Effects of objective and subjective competence on the reliability of crowdsourced relevance judgments
Parnia Samimi, Sri Devi Ravana, William Webber, and Yun Sing Koh
Introduction. Despite the popularity of crowdsourcing, the reliability of crowdsourced output has been questioned since crowdsourced workers display varied degrees of attention, ability and accuracy. It is important, therefore, to understand the factors that affect the reliability of crowdsourcing. In the context of producing relevance judgments, crowdsourcing has been recently proposed as an alternative approach to traditional methods of information retrieval evaluation, which are mostly expensive and scale poorly.
Aim. The purpose of this study is to measure various cognitive characteristics of crowdsourced workers, and explore the effect that these characteristics have upon judgment reliability, as measured against a human gold standard.
Method. The authors examined whether workers with high verbal comprehension skill could outperform workers with low verbal comprehension skill in terms of judgment reliability in crowdsourcing.
Results. A significant correlation was found between judgment reliability and measured verbal comprehension skill, as well as with self-reported difficulty of judgment and confidence in the task. Surprisingly, however, there is no correlation between level of self-reported topic knowledge and reliability.
Conclusions. Our findings show that verbal comprehension skill influences the accuracy of the relevance judgments created by the crowdsourced workers.
Introduction
The term crowdsourcing was coined by Howe (2006) in a Wired Magazine article, and based on Web 2.0 technology. Crowdsourcing is defined as outsourcing tasks, which were formerly accomplished inside a company or institution by employees, to a huge, heterogeneous mass of potential workers in the form of an open call through the Internet. Crowdsourced workers (henceforth workers) are hired through online Web services such as Amazon Mechanical Turk, and work online to perform repetitive cognitive piece-work (known as tasks) at low cost, with many workers potentially working in parallel to quickly complete a task. Tasks are referred to as human intelligence tasks. Crowdsourcing can be applied widely in various fields of computer science and other disciplines to test and evaluate studies (Zhao and Zhu, 2012). Crowdsourcing platforms were also suggested for the purposes of collecting survey data for behavioural research as a viable choice (Behrend, Sharek, Meade and Wiebe, 2011). Mason et al. ( 2012) have stated three advantages of crowdsourcing platforms: (i) allowing a large number of workers to take part in experiments with low payment; (ii) workers are from diverse countries, cultures and backgrounds, have different ages and speak different languages; and (iii) low cost at which the research can be carried out.
Recently, the use of crowdsourcing in information retrieval evaluation has attracted interest (Alonso and Mizzaro, 2012). Test collections are frequently used to evaluate information retrieval systems, in both laboratory experimentation and product development. This is sometimes referred to as the 'Cranfield paradigm', in reference to the original research on laboratory retrieval evaluation conducted by Cyril Cleverdon at Cranfield University (Cleverdon, 1967). The Text REtrieval Conference (TREC) was established in 1992 in order to support information retrieval research by providing the infrastructure for large scale evaluation of retrieval methodologies. A test collection consists of a set of documents or corpus, a set of topics or search requests and relevance judgments as to which documents are relevant to which topics. Relevance judgments are made by human assessment (unless pseudo-judgments are inferred from user click behaviour), and hiring expert assessors to perform these judgments is expensive and time-consuming. As the size and diversity of test collections have grown, this expense has become increasingly burdensome. Crowdsourcing has been proposed as an economically viable alternative to collect relevance judgments in order to overcome this issue, mainly due to its low cost and fast turnaround (Alonso, Rose and Stewart, 2008). However, the quality and reliability of crowdsourcing has been questioned for several reasons, such as:
- Workers may have inadequate expertise for the task at hand (Quinn and Bederson, 2011).
- Demographic and personality traits of workers may be different and unrecognized, which can affect the quality of crowdsourced relevance judgments (Kazai, Kamps and Milic-Frayling, 2012).
- The quality of the final relevance judgments is highly subjective to the workers' level of interest, incentive and attention for a given task (Kazai, Kamps and Milic-Frayling, 2011).
