Investigating perception biases on intellectual property search systems: a study on the priming effect
Mandy Dang, Yulei (Gavin) Zhang, and Kevin Trainor.
Introduction. This study aims to examine users’ perception biases on the use of intellectual property search systems. Particularly, the priming effect is investigated.
Method. A laboratory experiment is conducted, and two supraliminal primes are utilised. Subjects are randomly assigned to three conditions, including: (1) using an easy-to-use search system as the prime, (2) using a difficult-to-use system as the prime, and (3) a control group without any primes.
Analysis. Data analyses are performed in two steps. We first validate the setup of the two primes used in this study. After that, we examine the priming effect by comparing user perceptions of the target across the three conditions.
Results. In general, our data analysis results indicate the existence and significance of the priming effect. Specifically, users who are primed by a difficult-to-use intellectual property search system are found to have significantly more positive perceptions of the target system as compared to those who are primed by an easy-to-use system.
Conclusions. This study identifies significant priming effects in the context of intellectual property search systems.
DOI: https://doi.org/10.47989/irpaper869
Introduction
When assessing the success of using and adopting an information system or technology, one important aspect is to make sure that users possess a positive attitude toward the system or technology (DeLone and McLean, 1992, 2003; Venkatesh et al., 2003). However, users’ perceptions could be influenced by potential biases, and one type of those biases, caused by the priming effect, have caught the attention of researchers (Bargh et al., 2001; Minas and Dennis, 2019; Segal and Cofer, 1960).
Created by Segal and Cofer (1960), the term priming was used in their study to describe a situation in which users exposed to certain words in a task were more likely to use the same group of words in subsequent tasks. Over years, the priming effect has been studied by many researchers. It generally refers to the phenomena where individuals’ cognition and behaviour can be influenced by the presentation of a preceding stimulus (called a prime) (Minas and Dennis, 2019). In the current study, we aim to examine the existence and significance of the priming effect in the context of intellectual property search systems. We hope and believe the findings reported in this study could contribute to the existing literature on priming research as well as on human-computer interaction research in general.
To empirically examine the priming effect, we designed and conducted a laboratory experiment. We used supraliminal priming instead of subliminal priming, because supraliminal priming is believed to have a stronger effect (Dennis et al., 2013). Specifically, two primes were used in the study, including an easy-to-use intellectual property search system (referred to as the easy prime in the rest of the paper) and a difficult-to-use intellectual property search system (referred to as the difficult prime). Participants were randomly assigned into three conditions, including those who were exposed to the easy prime, the difficult prime, and no prime. Our data analysis results indicated the significant priming effect. Specifically, we found that users who were primed by the difficult-to-use system had significantly more positive perceptions of the target system as compared to those who were primed by the easy-to-use system.
The remainder of this paper is organized as follows. The next section presents the related literature. After that, the design of the research study is described in detail, followed by the data analysis and results. The paper concludes with discussions on research contributions and future research suggestions.
Related literature
Priming (sometimes called the priming effect) is defined as the phenomenon where a previous stimulus (that is the prime) can subconsciously influence an individual’s processing of and responses to future stimuli (that are the targets) (Hoff et al., 2016; Minas and Dennis, 2019). Priming is a psychological concept and is related to how people process information in working memory. How priming works is that if a concept presented to an individual can activate the mental representations in working memory that drive the desired behaviour, it can then influence how the individual interprets new information and thus change behaviour (Dennis et al., 2013; Minas and Dennis, 2019). In human psychology, evolution has made priming a fundamental part of our cognitive processing. Different explanations on the evolution of priming exist. Earlier, most researchers believed that priming was a memory effect (Valdez et al., 2018). Later, findings from modern neuroscience research found priming to be a result of neural pre-activation (Minas and Dennis, 2019; Valdez et al., 2018), meaning that similar stimuli were recognised easily by human beings since they could help warm up the neural correlates of those similar concepts. Context is very important in shaping people’s perception and behaviour in many cases. This is true for priming as well. Based on different contexts, people may treat the same thing differently. For example, when being presented to the same word (or sentence), children and adults would perceive it differently in terms of the levels of ease of understanding. Therefore, context plays an important role in influencing the impact of primes on individuals’ subsequent perception or behaviour toward the same target.
There are different types of priming, such as supraliminal priming (Bhagwatwar et al., 2013; Dennis et al., 2013; Li et al., 2015), subliminal priming (Chalfoun and Frasson, 2008; Pinder et al., 2017, 2018), semantic priming (Bodoff and Vaknin, 2016; Pinder et al., 2018), syntactic priming (Heyselaar et al., 2017), affective priming (D’Errico et al., 2019). Supraliminal priming and subliminal priming are opposite in concepts: supraliminal priming is above the threshold of human beings’ consciousness, while subliminal priming is below that threshold (Bhagwatwar et al., 2013). In detail, subliminal priming is defined as a situation in which participants are not aware of the existence of the stimulus; in contrast, supraliminal priming means that participants are consciously aware of the stimulus (Adomavicius et al., 2013; Dennis et al., 2013). However, in both scenarios, participants are not aware of the purpose behind them (Dennis et al., 2013).
Li et al. ( 2015) examined the effect of supraliminal priming in reducing people’s number entry errors. To implement it, they identified and used three types of primes based on the valued, or size, structure, and context of numbers, respectively. Specifically, those primes were presented to subjects as questions for them to answer before entering each number. To conduct the experiment, the authors designed three types of user interfaces, each based on one prime. Four conditions were formed, including: UI1 which had no primes, UI2 in which the priming question was for the participant to first compare the instruction number with a randomly generated number before entering the instruction number, UI3 in which the priming question was a descriptive question related to the instruction number, and UI4 in which the priming question was about the context of the number entry task. They found that all three types of primes could significantly help improve the accuracy of number entry and reduce the entry errors.
