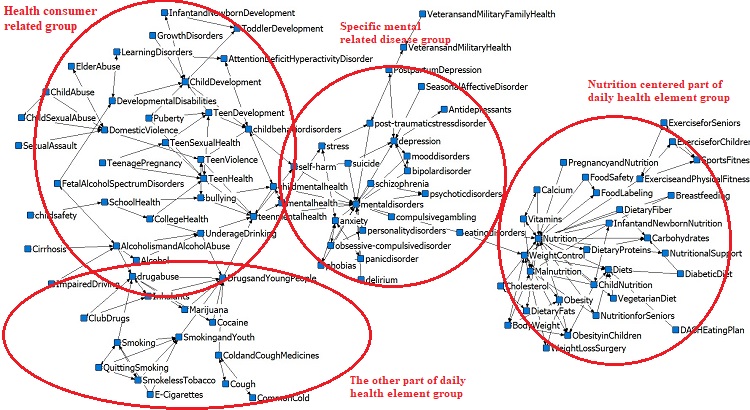
Figure 2: The display of the structural link network and the three groups of health topics
Bidirectional relationships aid navigation
To generate the semantic network, the cosine similarity measure was performed to check how similar two health topics' pages were based on their word frequency contained in the text. As a result, there were 260 edges built in the category of MedlinePlus (Edge Set B) and the average similarity value was 0.383677 while the average similarity among the rest of the 9442 pairs of topics (Edge Set C) was 0.098230. The overall average similarity was 0.105879.
Table 1. Summary of the cosine-similarity measure results | Health topics with link connections – Edge Set B (260) | Health topics without link connections – Edge Set C (9442) | All health topics (9702) |
---|---|---|---|
Average similarity | 0.383677 | 0.098230 | 0.105879 |
Standard Deviation of similarity | 0.195807 | 0.084155 | 0.100205 |
Among the 9,442 comparisons that did not appear on the structural link network, 133 pairs of topics were found to have a similarity value larger than the threshold generated from the structurally connected topics. Among these 133 pairs of topics, 110 pairs fit into the first scenario described in the methodology section, hence they were recommended to set up bidirectional connections and these pairs of edges are listed in Table 2. To be more specific, due to the word limitation, Table 2 only contains twenty out of the 110 pairs of the edges. For a complete list of the 110 bi-directional connections, please check the complete version of Table 2 in Appendix A. Meanwhile, since these comparisons included the same health topics in both directions, these related topics are displayed only once in the table to save space.
Topic A | Topic B | Similarity(cut-off=2) | Topic A | Topic B | Similarity(cut-off=2) |
---|---|---|---|---|---|
Child development | Child mental health | 0.735 | Child abuse | Child development | 0.531 |
Child safety | Child mental health | 0.449 | Child sexual abuse | Child development | 0.575 |
Learning disorders | Child mental health | 0.41 | Teenage pregnancy | Teen development | 0.509 |
Toddler development | Child mental health | 0.394 | Teen health | Body weight | 0.41 |
Obesity in children | Child mental health | 0.451 | Weight loss surgery | Diets | 0.506 |
Among these suggested bidirectional edges displayed on the table and the visualised network, some of them were straightforward since many paired topics had shared common words in their titles. Those shared title words indicated some linkages regarding either the health conditions or the related health consumer groups. For instance, Child Development and Child Mental Health shared a 0.735 similarity value in their textual content, which indicated that these two health topics had a lot of information in common from the semantic perspective. Similar cases also included Child Sexual Abuse and Child Mental Health. However, a few edges suggested, based on high similarity values, it might not be that clear compared with the previous examples. One example would be Exercise for Seniors and Exercise for Children: these two topics had a similarity value of 0.669, which might be caused by their common sections of text introducing exercise, while they were actually targeting different health consumer groups. However, there could be another circumstance worth noticing when considering the fact that users checking on information from MedlinePlus might be the family member that is responsible to take care of the whole family (e.g., a mother). If that is the case, such structural linkages set for the same daily health topic among various health consumer groups might be of great help. Therefore, such bidirectional linkages were still recommended.