A range of quality assurance and control techniques were used to reduce the noise (poor quality outputs) that were produced during or after the completion of a given task. However, little is known about the workers themselves and the role of individual differences in the reliability of crowdsourced relevance judgments. Individual differences in cognitive performance are defined as cognitive abilities. These abilities are mainly brain-based skills, concerning learning, remembering, problem-solving and attention and mindfulness (Ekstrom, French, Harman and Dermen, 1976). The objective of this study was to assess the human factors that influence the quality of crowdsourcing output and more specifically crowdsourced relevance judgments. We investigated the linguistic and cognitive capacities of workers through tests and questionnaires. The cognitive ability that we tested was verbal comprehension skill; that is, the ability to understand the English language, believed to be one of the key factors influencing informational retrieval behaviour (Allen, 1992), and potentially important also in judging the relevance of a document. We also tested the relationship between the reliability of the relevance judgments on the one hand, and self-reported difficulty of the task, confidence of the worker, and worker’s knowledge of the topic on the other. We hypothesized that more reliable judgments are produced by workers who are declaring the given tasks to be easy, showing higher confidence in their judgment and reporting themselves knowledgeable about the topics. The reliability of the workers was compared to that of an expert assessor, both directly as the overlap between relevance assessments, and indirectly by comparing the system effectiveness evaluation arrived at from expert and from worker assessors. Specifically, this study addressed the following research questions:
R1: Does the verbal comprehension skill of a worker have an effect on the quality of crowdsourcing output, specifically, on their relevance judgments?
R2: How do a worker’s (i) topic knowledge, (ii) perceived difficulty of the task, and (iii) confidence in correctness, relate to the accuracy of the worker’s relevance judgments?
We begin by surveying factors influencing the reliability of crowdsourcing output. Recent studies on the use of crowdsourcing to create relevance judgments for the evaluation of information retrieval systems are reviewed, followed by an overview of the role of cognitive abilities in the information retrieval process. Next, we explain our research methodology, design and dataset. Finally, we discuss the results of the experiment, and we draw our conclusions.
Background
Factors that affect the reliability of crowdsourcing output
Crowdsourcing suffers from low quality output due to various types of workers’ behaviour (Zhu and Carterette, 2010). In order to reduce the impact from malicious workers and improve the quality of crowdsourced output, it is useful to categorize workers according to their accuracy when performing the outsourced tasks, e.g., elite workers (who accomplish tasks with accuracy of 100%), competent workers, incompetent workers and so forth (Gadiraju, Kawase, Dietze and Demartini, 2015).
There are different factors that affect the reliability of crowdsourcing experiments such as experimental design, human features, and monetary factors. Experimental design is the most critical part of the crowdsourcing process (Alonso, 2012). Beyond the workers’ levels of attention, diversity of cultures and variations in preferences and skills, the presentation and properties of human intelligence tasks are the key factors for the quality of crowdsourcing. Indeed, the quality of the user interface, the instructions and the design of the crowdsourcing process have a direct relationship with the quality of the task performed by a worker. In the experimental design, the first information that needs to be presented to the workers is the definition of the given task. Task description is part of task preparation and is an important topic in implementing a crowdsourcing experiment. Task description along with clear instructions are crucial to getting a quick result. Ideally, all of the workers should have a common understanding about a chosen task, and the task must be understandable in the language of the workers (Alonso, 2012). Task description should be prepared according to the variation in the general characteristics of workers such as their language and/or the level of their expertise in the field (Allahbakhsh et al., 2013).
Human features of a worker define a worker profile, which consists of one’s reputation and expertise (credentials and experience) in accomplishment of tasks. A worker profile has a significant influence on the quality of results. Requesters may provide feedback about the quality of the particular work to a worker. Feedback scores are used in the system to determine the reputation of a worker (De Alfaro, Kulshreshtha, Pye and Adler, 2011). Reciprocally, requesters need to enhance their reputations in order to increase the probability that their human intelligence tasks will be accepted by workers (Paolacci, Chandler and Ipeirotis, 2010). Information such as language, location and academic degree builds credentials, but the knowledge that a worker achieves through the crowdsourcing system is referred to as experience (Allahbakhsh et al., 2013).
In crowdsourcing, monetary factors such as payment affect the accuracy of results. Workers satisfied by the payment more accurately accomplish tasks than those who are left unsatisfied (Kazai, Kamps and Milic-Frayling, 2013). Monetary and/or non-monetary reasons can be the motivation for the workers of crowdsourcing platforms (Hammon and Hippner, 2012). A study conducted by Ross et al. showed that financial gain is a main incentive for workers in crowdsourcing (Ross, Irani, Silberman, Zaldivar and Tomlinson, 2010). Ipeirotis (2010) reported that Amazon Mechanical Turk was the main income of 27% of Indian and 12% of US workers. Kazai (2011) reported that increasing the payment enhances the quality of work whilst there is some evidence that higher payment has an effect only on completion time rather than on quality of results (Potthast, Stein, Barrón-Cedeño and Rosso, 2010) and that a high level of payment motivates a worker to perform a task faster but not necessarily with better quality. Reasonable payment appears to be a more thoughtful and conservative solution as high pay tasks attract spammers as well as legitimate workers (Grady and Lease, 2010).