In another study, Dennis et al. (2013) investigated how a computer game based supraliminal prime could positively influence students’ test-taking performance. To do it, they designed a computer-based word game which primed the concept of achievement, with the purpose of improving an individual’s expectation of success and motivation. They found that participants who took a test (which consisted of both verbal and quantitative questions from a practice SAT (a scholastic assessment test)) immediately after playing the game outperformed those who played a placebo game. This result was found to be statistically significant on both the verbal reasoning and quantitative reasoning test questions.
Although it is agreed that the impact of subliminal priming is generally weaker than that of supraliminal priming (Dennis et al., 2013; Minas and Dennis, 2019), some researchers believe that subliminal priming also has the potential to influence people’s cognitive perceptions and behaviour, and thus making impacts in decision making. For example, Pinder et al. (2017) studied and discussed in detail the design, ethical, user acceptance, and technical issues in delivering mobile subliminal prime. They also argued that it could be more appropriate to use subliminal stimuli when people were working on tasks that required high levels of mental demand. In another study, Chalfoun and Frasson (2008) investigated how subliminal priming could influence learners’ physiological reactions in using an intelligent tutoring system in 3D virtual environments. Specifically, they empirically tested how subliminal priming could influence learner performance in constructing an odd magic square of any order by using the 3D virtual tutoring system, and found out that learners’ performance had significantly improved and the time for them to answer questions was shorter.
Semantic priming means that the priming is delivered to individuals through the use of words (Dennis et al., 2013). It occurs when an individual hears or reads a word to trigger the semantic networks in the brain in a subconscious manner, and thus activating a set of concepts related to the priming word in the individual’s working memory (Bodoff and Vaknin, 2016; Dennis et al., 2013). For example, it has been shown that when the word popcorn was first presented to individuals who were later asked to name public figures, they would be more likely to name movie stars than music stars since the prime popcorn could activate one’s semantic networks associated with movies and movie theatres (Dennis et al., 2013). A great body of existing research has been done on semantic priming. For example, Fu et al. (2010) studied the impact of semantic priming on users’ social tagging behaviour, and argued that if an individual was exposed to an existing tag (a word) before creating his/her own, the existing tag could act as a prime, and the individual would be more likely to use words that were semantically related to the prime word on subsequent tagging tasks. A similar study was conducted later by Bodoff and Vaknin (2016), with the consideration of both semantic priming and strategic influence in understanding user behaviour in social tagging.
In addition to semantic priming, another type of linguistic priming that has been examined in existing literature is syntactic priming. Syntax generally refers to the sentence structure in textual presentation, and syntactic priming means that people are influenced by and will adapt their sentence structures to match that of their partners. For example, Heyselaar et al. (2017) examined the language behaviour between human beings and computer avatars in virtual reality, with the purpose of validating that human-computer language interaction was comparable to human-human language interaction in the virtual environment. Specifically, they created and leveraged a syntactic priming task to compare participants’ language behaviour across three conditions, including working with a human partner, a human-like avatar partner (with human-like facial expressions and verbal cues), and computer-like avatar partner. The found significant and comparable priming effects in the first two conditions, but significantly decreased priming effect in the third condition. The general conclusion they made out of their study was that virtual reality was a valid platform for conducting dialogue interactions and language research at large.
Priming is also believed to be able to associate with people’s sentiment, emotions, and related behaviour. Affective priming means that if individuals are exposed to affective words (such as positive or negative ones), those priming words can activate their emotional states, which in turn, influence their subsequent judgement and behaviour (Chartrand et al., 2006; D’Errico et al., 2019; Minas and Dennis, 2019; Murphy and Zajonc, 1993). Most existing affective priming research focuses on the positive and negative emotional valence (Chartrand et al., 2006). For example, D’Errico et al. (2019) investigated the impact of affective priming (positive and negative) on prosocial orientation differences between digital natives (defined as those who could provide highly automatic and fast responses in hyper-textual environment and were generally believe to be the younger generation) and digital immigrants (defined as those who mainly focused on textual elements in the digital era and were generally believed to be the older generation) through the use of a mobile application. They found that the negative prime could influence an individual’s prosocial orientation negatively, while a positive one could influence his/her prosocial orientation in a positive way; but this finding was only statistically significant on digital natives (they used the age of 30 as the cut-off between digital natives and digital immigrants).
Priming effects have been studied in different contexts, including virtual reality (Bhagwatwar et al., 2013), visualisation (Valdez et al., 2018), artificial intelligence (Ragot et al., 2020) education (Chalfoun and Frasson, 2008; Dennis et al., 2013), etc. For example, Bhagwatwar et al. (2013) studied the priming effect of visual elements designed to improve creativity on team-brainstorming performance in virtual environments. They created two virtual offices using Open Wonderland, with one being a traditional office environment and the other one incorporating objects with different colours, shapes, and sizes (e.g., musical instruments and aircraft models) which aimed to help induce a creative work atmosphere. They found that teams that worked in the creative priming environment generated significantly more ideas and were of better quality. Valdez et al. (2018) examined the priming effect in visualisation, specifically on how users separated dots into classes in scatterplots. They found people separated the same scatterplot differently depending on the scatterplots they have seen before, indicating the existence of the priming effect. Ragot et al. (2020) investigated the priming effect in artificial intelligence vs. human beings. Specifically, they compared and tested user perception differences between paintings generated by artificial intelligence and human-created paintings. By using the declared identity of the painter (artificial or human) as the prime, they found the existence of significant priming effect, in that subjects evaluated paintings perceived as drawn by human beings significantly more highly than those made by artificial intelligence (even if the released identify was not the real case). As to education, significant priming effects also exist. For example, it was found that playing a computer game which was designed to increase an individual’s expectation of success and motivation (serving as the prime) could significantly improve students’ performance in tests and examinations (Dennis et al., 2013).