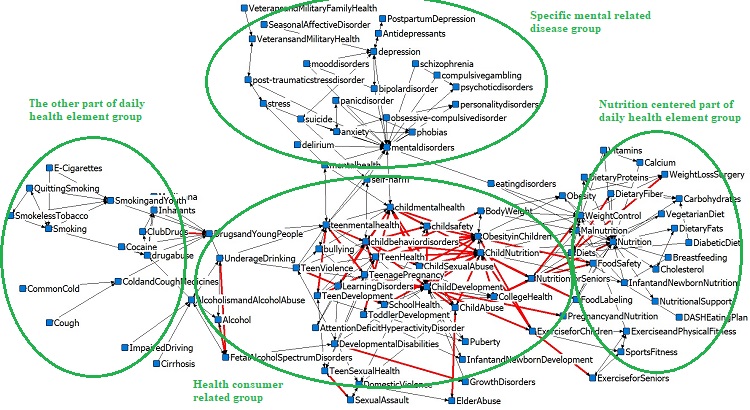
Figure 3. The display of link network after adding the suggested fifty-five bidirectional edges
A combined network consisted of the existing edges as well as the fifty-five suggested bidirectional edges is visualised and displayed in Figure 3. The recommended edges were marked in thick red lines while others were in thin black lines. The three health topic groups were highlighted in green circles. The first result that could be generated from this figure was that the area of the specific mental related disease group had no new edge to be added as a suggestion. This indicated that relationships built within this group of health topics were sound. Unlike the specific mental related disease group, the red lines in the health consumer related group were crowded, especially for the topics containing children and teenagers. These health consumer related topics included not only the mental health field, but also other specific issues such as sexual abuse and safety. The last observation was that a few recommended edges were also found in health topics like Nutrition, Diets, Alcohol. from the daily health element group.
Unidirectional relationships aid navigation
In addition to the 110 pairs of bidirectional edges discussed above, there were another twenty-three pairs of health topics sharing a similarity value that was higher than the threshold. The difference was that these twenty-three pairs of the topics fit into the second scenario illustrated in the methodology section. In other words, these health topics had already been linked from one direction on the structural link network. Hence, users would not be able to return to the previous topic. These health topics as well as their similarity measurement results are shown in Table 3. To be more specific Table 3 only contains six out of the twenty-three pairs of the unidirectional edges. For a complete list of the connections, please check the complete version of Table 3 in Appendix B.
Topic | Related topic | Similarity(cut-off=2) | Topic | Related topic | Similarity(cut-off=2) |
---|---|---|---|---|---|
Child mental health | Teen mental health | 0.472 | Quitting smoking | Smoking and youth | 0.746 |
Bipolar disorder | Mood disorders | 0.465 | Smokeless tobacco | Smoking and youth | 0.499 |
Veterans and military family health | Veterans and military health | 0.492 | Child abuse | Domestic violence | 0.504 |
The twenty-three pairs of unidirectional edges could be divided into two types: one type indicated a hierarchy relationship that was from general to specific or vice versa, such as Diets and Vegetarian Diet, Nutrition and Child Nutrition; the other type referred to a semantic relevance relationship, for example, Child Mental Health and Teen Mental Health, Food Labeling and Food Safety. Both types of the unidirectional edges were recommended to be adjusted to bidirectional edges for navigating purposes.
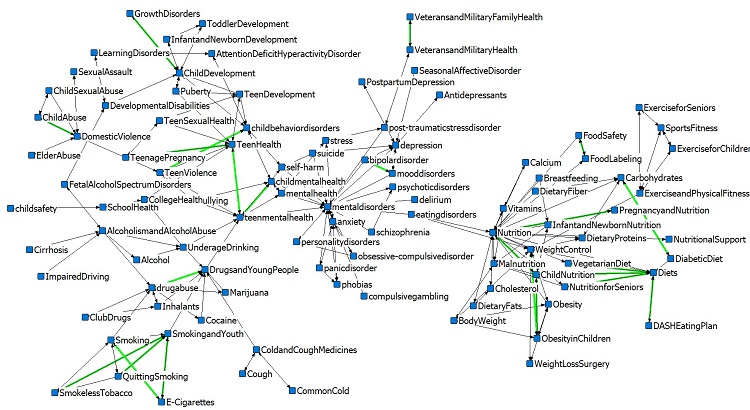
Figure 4. The display of structural link network after adding twenty-three unidirectional edges
A visual display combining the existing connections and the suggested twenty-three pairs of unidirectional edges is shown in Figure 4. The recommended unidirectional edges were marked in thick green lines while others were in thin black lines. In this figure, the suggested unidirectional edges had appeared in all three groups. To be more specific, health topics in the specific mental related disease group only had a few recommended edges, which suggested that the structure of this group had been well developed. This finding echoed the previous bidirectional result section. Meanwhile, the topics in the health consumer related group included most of the twenty-three pairs of unidirectional edges. For the daily health element group located at the lower left side and right side of the figure, the figure showed that the group needed more unidirectional edges than bidirectional edges since most of the connections had been identified in one way already. It indicated that the edges among the health topics in this group had been well recognised by the portal, hence the suggestions here focused more on the navigating purposes.
In the final stage, Figure 5 shows the complete recommended structural link network after adding both the fifty-five bidirectional edges and the twenty-three unidirectional edges to the original structural network in the MedlinePlus portal. In this figure, black lines refer to the original connections, red lines refer to the bidirectional connections, and green lines refer to the unidirectional connections. All the green lines were overlapping with their corresponding black lines to indicate an opposite direction towards the existing black lines. Moreover, the green lines were distributed more evenly than the red lines on the network.