Crowdsourcing in information retrieval evaluation
In 2011, Kazai et al. investigated the relationship between workers’ behavioural patterns, the accuracy of their judgments and their personality profiles (based on the Big Five personality traits (John, Naumann and Soto, 2008)). They found a strong correlation between the accuracy of judgments and the openness trait. Five types of workers (spammer, sloppy, incompetent, competent, and diligent) were identified, based on their behavioural patterns (Zhu and Carterette, 2010). In 2012, Kazai et al. studied the relationship between demographics, the personality of workers and label accuracy. They used two different task designs, namely full design, which has a strict quality control, and simple design, with less quality control. The results showed that the demographics and personality of the workers were strongly related to label accuracy. Among demographic factors, location had the strongest relationship with label accuracy, with the lowest accuracy from Asian workers and the highest accuracy from American and European workers. Asian workers were more likely to undertake the simple design, while American and European workers were more likely to undertake the full design, although the difference may have been an artifact of the pre-filtering process that happened in full design (in which workers without a sufficient reputation score were filtered out (Kazai et al., 2012)). In another study, the effect of the level of pay, effort to complete tasks and qualifications needed to undertake tasks on the quality of the labels was investigated, and correlated with various human factors. The study found that higher payment leads to better output quality among qualified workers, but also attracts workers that are less ethical. Higher effort tasks lead to labels that are more inaccurate, while enticing better performing workers. Limiting access to tasks to reliable workers increases the quality of the results. Earning money is the main motivation for workers to do the tasks (Kazai et al., 2013).
Alonso et al. (2008) ran five preliminary experiments with different alternatives, such as qualification tests and changing interface, through Amazon Mechanical Turk using TREC data and measured the agreement between crowdsourced workers and TREC assessors. The findings showed that the judgments of crowdsourced workers were comparable to the TREC assessors. In some cases, the workers detected TREC assessors’ errors. In Alonso and Mizzaro (2012), the use of crowdsourcing for creating relevance judgments was validated through a comprehensive experiment. The experimental results show that crowdsourcing is a low cost, reliable and quick solution, and an alternative to creating relevance judgments by expert assessors, but it is not a replacement for current methods because there are still several gaps and questions that are left for future research. For example, the scalability of this approach has not been investigated yet. Blanco et al. (2011) investigated the repeatability of crowdsourced evaluation. The results show that crowdsourcing experiments can be repeated over time in a reliable manner. Although there were differences between human expert judgments and crowdsourced judgments, the system ranking was the same. Clough et al. (2012) compared the reliability of crowdsourced and expert judgments when used in information retrieval evaluation. They evaluated two search engines on informational and navigational queries, using crowdsourced and expert judgments. The study found the crowdsourced judgments comparable to expert judgments, with a strong positive correlation between search effectiveness measured by each class of judgments. In terms of correlation between expert judgment and crowdsourced workers, the disagreements were more common on documents returned by the better performing system and on documents returned for informational queries.
Cognitive abilities in the information retrieval process
This study was motivated by the theory of the information retrieval process, which suggests cognitive abilities most likely influence information retrieval effectiveness. It explored the effect of cognitive abilities of workers on reliability of relevance judgments. We hypothesized that the same relationship would pertain to relevance assessment, as understanding the content of documents and topics in the relevance judgment task requires reading, understanding text and evaluating its relevancy. We thought it possible that people with higher level of cognitive ability would be more likely to create more accurate relevance judgments. This idea is derived from Allen (1992) who demonstrated that cognitive abilities influence the information retrieval processes. Recently, Brennan et al. claimed that information search is principally about cognitive activities (Brennan, Kelly and Arguello, 2014). Therefore, understanding the effect of cognitive abilities on search behaviour is an important research topic. One of the popular instruments to assess cognitive abilities is the kit of the Factor-Referenced Cognitive Tests, produced by the US-based Educational Testing Service (Ekstrom et al., 1976). This kit contains seventy-two tests to measure twenty-three different cognitive factors. The kit is still widely used in various areas of research (Geary, Hoard, Nugent and Bailey, 2013; Beaty, Silvia, Nusbaum, Jauk and Benedek, 2014; Salthouse, 2014).