In addition to the priming effect, there is another effect which, in most cases, also can lead to human biases in their perceptions, judgment, and decisions, that is the anchoring effect (Valdez et al., 2018). The anchoring effect states that people make decisions by starting from an initial value (i.e., the anchor), based on which they will make adjustments in order to get the estimate of their final answer (Tversky and Kahneman, 1974, 1992). It is generally believed that when different anchors are provided to different people, they will make different estimates (Wu and Niu, 2015). Previous research has examined the anchoring effect in various contexts, such as visualisation (Heijden, 2013; Valdez et al., 2018), information security (Tsohou et al., 2015), e-commerce (Wu et al., 2012), operations management (D’Urso et al., 2017), and recommendation systems (Adomavicius et al., 2013).
The priming and anchoring effects share some similarities. Some psychologists treat anchoring as an instance of priming. In general, it is believed that priming is the foundation, and anchoring is based on priming in the way that it further describes the effect that judgements might be biased (or anchored) towards a preceding stimulus (Valdez et al. 2018). In addition, they also have some differences. Priming as an idea stems from cognitive sciences, while anchoring is a result from behavioural sciences. The major difference between the priming effect and the anchoring effect is that the priming effect is about the phenomenon that human responses can be influenced by a preceding stimulus, while the anchoring effect refers to the phenomenon that a previous stimulus can provide a frame of reference which then aids an individual’s judgment in later tasks. However, both effects could shed light on our understanding about certain human biases in perceptions, judgement, and decision making. Valdez et al. leveraged both effects to understand human biases in low-level visual judgement tasks. By conducting a series of empirical studies, they found that both effects existed, and both of them could influence people’s judgement.
Research study
In this study, we focus on extending our knowledge of the priming effect. As mentioned in the above section, prior research has investigated the priming effect in different contexts; however, we are aware of no studies that empirically examined its existence and potential influences in the usage of intellectual property search systems. This study aims to contribute to existing literature by addressing this gap. Inspired by previous research on supraliminal priming, we would like to address the following two research questions:
RQ1: Does the priming effect exist in the context of intellectual property search systems?
RQ2: How could a prior system (the prime) influence user perceptions of a subsequent system (the target)?
Research method and study design
To answer the research questions, we operationalise the study by conducting a laboratory experiment with the use of online patent search systems. The primes used in this study are two patent search systems, with one being relatively easy for the users to use to perform patent search tasks (the easy prime), and the other one being relatively difficult to use (the difficult prime). To conduct the experiment, we adopt a repeated-measures factor design, with the patent search system being the repeated-measures factor.
The easy prime used in this study is the Google patent search system, specifically, its advanced patent search function. As shown in Figure 1, to form a search query, users need to specify certain keywords in different text boxes, which are related to different parts of the patent document, such as patent number, patent title, patent inventor(s), classification codes, publication date, etc. The search interface design is very structured and straightforward, which could help make it easy for users to learn how to use and get familiar with using it to conduct patent search tasks. Thus, we believe it is reasonable to use it as the easy prime of this study. To further valid this, we conducted a test which is reported in the next section.
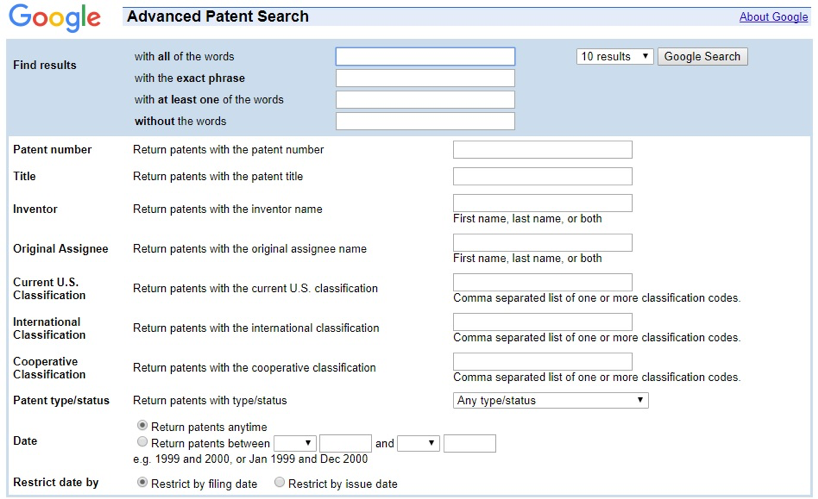
Figure 1: Google patent search system (advanced search interface)
The difficult prime used in this study is the United States Patent and Trademark Office (hereafter, the Patent Office) patent search system, particularly, its advanced search tool. Compared with the advanced search function of Google patent search, this function adopts a different interface design. To form a search query, users need to strictly follow certain syntax rules. Figure 2 shows a screenshot of it. Instead of providing various textboxes (related to different fields of the patent document) for the user to enter keywords into, this search interface provides one big, multi-line textbox for the user to enter and run the entire query. Since users need to devote more mental effort to learn and get familiar with the syntax rules in order to be able to conduct patent search tasks, we believe it is reasonable to expect that users will perceive this search function difficult to use in general. To further validate this, a test was conducted (reported in the next section).
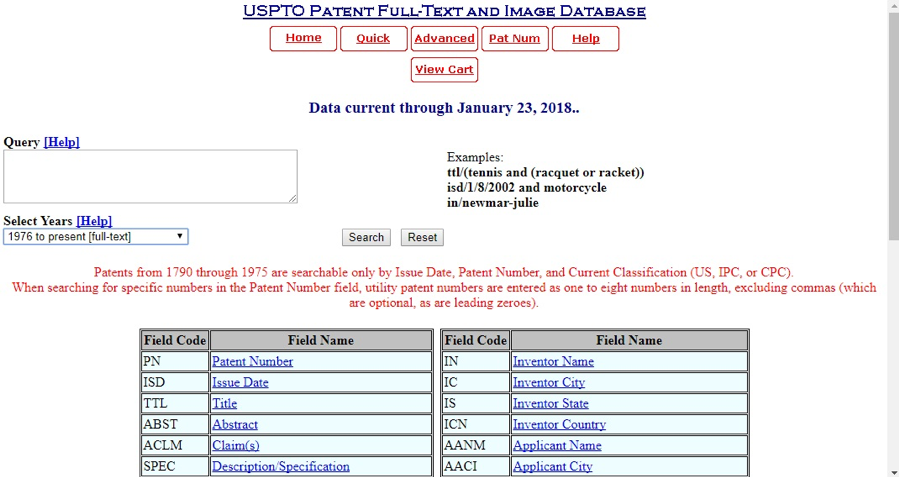
Figure 2: US Patent Office patent search system (advanced search interface)
To test the priming effect, after users are exposed to the prime (either the easy or the difficult prime), a target will then be presented to them. In our case, the target is a third patent search system, which is the FreePatentOnline search system. As shown in Figure 3, its interface design is similar to that of the advanced function of the Google patent search system, but consisting of more fields for users to search across.