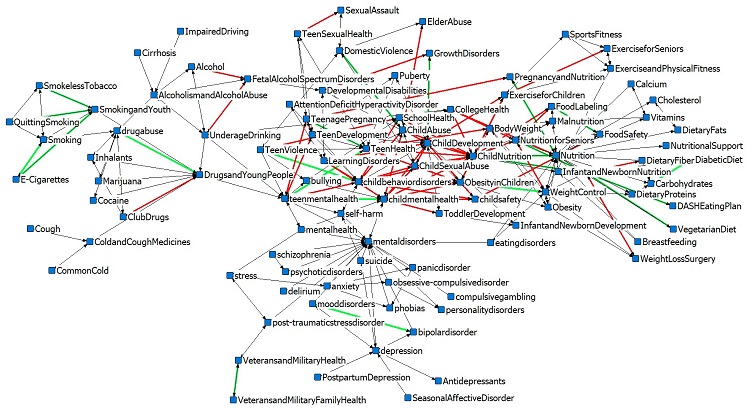
Figure 5. The display of the recommended structural link network
Recommendation result evaluation
To verify the effectiveness of the new recommended structural link network, a T-test was applied to the following hypothesis:
H0: There is no significant difference between the original structural link network and the recommended structural link network in terms of the topic similarity.
The result of the T-test is shown in Table 4. The null hypothesis was rejected (p<0.05) and there was a statistically significant difference between the original structural link network and the recommended structural link network in terms of the topic similarity. The mean similarity after the optimisation process was 0.42104, which was higher than the previous mean value (0.38368). Consequently, the suggestion proposed had indeed improved the connections among the involved health topics.
Similarity before optimisation | Similarity after optimisation | |
---|---|---|
Mean | 0.383677 | 0.42104 |
Standard Deviation | 0.195807 | 0.174835 |
df | 651 | |
t-value | -2.547 | |
p-value | 0.011 |
Meanwhile, two evaluators were invited to assess the optimisation results. Both evaluators were recruited from a formal research institute in the United States; they are research fellows in the Department of Dermatology of the University of California-Davis. They hold a Master's degree and a Doctoral degree in Predictive Medicine and they have five to ten years of experience in the field. These two domain experts are not the authors of this paper.
A list that contained 144 paired health topics was generated. Besides those combined seventy-eight pairs of recommended topics, an additional sixty-six pairs of health topics without structural edges were also evaluated for comparative purposes. The 144 pairs of topics were ordered alphabetically to avoid any potential bias caused by identifying bidirectional or unidirectional edges. A screenshot of a health topic's page including its related health topics list, along with a brief introduction about how the MedlinePlus portal creates and displays structural linkages among related health topics were provided to the evaluators. The evaluators were then asked to identify and mark the pairs of health topics that they considered as related. As a result, one evaluator confirmed sixty-eight pairs out of the 144 connections listed (47.22%) while the other evaluator confirmed seventy-one pairs (49.31%).
A Kappa test was performed between the two evaluation results and the measure of agreement value was 0.847 (p < 0.001), which indicated that a substantial agreement had been reached between the two evaluators. Then another Kappa test was employed to examine the consistency between the combined evaluation list from the two evaluators and the corresponding recommended results proposed by this study. The measure of agreement value was 0.819 (p < 0.001), which also achieved a nearly perfect agreement. Next, a Chi-squared test was employed to compare the combined evaluation list from the two evaluators and the corresponding recommended results proposed by this study. The Pearson Chi-squared value, df, and p-value were 0.125, 1, and 0.723, respectively. The test results showed there was no significant difference between the results generated by this study and the two evaluators. It suggested that the recommended results in this study were consistent with those from the expert evaluators.
Discussion
Health consumer related group
From the results generated and displayed, most suggested edges, including both unidirectional and bidirectional edges, were among the topics in the health consumer related group. With the exception of a few topics about pregnant women, veterans and their family, there were two primary types of health consumers involved in the majority of health topics in this group: teenagers and children. Although these teenagers- and children-related topics had formed a clear health consumer related group, the relationships among the topics had not been properly reflected in MedlinePlus.
These paired relationships might be questioned in two perspectives. (1) From the semantic perspective, some pairs of topics such as Child Development and Child Mental Health were not linked in the portal though they shared an extremely high similarity value in their Web page content. Hence, these topic pairs should be connected to make their semantic and structural relationships consistent. (2) From the structural perspective, the original links built in MedlinePlus did not function effectively since several paths between the two parent-child health topics were one-way. Hence portal users had no direct way to navigate back to its upper level page on the subject directory.