In the area of information retrieval, the effect of perceptual speed, logical reasoning, spatial scanning and verbal comprehension skills on how well academic librarians are suitable for their jobs and their performance in searching were investigated by Allen and Allen (1993). Furthermore, the cognitive abilities of librarians and students were compared. The results of this study showed that students had higher level of perceptual speed and librarians had higher level of logical reasoning and verbal comprehension skills. Cognitive abilities have an effect on information retrieval performance, and therefore, different approaches to information retrieval may be suitable for librarians and students. In a more recent study (Brennan et al., 2014), the effects of cognitive abilities on search behaviour were investigated during search tasks, measuring visualization ability, perceptual speed and memory. The findings of this study showed that among these three cognitive abilities, both perceptual speed and visualization ability had a higher positive correlation with search behaviour than memory. In a study about search effectiveness of users applying a TREC test collection, the effect of characteristics of users (for instance, whether they have some prior search experience) and their levels of cognitive ability was assessed (Al Maskari and Sanderson, 2011). Those users with higher perceptual skills and prior search experience demonstrated a better search effectiveness when compared with users with less experience and lower perceptual abilities.
Need for cognition defines an individual difference measure of 'the extent to which a person enjoys engaging in effortful cognitive activity' (Scholer, Kelly, Wu, Lee and Webber, 2013, p. 624). A study of the impact of need for cognition on relevance assessments showed that the participants with high need for cognition had a significantly higher level of agreement with expert assessors in terms of relevance assessment than low need for cognition participants. Indeed, we consequently expected that crowdsourced workers with high cognitive abilities would be likely to create more reliable relevance judgments. We predicted that information retrieval practitioners, in choosing individuals to create relevance judgments, would be likely to select workers with higher level of cognitive abilities. To the authors’ knowledge, however, no studies have investigated the cognitive abilities of crowdsourced workers and their effect on the workers’ reliability in judging the relevance of documents.
Method
Experiment data
Eight topics were chosen from the TREC-9 Web Track (http://trec.nist.gov/data/t9.Web.html), and twenty documents were randomly obtained for each topic from the WT10g document collection (http://ir.dcs.gla.ac.uk/test_collections/wt10g.html). All documents and topics were in English. According to the original TREC assessors, of the twenty chosen documents, ten were relevant and ten were non-relevant. However, in creating a relevance judgment set, based on the TREC setting, many non-relevant outcomes would be presented to the TREC assessors compared to the number of relevant ones. The reason for selecting an equal number of relevant and non-relevant documents in this study was that a long sequence of irrelevant documents might cause an assessor to lower their threshold of relevance, or instead to lose attention and miss relevant documents (Scholer et al., 2013). For each of the 160 topic, document pairs, ten binary judgments were obtained through crowdsourcing (each one from a different worker), and a total of 1600 judgments were made by workers. The number of workers who performed the tasks was 154. In this study, the gold standard dataset was the relevance judgments set created by the official TREC assessors to which relevance judgments made by the crowdsourced workers were compared.
Task design
In this study, there were forty tasks designed in (Crowdflower), a popular crowdsourcing platform. Each task was to be completed by 10 workers and required two steps to be completed. In the first step, each task had four topics, and each topic had a document to be assessed for the relevance judgment against a given topic. Upon completing each judgment, the workers were required to complete a questionnaire. The questionnaire consisted of the following three items, to be answered on a 4-point scale:
Question 1) Rate your knowledge on the topic: (Minimal 1 2 3 4 Extensive).
Question 2) How difficult was this evaluation: (Easy 1 2 3 4 Difficult).
Question 3) How confident were you in your evaluation: (Not confident 1 2 3 4 Very confident).
The second step was to examine the workers’ verbal comprehension skills. In this step, the workers were asked to complete a vocabulary test of ten out of thirty-six questions. These questions were randomly sampled from the suite of evaluation exercises known as the Kit of Factor-Referenced Cognitive Test ( Ekstrom et al., 1976). The workers were required to choose one of four words that had a similar meaning to the given word. The verbal comprehension score was then calculated on the basis of the overall vocabulary task.
In this study, the relevance judgment task setting was different from the classic judgment method by TREC assessors in which a TREC assessor judges all documents from the same topic. The reason why we had four topics and documents in each task was the use of the crowdsourcing platform in our experiment. Crowdsourcing tasks are commonly short. For a long and complex task, the suggestion is to split the task into a few simpler tasks, because they may attract more workers to complete the experiment (Alonso, 2012). In addition, because we were using crowdsourcing, we had to limit the number of questions for the vocabulary test to ten (out of thirty-six) questions. We also assessed whether the ten questions (10-question test) could provide acceptable outcomes to evaluate the verbal comprehension skill of workers. The later was assessed by comparing the outcomes with that of the full thirty-six question version of the test. Two verbal comprehension scores were calculated for each worker; one for the thirty-six question test and another one for the ten question test. According to the median split of the comprehension score, the workers were categorized into two groups, namely low verbal comprehension score and high verbal comprehension score, based on two scores. The kappa (κ) for goodness of fit was then calculated to find out whether there was an agreement for the grouping (Pallant, 2001) between the ten question test and the thirty-six question test. The kappa measure of agreement was used to evaluate the consistency of the two tests, showing a strong agreement (κ = 0.70) between the two tests. Therefore, in this study, the use of the ten question test could work around the limitations posed by crowdsourcing, and could provide a statistically meaningful tool to assess workers’ verbal comprehension skill.