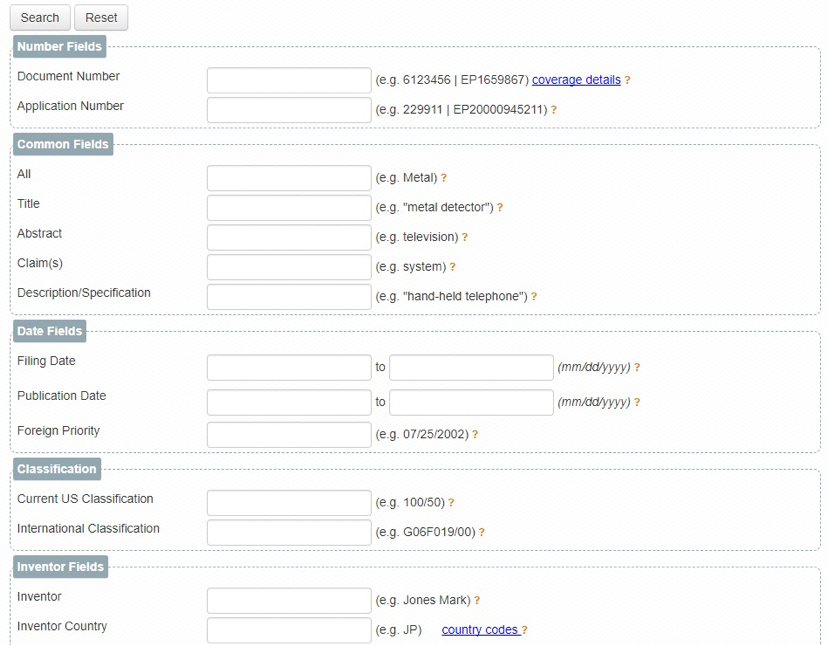
Figure 3: FreePatentOnline (quick search interface)
Our experiment includes three conditions. The first condition (C1) includes the use of the easy prime. Users in this condition will need to use the Google advanced patent search system first, and then use the FreePatentOnline system (which is the target), to perform several patent search tasks. The second condition (C2) includes the use of the difficult prime. In this condition, users will use the Patent Office advanced patent search system first, and then the FreePatentOnline system, to conduct several patent searches. A control condition (C3) is designed without any primes. Users in C3 will go ahead to use the target (i.e., the FreePatentOnline system) directly.
Participants and procedure
Subjects of this study were junior and senior undergraduate students from a public university in the United States. As an incentive for their voluntary participation, a small amount of extra credit was given toward one of their classes. The Institutional Review Board application related to this research was submitted and got approved before conducting the experiment. Each participant had read and signed the consent form before taking the study, and they had the right to leave the study at any time if they felt uncomfortable. To avoid any potential biases, we did not tell participants the specific research purposes of this research (which is to assess the priming effect). Instead, we briefly informed them that they would be asked to use one or two online patent search systems to complete some search tasks and then evaluate the system(s) based on their usage experience.
The experiment was conducted in a computer lab. Multiple sessions were offered at different time slots on different days. An online registration system was used for the user to choose and register in the session that best fit his/her schedule. Each session ran for about one hour, and all participants were able to complete the study within the time slot. We did not interrupt or stop any participants. All of them worked on the study at their own pace, and they were free to leave the laboratoryonce they were done.
At the beginning of each session, a researcher first distributed the consent forms to the participants. After reading the form, if an individual decided to participate, they would need to sign the form. Otherwise, they were free to leave. After that, the researcher spent five to ten minutes to introduce all three patent search systems to the participants and showed them how to use the search functions briefly. Then, participants were sent randomly into the three conditions. Based on which condition a participant was sent into, a package was distributed to them, which provided the URL of the patent system to be used and the tasks to be performed. The last part of the package contained the questionnaire for the participant to fill out. For participants in conditions C1 and C2, they first got the package related to the Google patent search system and Patent Office search system, respectively. Upon completing and returning the first package, a second one about the FreePatentOnline search system was given to them. Participants in C3 completed one package that was about the FreePatentOnline search system. To make sure the search tasks would not be the cause of any cross-condition differences obtained in this study, the same tasks were used in all packages. Also, the same questionnaire items (except for the name of the system) were used. Three search tasks were included in each package. An example is,
Please find a patent that has both keywords robotic and surgery in its title and was issued (i.e., published) by the assignee (i.e., the company or individual that the patent was assigned to at time of issue) of Intuitive Surgical Operations, Inc. during year 2011 (i.e., from 01/01/2011 to 12/31/2011). Please write down the patent number, patent title, and the issue/publication date of the patent you identified. (Please note that the search function may return more than one patent and you can choose any one of them as your answer.)
In total, 140 subjects participated and completed the study, with 50 in C2, 41 in C1, and 49 in C3, respectively. Across all participants, two of them reported to have used Google patent search before, but very occasionally and not its advanced search function. None of them had either used or heard of either the Patent Office or FreePatentOnline search systems before taking part in the study.