Specific mental related disease group
Another interesting finding related to the original structural network was that the MedlinePlus portal had no consistent standards when deciding if a bi- or unidirectional relationship should be built, no matter if it was a hierarchy or a semantic relevance relationship, the reason for creating a bidirectional linkage was not clear. For instance, Stress and PTSD had been listed as related health topics for each other and this was a typical hierarchy relationship because PTSD is a representative disorder related to Stress. However, for a similar relationship between Veterans and Military Health and Veterans and Military Family Health, the connection was set to be unidirectional, thus causing the Web page of Veterans and Military Family Health to become a dead end on the subject directory.
As a comparison, the health topics contained in this group had shown a complete and well-designed structural network that nearly perfectly fit into the semantic connections: all the pairs of health topics that shared high similarity values had been linked bidirectionally and only a few unidirectional connections might need to be adjusted to be linked to each other.
Daily health element group
Compared to the specific mental related disease group and the health consumer related group, the topics in the daily health element group were different. Unlike those two groups, which were located together, the daily health element group formed two sub-groups and linked to the other two major groups. In Figure 4, one of the subgroups including Smoking, Drug Abuse. is linked to the specific mental related disease group through the bridging topic Teen Mental Health, while the other subgroup that consisted of Nutrition, Diets., was connected to the health consumer related group through the bridging topics Child Development and Child Nutrition.
From earlier studies (Brown et al., 1996), the connection between the Smoking and Drug Abuse centred subgroup and Mental Disorder through the bridging topic Teen Mental Health made sense. This conclusion was echoed by some other studies since they regarded adolescence and young adulthood as critical timing for the appearance of mental health problems (Burns et al., 2010). On the other hand, the other subgroup which included Nutrition and Diets seemed to be far from the topics contained in the specific mental related disease group and the health consumer related group. This fact of isolation was also reflected and discussed on the original structural link network displayed in Figure 2 and the corresponding analysis.
Control of the recommended edges/links
In this study, the average similarity of the edges on the original structural link network was used as the threshold to determine or control the recommended edge set to the original structural link network. The rationale is that the similarities of the recommended links should have more close semantic relationships than the average links on the original structural link network. The threshold is adjustable. As the threshold increases, more edges are filtered out and the number of the recommended edges decreases, and vice versa. As the threshold increases, less relevant edges are removed from the recommended edge set. As a result, the optimisation results would get better. On the other hand, if the size of the recommended edge set is too small, reducing the threshold can result in increase of the recommended edge set.
Implication of the study
The practical implication of this study is that the research method could be utilised in similar Web portals which use a subject directory system. A subject directory has been recognised as a vital and effective mechanism for browsing and navigating a Web portal. It is feasible to apply a similar research method to different domains such as medical, agricultural and artistic, to generate sound results.
The theoretical implication of this study is that social network analysis has been proved to be effective for assessing and improving subject directory systems for health Web portals like the MedlinePlus. Moreover, the visualised social networks can help people identify clustering patterns hidden in the connections. The combination of research methodologies utilised in this study provides a novel way for subject directory optimisation. These findings generated through social network analysis regarding the subject directory system of MedlinePlus suggest that the social network analysis method is reliable and sound for optimising a subject directory like that on MedlinePlus. It can effectively discover missing links or connections in an existing subject directory and improve its structure by adding more reasonable links or connections. Social network analysis can be applied to a variety of domain situations.
Limitations
One limitation of this study was not recommending the removal of the currently linked edges with low sematic relationships. Even though, based on the semantic relationships identified and calculated, new edges were recommended to be added to the original structural link network, those edges from similarities of the health topic edges on the original structural link network that were very low were not recommended to be removed.
Conclusion
Public health portals have become a vital source for health consumers to seek health information. Among these portals, the MedlinePlus portal has earned a global reputation due to its accurate, up-to-date, and authoritative health information. The subject directory system of the MedlinePlus portal offers a convenient mechanism for browsing.
Subject directories matter because they help address information seeking needs in a way that a query search may fail because they give users a starting point when they have no idea or lack prior knowledge toward their search target They provide information from general level to specific, or vice versa. Moreover, subject directories offer users relevant information that they might not have thought of before the search.
This study explored the MedlinePlus portal's subject directory system and investigated the consistency between the structural and semantic connections among ninety-nine health topics that are related to mental health. Based on the semantic relationships calculated by the cosine similarity measurement, fifty-five bi-directional and twenty-three unidirectional edges were recommended to the MedlinePlus portal creators to be added to the original structural link network in order to optimise and enhance its accuracy and effectiveness. A statistical evaluation of the recommended new structural link network has shown a significant improvement in the accuracy and effectiveness of the directory. To be more specific, after adding the recommended connections to the original subject directory system related to mental health, the subject directories were found to have a significantly stronger consistency between the structural and semantic relationships among the involved health topics. In other words, the portal users are now able to explore more health topics that are semantically relevant to each other. Moreover, this improved directory was evaluated by two domain experts and shown to be improved. In addition to improving the directory this study found that teenagers and children have been identified to be the most related health consumer groups to mental health. It also discovered that mental health was found to be related to some daily health issues, such as nutrition, diets and smoking.