Filtering spam
Crowdsourcing is subject to untrustworthy workers, who complete tasks fast but carelessly (with least effort), just to earn the money. Filtering such workers is a common quality control procedure in crowdsourcing ( Kazai et al., 2013). As the vocabulary test is a multiple-choice test with four choices per question and ten questions, a worker selecting at random has an expected score of 2.5. Put another way, a worker selecting at random has less than a one in four chance of achieving a score of 4 or higher. In our experiment, workers completed the vocabulary test for each task they accepted. The filtering method was based on the score of the vocabulary test achieved by a worker for each task. Those tasks in which workers achieved verbal comprehension scores of 3 or less were considered unreliable: either they were spammers or workers with no English language ability. The intention behind considering tasks rather than workers in the filtering process was that a worker might accomplish different tasks with various levels of accuracy. In other words, a worker might accomplish one task precisely and another task precipitately. Applying the filtering technique in this study, there were eighty-one unreliable tasks out of 400 tasks. Therefore, of the 1600 judgments submitted, we could only consider 1276 judgments as reliable, constituting 147 workers (out of 154).
Analysis methods
Reliability of relevance judgments is measured as the agreement between the worker and the TREC expert assessor (gold standard). However, relevance judgments are subjective and can vary among assessors (Kazai et al., 2013). For instance, an agreement between two TREC assessors was reported 70% to 80% on average (Voorhees and Harman, 2005). In this study, the agreement in terms of relevance judgments was evaluated based on two different methods: (i) percentage agreement, which is the simplest and easiest measure calculated by dividing the number of times for each rating (e.g. 1, 2, ... 5) assigned by each assessor, by the total number of the ratings, and (ii) Cohen's kappa, which is an adjusted version of accuracy measuring the probability of chance agreement. Qualitative interpretation of Cohen’s kappa is established through a five-level scale proposed by Landis and Koch (1977). The five-level scale consists of slight agreement (0.01–0.20), fair agreement (0.21–0.40), moderate agreement (0.41–0.60), substantial agreement (0.61–0.80) and perfect agreement (0.81–0.99).
Accuracy of the relevance judgments is the proportion of judgments on which the worker and the gold standard agree (i.e. TREC assessors in our study) ( Kazai et al., 2013). Accuracy is ranged from 0 (no agreement) to 1 (complete agreement). Accuracy can be measured over the number of documents included in a single human intelligence task (in our case there were four tasks):

We used Pearson’s correlation coefficient to measure the relationship between two real-valued user factors (for instance, verbal comprehension score and accuracy). A correlation of 1 means a perfect positive linear correlation (i.e. the factors form an upward-sloping straight line if plotted on a graph); a correlation of 0 means there is no correlation (as would occur if the factors were independent); and a correlation of -1 means perfect negative linear correlation (a downward-sloping straight line).
The agreement between effectiveness evaluation scores over two sets of systems can be measured by Kendall’s tau (Kendall, 1938), which is a standard procedure in information retrieval evaluation (Scholer, Turpin and Sanderson, 2011). Kendall’s tau measures the agreement in the ranking between two sets of paired values. The motivation for its use in information retrieval evaluation is that we primarily care about whether one system is better than another, but do not place much importance on the precise value of the effectiveness metric. We used Kendall’s tau to measure the agreement between the system rankings produced using worker and gold standard judgments. In this study, the independent-samples t-test, a parametric significance test, was used to compare two independent groups. The chi-squared test for independence was used to explore relationships between categorical variables. This test compares the observed proportion of cases that occur in each of the categories, and tests the null hypothesis that the population proportions are identical (Soboroff, Nicholas and Cahan, 2001).
Results and discussion
The effects of workers’ level of verbal comprehension skill on reliability of judgments
Workers were divided into two groups based on their verbal comprehension scores, the high values above the median and the low values below the median. A median split is one method for turning a continuous variable into a categorical one (Reis and Judd, 2000):
- Group 1 - low score: verbal comprehension score between 4 and 8, consisting of 156 tasks.
- Group 2 - high score: verbal comprehension score between 9 and 10, consisting of 163 tasks.
(As described previously, tasks with a verbal comprehension score below 4 were filtered out as spammers or as having no English language competence).