Measures
To assess user perceptions of each patent search system, we included in the questionnaire a set of measurement items related to some widely adopted constructs in the information systems literature, including task-technology fit (Goodhue and Thompson, 1995), performance expectancy, effort expectancy, facilitating conditions, behavioural intention to use the system, and user satisfaction (Venkatesh et al., 2003).
Based on the original literature and adapted to our context, we define the measures (theoretical constructs) used in this study as follows.
- Task-technology fit is defined as the level of fit (or match) between an online patent search system and the patent search tasks that the user needs to perform.
- Performance expectancy is defined as the degree to which the user perceives that using an online patent search system will help increase their performance in searching for patents and related information.
- Effort expectancy is defined as the degree of ease associated with the use of an online patent search system
- Facilitating conditions is defined as the user’s belief on the existence of organizational and technical support of using an online patent search system. More specifically, it is about the extent to which users believe there is enough support, as well as clear and helpful instructions and other related resources, from the website to support their use of an online patent search system.
- Behavioural intention is defined as the user’s willingness to use an online patent search system to perform patent search tasks in the future, when they need to do it.
- Satisfaction is used to measure how satifised the user is to use an online patent search system to complete related patent search tasks.
In addition, as an important indicator in examining the amount of burden imposed on a user’s mental system when performing certain type of tasks, frustration level (Hart and Staveland, 1988) was also included in this study.
Specific measurement items on performance expectancy, effort expectancy, facilitating conditions, and behavioural intention to use the system were adopted from (Venkatesh et al., 2003). Items on satisfaction were adopted from (Bhattacherjee, 2001). We developed our own items on task-technology fit according to the general functionality of patent search systems. Items on frustration level were created based on its definition from (Hart and Staveland, 1988). The seven-point Likert scale was used for all measurement items, with ‘1’ being strongly disagree and ‘7’ being strongly agree. In our data analysis, we aggregated the measurement items by using the averaged values across the items.
Data analysis and results
To answer our research questions and assess the priming effect in the context of patent search systems, we conducted the data analysis in two steps. We first validated the setup of the two primes, the easy and difficult ones. Then, we performed detailed comparisons across conditions.
Validating the ‘easy’ and ‘difficult’ primes
As mentioned in the above section, the easy prime used in this study is the advanced search function of the Google patent search system, and the difficult prime is the advanced search function of the Patent Office search system. To valid this setup, we compared users’ task performances in terms of accuracy and efficiency. Accuracy was measured as the number of correctly answered parts in the search tasks divided by the total number of parts in search tasks; efficiency was measured as the amount of time a subject took to complete a search task. Here, the measure of accuracy is different than the measure of performance expectancy. The former is a percentage (with its possible range being 0-100%) which indicates users’ actual performance results on specific tasks that they have worked on, while the latter is based on survey questionnaire (using the seven-point Likert scale) which reflects how well users perceive using a given system will help increase their performance in searching for patents in general.
For each participant, we recorded the start time and end time that they used to perform each search task. The between-subject t-tests were conducted. For accuracy, the mean values for the Google and Patent Office systems were 88.73% and 89.33%, respectively, with no statistically significant differences. For efficiency, the mean values for Google and Patent Office systems were 3.33 and 6.70 minutes, respectively, and it took the participants significantly more time in using the Patent Office search system to perform a task compared with using the Google patent search system (p <0.0001).
These findings showed that both systems could assist users’ patent search tasks relatively effectively, and at a similar level. This indicated the comparability between them in terms of functionality. In addition, the profound and significant difference in the amount of time users needed to perform the same search tasks between the two systems indicated that significantly more mental effort was needed for a user to use the Patent Office search system. Thus, we believe our setup of using the Google patent search system as the easy prime and the Patent Office search system as the difficult prime is reasonable.
To further validate this, we performed between-subject t-tests on all measures based on ratings from the questionnaire, between the use of the Google patent search system (in C1) and Patent Office search system (in C2). Table 1 shows the descriptive statistics and the t-test results.
Measure | C1: Google mean (std. dev.) | C2: Patent Office mean (std. dev.) | t-stats | p-value |
---|---|---|---|---|
Task-technology fit | 5.69 (1.04) | 3.83 (1.43) | 6.963 | < 0.0001* |
Performance expectancy | 5.77 (0.97) | 3.84 (1.78) | 6.235 | < 0.0001* |
Effort expectancy | 5.81 (1.14) | 3.87 (1.51) | 6.812 | < 0.0001* |
Facilitating conditions | 5.24 (0.86) | 4.51 (1.10) | 3.482 | 0.0004* |
Behavioural intention | 5.39 (1.12) | 3.39 (1.43) | 7.795 | < 0.0001* |
Satisfaction | 5.88 (1.19) | 3.16 (1.95) | 7.346 | < 0.0001* |
Frustration level | 2.88 (1.59) | 4.15 (1.66) | 3.708 | 0.0002* |
Note: * = statistically significant |
As shown in Table 1, the ratings on the Google patent search system were all (except for the frustration level) above the mid-point of the 1-7 scale (i.e., 4). However, ratings on the Patent Office patent search system were generally below the mid-point of 4 (except for facilitating conditions and frustration level). As to frustration level, a high value actually means a negative perception. The t-test results showed that participants rated the Google patent search system significantly higher than the Patent Office patent search system on all measures, including task-technology fit, performance expectancy, effort expectancy, facilitating conditions, behavioural intention, and satisfaction. As to frustration level, the rating was significantly lower on the Google patent search system than on the Patent Office patent search system. These results, once again, confirmed users’ perception differences of the two systems, thus indicating the appropriateness of using them as the two primes for this study.
Examining the priming effect
To examine the priming effect, we conducted between-subject t-tests. We chose to use the t-test because of its popularity in between and among group comparisons in human-computer interaction studies using survey data. Since the comparisons in our study were across three conditions, we performed the Bonferroni adjusted t-tests which is a technique that has been used in previous priming literature on comparisons across multiple (more than two) groups experiencing different primes (Li et al., 2015).