The future research directions include, but are not limited to, applying the social network analysis method to a wider range of subject directory systems in health portals or health information systems. Meanwhile, more elements of the social network analysis methodology, such as closeness, betweenness, and density could be used in similar studies.
Acknowledgements
The authors appreciate the regional editor, the copy-editor, and the anonymous reviewers for their insightful comments and constructive suggestions.
Ethics approval This study was approved by the Institutional Review Board at the corresponding institution.
About the author
Yifan Zhu is a doctoral candidate from School of Information Studies at the University of Wisconsin Milwaukee, U.S.A.. He received his Bachelor's degree from Sichuan University, China, and his Master's degree from the University of Wisconsin Madison. His research interests include information retrieval, information seeking behaviour, health information, and information ethics and policies. He can be reached at yifanzhu@uwm.edu
Jin Zhang is a Professor of School of Information Studies, the University of Wisconsin Milwaukee, U.S.A.. He received his Bachelor's degree and Master's degree from Wuhan University in China, and Doctoral degree from the University of Pittsburgh. His research interests include information retrieval, information visualization, consumer health informatics, social media, data mining, search engine evaluation, and metadata. He can be contacted at jzhang@uwm.edu
References
Note: A link from the title is to an open access document. A link from the DOI is to the publisher's page for the document.
- Baeza-Yates, R., & Ribeiro-Neto, B. (1999). Modern information retrieval. ACM Press and Addison-Wesley
- Baird, A., Furukawa, M. F., & Raghu, T. S. (2012). Understanding contingencies associated with the early adoption of customer-facing Web portals. Journal of Management Information Systems, 29(2), 293–324. https://doi.org/10.2753/MIS0742-1222290210
- Barker, A. (2019) Navigating life: a taxonomy of wayfinding behaviours. Journal of Navigation, 72(3), 539–554. https://doi.org/10.1017/S0373463319000043
- Barnes, J. A. (1954). Class and committees in a Norwegian island parish. Human Relations, 7(1), 39–58. https://doi.org/10.1177/001872675400700102
- Bates, M. J. (1989). The design of browsing and berrypicking techniques for the online search interface. Online Review, 13(5), 407–424. https://doi.org/10.1108/eb024320
- Borgatti, S. P., Mehra, A., Brass, D. J., & Labianca, G. (2009). Network analysis in the social sciences. Science, 323(5916), 892–895. https://doi.org/10.1126/science.1165821
- Brown, R. A., Lewinsohn, P. M., Seeley, J. R., & Wagner, E. F. (1996). Cigarette smoking, major depression, and other psychiatric disorders among adolescents. Journal of the American Academy of Child and Adolescent Psychiatry, 35(12), 1602–1610. https://doi.org/10.1097/00004583-199612000-00011
- Burns, J. M., Davenport, T. A., Durkin, L. A., Luscombe, G. M., & Hickie, I. B. (2010). The Internet as a setting for mental health service utilisation by young people. Medical Journal of Australia, 192, S22–S26. https://doi.org/10.5694/J.1326-5377.2010.TB03688.X
- Choo, C. W., Detlor, B., & Turnbull, D. (2000). Information seeking on the Web: an integrated model of browsing and searching. First Monday, 5(2), 3–16. https://doi.org/10.5210/fm.v5i2.729
- Chung, Y. M., & Noh, Y. H. (2003). Developing a specialized directory system by automatically classifying Web documents. Journal of Information Science, 29(2), 117-126. https://doi.org/10.1177/016555150302900204
- Coleman, J. S. (1986) Social theory, social research, and a theory of action. American Journal of Sociology, 91(6), 1309–1335. https://doi.org/10.1086/228423
- Conner, K. O., Copeland, V. C., Grote, N. K., Koeske, G., Rosen, D., Reynolds, C. F., & Brown, C. (2010). Mental health treatment seeking among older adults with depression: the impact of stigma and race. The American Journal of Geriatric Psychiatry, 18(6), 531–543. https://doi.org/10.1097/JGP.0b013e3181cc0366
- Demelo, J., Parsons, P., & Sedig, K. (2017). Ontology-driven search and triage: design of a Web-based visual interface for MEDLINE. JMIR Medical Informatics, 5(1), e4. https://doi.org/10.2196/medinform.6918