Agreement between Group 1 and TREC assessors (35.93% on relevant and 26.01% on not relevant) is 61.94%. The level of disagreement between them is 34.2% while 3.7% of workers chose “Don’t know”. The level of agreement between Group2 and TREC assessors is 75.9% (32.7% on relevant and 43.1% on not relevant), which is higher than that observed for Group1. The disagreement between Group 2 and TREC assessors and the percentage of those workers who chose “Don’t know” is 21.4% and 2.6%, respectively.
Cohen’s kappa agreement between the relevance judgments of crowdsourced workers and TREC assessors is 0.3 (fair agreement) for Group 1 and is 0.57 (moderate agreement) for Group 2. Apparently, Group 2 is more reliable in their judgments when compared with Group 1. Consistent with the results of a previous study (Alonso and Mizzaro, 2012), neither of the groups showed a strong agreement when compared with the gold standard. The study showed an agreement level of 68% between relevance judgments created by workers and relevance judgments provided by TREC assessors, which is a fair agreement. In another study (Al Maskari, Sanderson and Clough, 2008), the agreement between relevance judgments of TREC and non-TREC assessors (recruited to perform a search task) for fifty-six topics showed a moderate agreement.
Pearson’s correlation between verbal comprehension score and accuracy is 0.32 (p < 0.001). The verbal comprehension score shows a moderate but significant correlation with accuracy. Dividing workers into low and high score groups, we again see that higher verbal comprehension skill leads to higher accuracy (Figure 1), and there are significant differences in accuracy (t (317) = -5.20, p < 0.001) between Group1 (μ = 0.62, σ = 0.25) and Group2 (μ = 0.76, σ = 0.21).
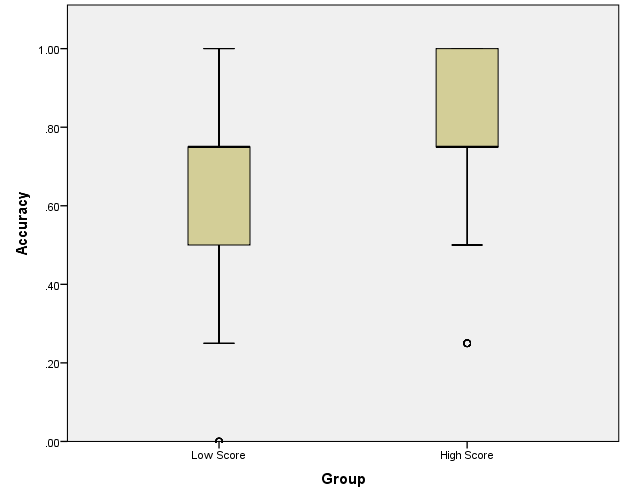
Figure 1: Accuracy in relation to verbal comprehension score.
The effects of workers’ level of verbal comprehension skill on system rankings
The influence of crowdsourced judgments on system rankings was assessed to find out whether crowdsourced judgments are reliable for evaluation purposes. One set of relevance judgments was generated from Group 1, and another set from Group 2. Multiple assessors assessed the documents, and majority voting method was used to aggregate the judgments. Each relevance judgment set consisted of 160 relevance judgments. The information retrieval systems that participated in the TREC-9 Web Track were then scored using Mean Average Precision (MAP), ranked using the Group 1 judgments, and then using the Group 2 judgments. Each of these rankings was compared to the ranking achieved by the systems on the original TREC assessments. Kendall’s tau was computed for this rank comparison. The Kendall’s tau correlation coefficients between workers and TREC assessors is shown in Table 1. Figure 2 presents the system rankings.
As explained earlier, ten topics were chosen in this study. In a typical system-based TREC experiment, it was suggested to use fifty topics to intensify the reliability of the experimental results (Buckley and Voorhees, 2000). However, average precision is considered to be a reasonably stable and discriminating option for general purpose retrieval. A plot was presented in (Buckley and Voorhees, 2000) showing the average error rate over 100 trials (where each trial's error rate is the average over the fifty permuted query sets) for each of the topic set sizes smaller than fifty. For all measures, the average error rate decreases as the number of topics increases. Precision (depth = 10) has a relatively higher error rate than mean average precision which has a relatively lower error rate at small topic set sizes (Buckley and Voorhees, 2000). In this study, we initially computed mean average precision at the evaluation depth of 1000. However, multiplying across the number of systems that participated in TREC-9 may suggest that the vast majority of topic-document pairs that occur in any particular system run will be unjudged. Usually, mean average precision will simply treat these (majority) unjudged items as being not relevant. This scenario could have a substantial impact on the metric scores. Hence, to address this issue, we considered calculating mean average precision to a shallower depth of 10.