Measure | Descriptive statistics | Comparisons | ||||
---|---|---|---|---|---|---|
C1: FPO mean (std. dev.) | C2: FPO mean (std. dev.) | C3: FPO mean (std. dev.) | C1 vs. C2 t-stat (p-value) | C1 vs. C3 t-stat (p-value) | C2 vs. C3 t-stat (p-value) | |
Task-technology fit | 3.92 (1.49) | 5.43 (1.42) | 5.37 (0.99) | 4.926 (< 0.0001)* | 5.502 (< 0.0001)* | 0.241 (0.810) |
Performance expectancy | 4.11 (1.57) | 5.65 (1.54) | 5.59 (0.94) | 4.704 (< 0.0001)* | 5.497 (< 0.0001)* | 0.266 (0.791) |
Effort expectancy | 4.15 (1.58) | 5.75 (1.53) | 5.81 (0.87) | 4.860 (< 0.0001)* | 6.287 (< 0.0001)* | 0.243 (0.808) |
Facilitating conditions | 4.74 (1.10) | 5.15 (1.04) | 5.43 (0.64) | 1.784 (0.078) | 3.706 (< 0.0001)* | 1.658 (0.100) |
Behavioural intention | 3.98 (1.30) | 5.14 (1.41) | 5.36 (1.09) | 4.015 (< 0.0001)* | 5.465 (< 0.0001)* | 0.875 (0.384) |
Satisfaction | 2.98 (1.74) | 5.54 (1.56) | 5.48 (1.44) | 7.421 (< 0.0001)* | 7.494 (< 0.0001)* | 0.189 (0.850) |
Frustration level | 3.62 (1.54) | 2.63 (1.52) | 2.60 (1.23) | 3.073 (< 0.0001)* | 3.486 (< 0.0001)* | 0.101 (0.920) |
Note: * Statistically significant. The alpha used for Bonferroni adjusted t-tests is 0.017 (which is 0.05 divided by 3) |
As shown in Table 2, significant differences were found on almost all measures (except for facilitating conditions) between conditions C1 and C2, indicating the general existence and significance of the priming effect. Specifically, compared with subjects who experienced the easy prime (C1), those subjects who exposed to the difficult prime (C2) perceived that the target system could provide a significantly better fit to their patent search tasks (p <0.0001), and higher levels of performance (p < 0.0001) and effort expectancies (p < 0.0001). They also were more intended to use the target system (p < 0.0001) and were more satisfied with using it (p < 0.0001). They expressed a much lower level of frustration when using the target system (p < 0.0001). These results may suggest that when primed by a difficult-to-use search system, users may find it mentally easy to learn how to use and adjust to a new system, and thus interpreting their usage experience on the new system in a more positive way, as compared to those who were primed by an easy-to-use system.
As to the control group (C3), significant differences were found between it and C1, on all measures (p<.0001). The results suggested that when not being exposed to any primes, users tended to have more positive attitudes (with higher ratings) on the target system, compared with being primed by an easy-to-use system. However, no significant differences were found between the control group (C3) and the group primed by the difficult-to-use system (C2). Overall, these findings could suggest that an easy prime might lead to a significant amount of influence on how users perceive a subsequent system (the target), and most likely in a negative way; however, a difficult prime does not seem to provide such a strong level of influence. In other words, this may indicate that an easy-to-use system could have a significant priming effect on participants’ perception of the target system (C1 vs. C3 comparison), whereas a difficult-to-use system could not (C2 vs. C3 comparison).
This result might be because that when first exposed to an easy prime, users may feel comfortable and satisfied with it, resulting in less motivation to change to the target system. Thus, when they are asked to switch to the target system, they may be reluctant and treat the second system less valuable. On the contrary, the non-significant comparison result on C2 vs. C3 may suggest that when users are first exposed to a difficult prime, they tend to treat the subsequent system in a more objective manner (with consistent and comparable results to the control group in which users are not exposed to any prime). As to the non-significant priming effect of the difficult-to-use system, it may be because that although the search function of the system is difficult to use (users need to spend significantly more time in using it to conduct search tasks), it is effective enough (with the same level of accuracy as associated with the use of the easy-to-use system) for the users to complete the search tasks. Therefore, users may be satisfied with its overall performance, thus leading to a relatively unbiased perception of the subsequent system. To further validate this possible explanation, future research may compare two types of difficult-to-use systems, with one being effective and the other being ineffective (meaning that users’ task performance accuracy associated with it is significantly lower).
Discussion and future research suggestions
This study makes several contributions. First, we empirically investigated the priming effect in the context of intellectual property search systems, which has not been seen in existing literature. To do it, a laboratory experiment was conducted with three conditions, including: users who were primed by an easy-to-use system (C1), primed by a difficult-to-use system (C2), and without any primes (C3). Detailed comparisons were conducted across the three conditions on user perceptions of a target system on a group of measures (including task-technology fit, performance expectancy, effort expectancy, facilitating conditions, behavioural intention, satisfaction, and frustration level). Significant differences were found between C1 and C2, indicating the existence of the priming effect. We also found out that users primed by the difficult-to-use system rated the target system significantly higher (reversely on frustration level) compared with those primed by the easy-to-use system. Comparisons to the control group showed that an easy prime could significantly influence user perceptions on the target system and in a negative way. Overall, this study contributes to the literature by validating the existence of the priming effect in the context of intellectual property search systems and identifying some details on how the priming systems could influence user perceptions on the target system.