- Evers, K. E. (2006). eHealth promotion: the use of the Internet for health promotion. American Journal of Health Promotion, 20(4), 1–14. https://doi.org/10.4278/0890-1171-20.4.1
- Fergie, G., Hilton, S., & Hunt, K. (2016). Young adults' experiences of seeking online information about diabetes and mental health in the age of social media. Health Expectations, 19(6), 1324–1335. https://doi.org/10.1111/hex.12430
- Fox, S., & Rainie, L. (2000). The online health care revolution: how the Web helps Americans take better care of themselves. Pew Research Center. http://www.medicalmarketingmatters.com/wp-content/uploads/PIP_Health_Report.pdf.pdf (Archived by the Internet Archive at https://bit.ly/2Il9pCk)
- Gray, N. J., Klein, J. D., Noyce, P. R., Sesselberg, T. S., & Cantrill, J. A. (2005). Health information-seeking behaviour in adolescence: the place of the Internet. Social Science & Medicine, 60(7), 1467–1478. https://doi.org/10.1016/j.socscimed.2004.08.010
- Halpert, A., Dalton, C. B., Palsson, O., Morris, C., Hu, Y., Bangdiwala, S., Hankins, J., Norton, N., & Drossman, D. (2007) What patients know about irritable bowel syndrome (IBS) and what they would like to know. National survey on patient educational needs in IBS and development and validation of the patient educational needs questionnaire (PEQ). The American Journal of Gastroenterology, 102(9), 1972–1982. https://doi.org/10.1111/j.1572-0241.2007.01254.x
- Horgan, Á., & Sweeney, J. (2010). Young students' use of the Internet for mental health information and support. Journal of Psychiatric and Mental Health Nursing, 17(2), 117–123. https://doi.org/10.1111/j.1365-2850.2009.01497.x
- Johnson, J. D., & Case, D. O. (2012). Health information seeking. Peter Lang.
- Johnston, K. (2006). What are subject directories? Board of Trustees of the University of South Carolina. https://www.tcd.ie/Education/ICT/unit02/demonstration03.htm (Archived by the Internet Archive at https://bit.ly/2Il9KoA)
- Kessler, R. C., Berglund, P., Demler, O., Jin, R., Merikangas, K. R., & Walters, E. E. (2005). Lifetime prevalence and age-of-onset distributions of DSM-IV disorders in the national comorbidity survey replication. Archives of General Psychiatry, 62(6), 593. https://doi.org/10.1001/archpsyc.62.6.593
- Kim, E., Hou, J., Han, J. Y., & Himelboim, I. (2016). Predicting retweeting behavior on breast cancer social networks: network and content characteristics. Journal of Health Communication, 21(4), 479–486. https://doi.org/10.1080/10810730.2015.1103326
- Korfhage, R. R. (1997). Information storage and retrieval. Wiley Computer Pub.
- Medical Library Association. (n.d.). For health consumers and patients: top health Websites. https://www.mlanet.org/page/top-health-Websites (Archived by the Internet Archive at https://bit.ly/38B3eoP)
- Mertens, F., Saint-Charles, J., & Mergler, D. (2012). Social communication network analysis of the role of participatory research in the adoption of new fish consumption behaviors. Social Science & Medicine, 75(4), 643–650. https://doi.org/10.1016/j.socscimed.2011.10.016
- Miller, N., Lacroix, E. M., & Backus, J. E. (2000). MEDLINEplus: building and maintaining the National Library of Medicine's consumer health Web service. Bulletin of the Medical Library Association, 88(1), 11–17. http://www.ncbi.nlm.nih.gov/pubmed/10658959 (Archived by the Internet Archive at https://bit.ly/32pALym)
- Möller, J., van de Velde, R. N., Merten, L., & Puschmann, C. (2019). Explaining online news engagement based on browsing behavior: creatures of habit? Social Science Computer Review, 38(5), 616-632. https://doi.org/10.1177/0894439319828012
- Morahan-Martin, J. M. (2004). How Internet users find, evaluate, and use online health information: a cross-cultural review. CyberPsychology & Behavior, 7(5), 497–510. https://doi.org/10.1089/cpb.2004.7.497
- Otte, E., & Rousseau, R. (2002). Social network analysis: a powerful strategy, also for the information sciences. Journal of Information Science, 28(6), 441–453. https://doi.org/10.1177/016555150202800601
- Pang, P. C. I., Chang, S., Verspoor, K., & Pearce, J. (2016). Designing health Websites based on users' Web-based information-seeking behaviors: a mixed-method observational study. Journal of Medical Internet Research, 18(6), e145. https://doi.org/10.2196/jmir.5661