Workers | Tau depth=10 | Tau depth=1000 |
---|---|---|
Group 1 (low score) | 0.73 | 0.86 |
Group 2 (high score) | 0.85 | 0.90 |
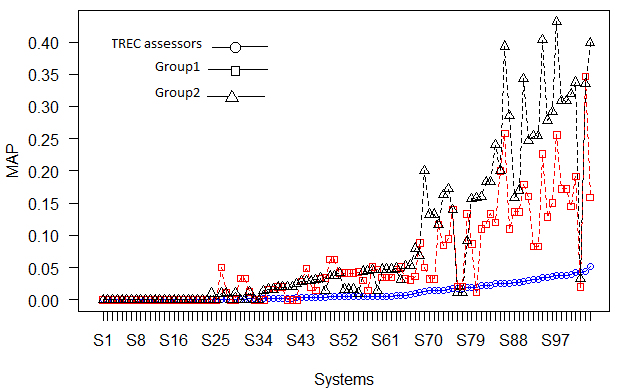
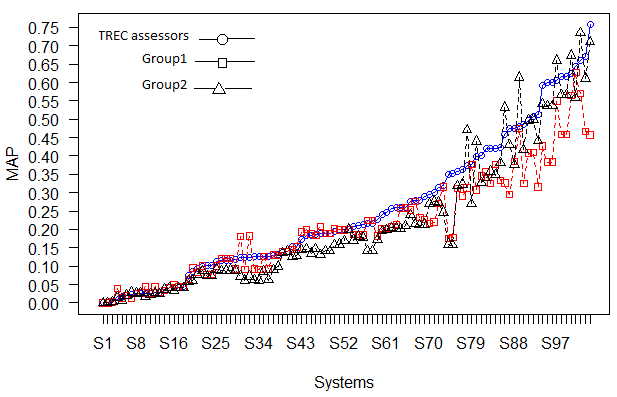
(In both figures the systems are sorted in ascending order of mean average precision scores generated using TREC assessors’ judgments)
There is a slightly higher correlation between TREC rankings and those using the Group 2 judgments (for depth 10 and 1000) than those using the Group 1 judgments. This trend reveals that the assessments performed by workers with high verbal comprehension skill to evaluate and rank retrieval systems by effectiveness are relatively similar to those of the official TREC assessments. This finding is consistent with previous studies, which reported that different judges have a little effect on system rankings. For instance, in a study investigating the effect of task design on the system rankings, the authors found that a full set of quality control methods can lead to better system rankings showing a high correlation with the system rankings of the gold set. Accordingly, they found that removing low accuracy workers had a slight effect on system ranking (Kazai, Kamps, Koolen and Milic-Frayling, 2011). In a separate study (Trotman and Jenkinson, 2007), the Spearman’s r rank correlation between multiple judges and gold set for sixty-four systems was 0.95. They concluded that different judges have a little effect on system rankings. However, a number of studies found that the variation in relevance judgments does not have an influence on system rankings (Lesk and Salton, 1968;C. W. Cleverdon, 1970;Kazhdan, 1979;Burgin, 1992). In a preliminary study (Voorhees, 2000), the relevance judgments of both National Institute of Standards and Technology (NIST) judges and University of Waterloo judges were compared for a TREC-6 dataset. The Kendall’s tau correlation between these two groups showed 0.896 for seventy-six systems ranked by mean average precision. The study concluded that the variation in relevance judgments rarely influences the system rankings.
The effect of self-reported worker features on relevance judgment accuracy
After judging the relevance of each topic and document, workers rated their confidence in their evaluation using a 4-point Likert scale. Table 2 summarizes the accuracy for each level of confidence, across 1276 relevance judgments. The result showed that less confident workers achieved lower accuracy rates for the relevance judgments, while confident workers achieved higher accuracy rates. The result of the chi-squared test for the relationship between confidence and accuracy was significant (χ2 = 20.05, p < 0.01).