The second contribution of this study is the way of setting up the primes. Previous research on investigating the priming effect mainly utilized certain visual objects in different types and formats as the primes, such as numbers and symbols (Pinder et al., 2018), words (Pinder et al., 2017), identity information (Heyselaar et al., 2017), short descriptions (Li et al., 2015), images (D’Errico et al., 2019; Ragot et al., 2020; Valdez et al., 2018), and 3-D visual objects (Bhagwatwar et al., 2013). A few of them utilised more complex ones that users needed to actively participate, with the requirement of either physical movement (Hoff et al., 2016) or mental processing (Dennis et al., 2013). Different from them, the primes used in this study are a type of advanced information systems, particularly intellectual property search systems, and users need to actually use them to complete related tasks. Specifically, users were asked to use the priming system first, followed by the target system, in order to investigate whether and how their priming system usage experience could influence their perceptions of the target system. In addition, to better understand how the priming system could influence user attitude, we utilized two primes in this study, which varied significantly at the level of ease of use associated with them, including an easy prime (i.e., a system that was generally believed to be easy to use) and a difficult prime (i.e., a system that was generally believed to be difficult to use). Validation tests were conducted to make sure the two systems used as the primes were appropriate.
There are several limitations of this study that future research could further address. First, since this is one of the first studies examining the priming effect in the context of intellectual property search systems, and particularly using this type of systems as the primes, there is not enough support from existing literature for us to develop specific hypotheses (such as whether priming in this context is clearly a natural phenomenon or surprising cognitive bias). The present study is explorative in nature. Future research could extend the current work to focus on identifying the related psychological explanations and develop specific hypotheses for testing. Another limitation is the unbalanced time spans on system use across the three conditions. In both C1 and C2, participants needed to use two systems, making it likely for them to spend longer time to complete the experiment, compared with those in C3 who used only one system. Although all participants across all three conditions could complete the entire experiment within an hour, and we did not hear any complains about the length of the experiment from any participants, it would be better if the time needed to complete the experiment in all conditions could be equally long.
Future research could consider using a filler task technique in the control condition. In addition, the use of the same tasks in both systems in C1 and C2 might be another limitation. We chose to use the same tasks because we wanted to avoid any potential biases that might be introduced because of the differences in tasks. However, using the same tasks might bring in other types of biases. Future research may consider leveraging very similar but not identical tasks with different system usage. As to the findings about the comparisons across the three conditions, significant differences were found in both C1 vs. C2 and C1 vs. C3 comparisons, but not on C2 vs. C3. These results did suggest the existence of the priming effect in our context of study. But because of the lack of enough literature support, as well as the explorative nature of the current study, it is difficult for us to identify the specific underlying reasons of these findings, especially on the non-significant results. Future research could put more effort in this direction to investigate the specific reasons. In addition, the use of student subjects could be another limitation of this study. Future research could consider recruiting employees from real-world organizations to further test and validate the results found in this study. Future research could also extend this study by investigating the priming effect on other types of systems and including more measures for the assessment.
About the authors
Mandy Yan Dang is Associate Professor and Franke Professor in the W. A. Franke College of Business, Northern Arizona University, 101 E. McConnell Drive, Flagstaff, Arizona, 86011, USA. She received her Ph.D. from the Eller College of Management, University of Arizona. Her research interests are in the implementation and adoption of information technology, human cognition and decision making, human-computer interaction, and information systems education. She can be contacted at mandy.dang@nau.edu
Yulei (Gavin) Zhang is Associate Professor and Franke Professor in the W. A. Franke College of Business, Northern Arizona University, 101 E. McConnell Drive, Flagstaff, Arizona, 86011, USA. He received his Ph.D. from the Eller College of Management, University of Arizona. His research interests are in social computing and social media analytics, web and text mining, and information systems education. He can be contacted at yulei.zhang@nau.edu
Kevin Trainor is Associate Professor and Franke Professor in the W. A. Franke College of Business, Northern Arizona University, 101 E. McConnell Drive, Flagstaff, Arizona, 86011, USA. He received his Ph.D. from Kent State University. His research interests are in innovation and new product development, social media marketing, customer relationship management. He can be contacted at kevin.trainor@nau.edu
References
Note: A link from the title is to an open access document. A link from the DOI is to the publisher's page for the document.
- Adomavicius, G., Bockstedt, J. C., Curley, S. P., & Zhang, J. (2013). Do recommender systems manipulate consumer preferences? A study of anchoring effects. Information Systems Research, 24(4), 956-975.
- Bargh, J. A., Gollwitzer, P. M., Lee-Chai, A., Barndollar, K., & Trötschel, R. (2001). The automated will: nonconscious activation and pursuit of behavioral goals. Journal of Personality and Social Psychology, 81(6), 1014-1027.
- Bhagwatwar, A., Massey, A., & Dennis, A. R. (2013). Creative virtual environments: effect of supraliminal priming on team brainstorming. 2013 46th Hawaii International Conference on System Sciences, Wailea, Maui, HI, USA.
- Bhattacherjee, A. (2001). Understanding information systems continuance: an expectation-confirmation model. MIS Quarterly, 25(3), 351-370. http://dx.doi.org/10.2307/3250921
- Bodoff, D., & Vaknin, E. (2016). Priming effects and strategic influences in social tagging. Human–Computer Interaction, 31(2), 133-171. https://doi.org/10.1080/07370024.2015.1080609
- Chalfoun, P., & Frasson, C. (2008). Subliminal priming enhances learning in a distant virtual 3D intelligent tutoring system. IEEE Multidisciplinary Engineering Education Magazine, 3(4), 125-130.
- Chartrand, T. L., van Baaren, R. B., & Bargh, J. A. (2006). Linking automatic evaluation to mood and information processing style: consequences for experienced affect, impression formation, & stereotyping. Journal of Experimental Psychology: General, 135(1), 70-77. https://doi.org/10.1037/0096-3445.135.1.70
- D’Errico, F., Paciello, M., Fida, R., & Tramontano, C. (2019). Effect of affective priming on prosocial orientation through mobile application: differences between digital immigrants and natives. Acta Polytechnica Hungarica, 16(2), 109-128. https://doi.org/10.12700/APH.16.2.2019.2.7
- D’Urso, D., Mauro, C. D., Chiacchio, F., & Compagno, L. (2017). A behavioural analysis of the newsvendor game: anchoring and adjustment with and without demand information. Computers and Industrial Engineering, 111(C), 552-562. https://doi.org/10.1016/j.cie.2017.03.009
- DeLone, W. H., & McLean, E. R. (2003). The Delone and McLean model of information systems success: a ten-year update. Journal of Management Information Systems, 19(4), 9-30. https://doi.org/10.1080/07421222.2003.11045748
- DeLone, W. H., & McLean, E. R. (1992). Information systems success: the quest for the dependent variable. Information Systems Research, 3(1), 60-95. https://doi.org/10.1287/isre.3.1.60
- Dennis, A. R., Bhagwatwar, A., & Minas, R. K. (2013). Play for performance: using computer games to improve motivation and test-taking performance. Journal of Information Systems Education, 24(3), 223-231.