- Pang, P. C. I., Harrop, M., Verspoor, K., Pearce, J., & Chang, S. (2016). What are health Website visitors doing? Insights from visualisations towards exploratory search. In Proceedings of the 28th Australian Computer-Human Interaction Conference, OzCHI 2016, (pp. 631–633). Association for Computing Machinery. https://doi.org/10.1145/3010915.3011843
- Paolino, L., Genser, L., Fritsch, S., de' Angelis, N., Azoulay, D., & Lazzati, A. (2015). The Web-surfing bariatic patient: the role of the Internet in the decision-making process. Obesity Surgery, 25(4), 738–743. https://doi.org/10.1007/s11695-015-1578-x
- Postigo, H. (2011). Questioning the Web 2.0 discourse: social roles, production, values, and the case of the human rights Portal. Information Society, 27(3), 181–193. https://doi.org/10.1080/01972243.2011.566759
- Pow, J., Gayen, K., Elliott, L., & Raeside, R. (2012). Understanding complex interactions using social network analysis. Journal of Clinical Nursing, 21(19pt20), 2772–2779. https://doi.org/10.1111/j.1365-2702.2011.04036.x
- Rains, S. A. (2018). Coping with illness digitally. The MIT Press. https://doi.org/10.7551/mitpress/11219.001.0001
- Schleyer, T., & Spallek, H. (2002). An evaluation of five dental Internet portals. The Journal of the American Dental Association, 133(2), 204–212. https://doi.org/10.14219/jada.archive.2002.0145
- Shevchenko, L. (2020). Analysis of library Website users' behavior to optimize virtual information and library services. Journal of Information Science Theory and Practice, 8(1), 45–55. https://doi.org/10.1633/JISTaP.2020.8.1.4
- Smith, K. P., & Christakis, N. A. (2008). Social networks and health. Annual Review of Sociology, 34(1), 405–429. https://doi.org/10.1146/annurev.soc.34.040507.134601
- Sterling, E. W., von Esenwein, S. A., Tucker, S., Fricks, L., & Druss, B. G. (2010). Integrating wellness, recovery, and self-management for mental health consumers. Community Mental Health Journal, 46(2), 130–138. https://doi.org/10.1007/s10597-009-9276-6
- Taylor, R. S. (1967). Question-negotiation and information-seeking in libraries. Lehigh University. Center for the Information Sciences. https://apps.dtic.mil/sti/citations/AD0659468 (Archived by the Internet Archive at https://bit.ly/32nl8Hm)
- Wege, C. (2002). Portal server technology. IEEE Internet Computing, 6(3), 73–77. https://doi.org/10.1109/MIC.2002.1003134
- World Health Organization. (2018). WHO Mental Disorders Fact Sheet. https://www.who.int/en/news-room/fact-sheets/detail/mental-disorders (Archived by the Internet Archive at https://bit.ly/3k8vpx9)
- Yang, H.-C., & Lee, C.-H. (2004). A text mining approach on automatic generation of Web directories and hierarchies. Expert Systems with Applications, 27(4), 645–663. https://doi.org/10.1016/j.eswa.2004.06.009
- Yang, H.-C., & Lee, C.-H. (2006). NaviSOM: automatic construction of navigational structure for Web pages using self-organizing maps. In The 2006 IEEE International Joint Conference on Neural Network Proceedings, Vancouver, BC, Canada, 16-21 July, 2006 (pp. 985–992). https://doi.org/10.1109/IJCNN.2006.246793
- Yeo, M.-H., Lee, Y.-K., Roh, K.-J., Park, H.-S., Kim, H.-S., Park, J.-H., Kang, T.-H., Kim, H.-Y., & Yoo, J.-S. (2010). Design and implementation of a directory system for disease services. International Journal of Contents, 6(1), 59–64. https://doi.org/10.5392/IJoC.2010.6.1.059
- Yonker, L. M., Zan, S., Scirica, C. V, Jethwani, K., & Kinane, T. B. (2015). "Friending" teens: systematic review of social media in adolescent and young adult health care. Journal of Medical Internet Research, 17(1), e4. https://doi.org/10.2196/jmir.3692
- Zhang, J., & An, L. (2009). Visualization relevance analysis for weight control-related subjects. Proceedings of the American Society for Information Science and Technology, 46(1), 1–5. https://doi.org/10.1002/meet.2009.145046032
- Zhang, J., & Rasmussen, E. M. (2001). Developing a new similarity measure from two different perspectives. Information Processing and Management, 37(2), 279–294. https://doi.org/10.1016/S0306-4573(00)00027-3
- Zhang, J., Wolfram, D., Wang, P., Hong, Y., & Gillis, R. (2008). Visualization of health-subject analysis based on query term co-occurrences. Journal of the American Society for Information Science and Technology, 59(12), 1933–1947. https://doi.org/10.1002/asi.20911
- Zhang, J., Zhai, S., Liu, H., & Stevenson, J. A. (2016). Social network analysis on a topic-based navigation guidance system in a public health portal.Journal of the Association for Information Science and Technology, 67(5), 1068–1088. https://doi.org/10.1002/asi.23468