- Fu, W.-T., Kannampallil, T., Kang, R., & He, J. (2010). Semantic imitation in social tagging. ACM Transactions on Computer-Human Interaction, 17(3), 1-37. https://doi.org/10.1145/1806923.1806926
- Goodhue, D. L., & Thompson, R. L. (1995). Task-technology fit and individual performance. MIS Quarterly, 19(2), 213-236. https://doi.org/10.2307/249689
- Hart, S. G., & Staveland, L. E. (1988). Development of NASA-TLX (task load index): results of empirical and theoretical research. In P. A. Hancock and N. Meshkati (Eds.), Human mental workload (pp. 139-183). Elsevier Science Publishers.
- Heijden, H. v. d. (2013). Evaluating dual performance measures on information dashboards: effects of anchoring and presentation format. Journal of Information Systems, 27(2), 21-34. https://doi.org/10.2308/isys-50556
- Heyselaar, E., Hagoort, P., & Segaert, K. (2017). In dialogue with an avatar, language behavior is identical to dialogue with a human partner. Behavior Research Methods, 49(1), 46-60. https://doi.org/10.3758/s13428-015-0688-7
- Hoff, L., Hornecker, E., & Bertel, S. (2016). Modifying gesture elicitation: do kinaesthetic priming and increased production reduce legacy Bias? In Proceedings of the TEI '16: Tenth International Conference on Tangible, Embedded, & Embodied Interaction, Eindhoven, the Netherlands, February, 2016. (pp. 86-91). ACM. https://doi.org/10.1145/2839462.2839472
- Li, Y., Oladimeji, P., & Thimbleby, H. (2015). Exploring the effect of pre-operational priming intervention on number entry errors. In Proceedings of the 33rd Annual ACM Conference on Human Factors in Computing Systems; Seoul, Republic of Korea; April 2015. (pp. 1335-1355). ACM. https://doi.org/10.1145/2702123.2702477
- Minas, R. K., & Dennis, A. R. (2019). Visual background music: creativity support systems with priming. Journal of Management Information Systems, 36(1), 230-258. https://doi.org/10.1080/07421222.2018.1550559
- Murphy, S. T., & Zajonc, R. B. (1993). Affect, cognition, & awareness: affective priming with optimal and suboptimal stimulus exposures. Journal of Personality and Social Psychology, 64(5), 723-739. https://doi.org/10.1037/0022-3514.64.5.723
- Pinder, C., Vermeulen, J., & Cowan, B. R. (2018). Subliminal semantic number processing on smartphones. In MobileHCI '18: Proceedings of the 20th International Conference on Human-Computer Interaction with Mobile Devices and Services (Article 24). ACM. https://doi.org/10.1145/3229434.3229451
- Pinder, C., Vermeulen, J., Cowan, B. R., Beale, R., & Hendley, R. J. (2017). Exploring the feasibility of subliminal priming on smartphones. In MobileHCI 2017: the 19th International Conference on Human-Computer Interaction with Mobile Devices and Services, Vienna, Austria. (Article 21). ACM. https://doi.org/10.1145/3098279.3098531
- Ragot, M., Martin, N., & Cojean, S. (2020). AI-generated vs. human artworks. A perception bias towards artificial intelligence? In CHI '20: CHI Conference on Human Factors in Computing Systems Honolulu. HI. USA April, 2020 (10 p.) Association for Computing Machinery. https://doi.org/10.1145/3334480.3382892
- Segal, S. J., & Cofer, C. N. (1960). The effects of recency and recall on word association. [Abstract in Program of the sixty-eighth annual convention of the American Psychological Association] American Psychologist, 15(7), 451.
- Tsohou, A., Karyda, M., & Kokolakis, S. (2015). Analyzing the role of cognitive and cultural biases in the internalization of information security policies: recommendations for information security awareness programs. Computers and Security, 52, 128-141. https://doi.org/10.1016/j.cose.2015.04.006
- Tversky, A., & Kahneman, D. (1992). Advances in prospect theory: cumulative representation of uncertainty. Journal of Risk and Uncertainty, 5(4), 297-323. https://doi.org/10.1007/BF00122574
- Tversky, A., & Kahneman, D. (1974). Judgment under uncertainty: heuristics and biases. Science, 185(4157), 1124-1131. https://doi.org/10.1126/science.185.4157.1124
- Valdez, A. e. C., Ziefle, M., & Sedlmair, M. (2018). Priming and anchoring effects in visualization. IEEE Transactions on Visualization and Computer Graphics, 24(1), 584-594. https://doi.org/10.1109/TVCG.2017.2744138
- Venkatesh, V., Morris, M. G., Davis, G. B., & Davis, F. D. (2003). User acceptance of information technology: toward a unified view. MIS Quarterly, 27(3), 425-478. https://doi.org/10.2307/30036540
- Wu, C.-S., Cheng, F.-F., & Yen, D. C. (2012). The role of Internet buyer’s product familiarity and confidence in anchoring effect. Behaviour and Information Technology, 31(9), 829-838. https://doi.org/10.1080/0144929X.2010.510210
- Wu, I.-C., & Niu, Y.-F. (2015). Effects of anchoring process under preference stabilities for interactive movie recommendations. Journal of the Association for Information Science and Technology, 66(8), 1673-1695. https://doi.org/10.1002/asi.23280