- Zhang, J., Zhai, S., Stevenson, J. A., & Xia, L. (2016). Optimization of the subject directory in a government agriculture department Web portal. Journal of the Association for Information Science and Technology, 67(9), 2166–2180. https://doi.org/10.1002/asi.23550
How to cite this paper
Appendices
Appendix A: Fifty-five bidirectional pairs of health topics that require structural linkages
Topic A | Topic B | Similarity(cut-off=2) | Topic A | Topic B | Similarity(cut-off=2) |
---|---|---|---|---|---|
Child development | Child mental health | 0.735 | Child abuse | Child development | 0.531 |
Child safety | Child mental health | 0.449 | Child sexual abuse | Child development | 0.575 |
Learning disorders | Child mental health | 0.41 | Teenage pregnancy | Teen development | 0.509 |
Toddler development | Child mental health | 0.394 | Teen health | Body weight | 0.41 |
Obesity in children | Child mental health | 0.451 | Weight loss surgery | Diets | 0.506 |
Child nutrition | Child mental health | 0.59 | Dietary fiber | Diets | 0.497 |
Child abuse | Child mental health | 0.58 | College health | Nutrition | 0.429 |
Child sexual abuse | Child mental health | 0.622 | Obesity in children | Teen health | 0.389 |
Child behavior disorders | Teen mental health | 0.419 | Child nutrition | Teen health | 0.455 |
Teen development | Teen mental health | 0.661 | Fetal alcohol spectrum disorders | Alcohol | 0.606 |
Teenage pregnancy | Teen mental health | 0.463 | Food safety | Child safety | 0.552 |
School health | Child behavior disorders | 0.464 | Child nutrition | College health | 0.446 |
Child safety | Child behavior disorders | 0.402 | Nutrition for seniors | College health | 0.456 |
Learning disorders | Child behavior disorders | 0.408 | Growth disorders | Developmental disabilities | 0.595 |
Obesity in children | Child behavior disorders | 0.422 | Sexual assault | Teen sexual health | 0.623 |
Child nutrition | Child behavior disorders | 0.565 | Exercise for children | Obesity in children | 0.397 |
Child abuse | Child behavior disorders | 0.552 | Child sexual abuse | Obesity in children | 0.39 |
Child sexual abuse | Child behavior disorders | 0.569 | Nutrition for seniors | Child nutrition | 0.545 |
Nutrition for seniors | Weight control | 0.39 | Exercise for children | Child nutrition | 0.465 |
Club drugs | Drugs and young people | 0.431 | Food labeling | Child nutrition | 0.407 |
School health | Teen violence | 0.419 | Child abuse | Child nutrition | 0.434 |
Fetal alcohol spectrum disorders | Underage drinking | 0.559 | Child sexual abuse | Child nutrition | 0.471 |
Child nutrition | School health | 0.463 | Food labeling | Nutrition for seniors | 0.491 |
Child safety | Child development | 0.431 | Food safety | Nutrition for seniors | 0.455 |
Learning disorders | Child development | 0.436 | Exercise for seniors | Exercise for children | 0.669 |
Obesity in children | Child development | 0.504 | Teenage pregnancy | Pregnancy and nutrition | 0.603 |
Child nutrition | Child development | 0.649 | Elder abuse | Child abuse | 0.468 |
Exercise for children | Child development | 0.43 |
Appendix B: Twenty-three unidirectional edges recommended
Topic | Related topic | Similarity(cut-off=2) | Topic | Related topic | Similarity(cut-off=2) |
---|---|---|---|---|---|
Child mental health | Teen mental health | 0.472 | Quitting smoking | Smoking and youth | 0.746 |
Bipolar disorder | Mood disorders | 0.465 | Smokeless tobacco | Smoking and youth | 0.499 |
Veterans and military family health | Veterans and military health | 0.492 | Child abuse | Domestic violence | 0.504 |
Child behavior disorders | Teen violence | 0.392 | Teen mental health | Teen health | 0.614 |
Growth disorders | Child development | 0.442 | Teenage pregnancy | Teen health | 0.517 |
Child nutrition | Diets | 0.394 | Weight control | Obesity in children | 0.43 |
Dash diet | Diets | 0.503 | Child nutrition | Obesity in children | 0.568 |
Nutrition for seniors | Diets | 0.444 | Diabetic diet | Carbohydrates | 0.405 |
Vegetarian diet | Diets | 0.404 | Food safety | Food labeling | 0.468 |
Child nutrition | Nutrition | 0.6 | Drugs and young people | Drug abuse | 0.611 |
Pregnancy and nutrition | Nutrition | 0.41 | E-cigarettes | Smoking | 0.396 |
E-cigarettes | Smoking and youth | 0.451 |