Level | Number of judgments | Correct judgments | Accuracy | |
---|---|---|---|---|
Confidence in judgment | 1 | 44 | 21 | 0.47 |
2 | 170 | 103 | 0.60 | |
3 | 530 | 368 | 0.69 | |
4 | 532 | 391 | 0.73 | |
Difficulty of the judgment | 1 | 342 | 276 | 0.80 |
2 | 303 | 210 | 0.69 | |
3 | 541 | 345 | 0.63 | |
4 | 90 | 52 | 0.57 | |
Knowledge of the topic | 1 | 207 | 138 | 0.66 |
2 | 319 | 241 | 0.75 | |
3 | 469 | 335 | 0.71 | |
4 | 281 | 169 | 0.60 | |
Ratings in Table 2 are based on a 4-point Likert-type scale, ranged between level 1 and level 4 for minimum to maximum level of confidence in judgment/difficulty of the judgment/knowledge on the topic. |
Once workers had performed a relevance judgment evaluation of each topic and document, they were asked to rate the level of difficulty of the evaluation using a 4-point Likert scale. The accuracy was then calculated for each level of difficulty to find out whether the difficulty level of a judgment influences the accuracy of the performance. Table 2 shows the accuracy for each level of difficulty. Those workers who claimed that a task was difficult achieved lower accuracy, while the workers who found a task easy obtained higher accuracy. A chi-squared test for the relationship between difficulty and accuracy was significant (χ2 = 34.22, p < 0.01).
The workers were asked to rate their knowledge about a given topic, using a 4-point Likert scale. Interestingly, the results showed that those workers with extreme level of self-reported knowledge (either low or high) were less accurate when compared with those who rated their knowledge at the moderate level. The relationship is significant using the chi-squared test for equality of proportions (χ2 =18.56, p < 0.01), even though the relationship is apparently not monotonic (Table 2). This trend may be in conflict with what would be generally expected. There are several possibilities to justify this finding. Firstly, knowledge on the topic was self-reported and it might show their work in a better light. Secondly, the responses could refer to the workers’ attitude and confidence in their tasks. The reason why workers with high self-reported knowledge are apparently less reliable may be that incompetent workers have an inflated sense of their own knowledge (Behrend et al., 2011); or, if self-reported knowledge was accurate, it may be that those knowledgeable workers were more opinionated, and for that reason most likely to disagree with the original assessor on the relevance of an article to a topic. To summarize, our results seem consistent with a previous work which found that knowledge on the topic did not influence the accuracy of relevance judgments (Kazai et al., 2013), while contrasting with previous studies which found that knowledge on the topic and the task plays an important role in the accuracy of relevance judgments (Bailey et al., 2008;Kinney, Huffman and Zhai, 2008).
Conclusion
This article has presented the results of an experiment in which crowdsourced workers performed relevance assessments in the evaluation of information retrieval systems. Our objective was to explore the relationship between workers’ verbal comprehension skill and self-reported competence on the one hand, and assessment reliability on the other, where assessment reliability was measured as agreement with the original expert human assessors. We found a significant positive correlation between verbal comprehension skill and judgment reliability. Similarly, when the assessments of workers with high verbal comprehension skill were used to evaluate and rank retrieval systems by effectiveness, they gave a ranking more similar to that of the official assessments than when the assessments of workers with low verbal comprehension skill were used.
The findings around self-reported competence were more mixed. Workers who reported greater confidence in their assessments, and found the task easier, gave more reliable judgments. Workers reporting high knowledge on the topic, however, gave the least reliable judgments (or at least, those least likely to agree with the original assessors), while the more reliable workers where those reporting only moderate knowledge. Whether this surprising finding is due to the self-confidence of incompetence, or the opinionatedness of the capable, or to some other effect requires further study. In any case, relying on self-declared knowledge to gauge assessment reliability is clearly questionable, and more objective measures of ability should be sought.
In summary, our findings show that verbal comprehension skill influences the accuracy of crowdsourced workers who create the relevance judgments set. In the light of the findings, it is reasonable to argue that certain worker characteristics can be used to predict accuracy or to explain differences in accuracy between worker groups. Indeed, finding the relationship between cognitive abilities of crowdsourced workers and the reliability of relevance judgments can lead to new quality control approaches to improve the reliability of relevance judgments. However, as this experiment was conducted on a small dataset, in future work we are going to investigate whether these findings remain stable on a larger scale. The interesting result of the relationship between self-reported competence (confidence and difficulty) and reliability of relevance judgments motivates further investigation to utilize this competence in quality control approaches.
Acknowledgements
This research is supported by the Exploratory Research Grant Scheme (ER027-2013A), Ministry of Higher Education of Malaysia and by High Impact Research Grant (UM.C/625/1/HIR/MOHE/FCSIT/14), University of Malaya, Malaysia.
About the authors
Parnia Samimi received her Ph.D. in Information Technology in 2016 from Department of Information Systems, Faculty of Computer Science and Information Technology, University of Malaya, Malaysia. Her research interests are in information systems, evaluation of information retrieval systems and distributed computing (cloud computing). She can be contacted at: parniasamimi62@gmail.com.
Sri Devi Ravana received her Master of Software Engineering from University of Malaya, Malaysia in 2001, and PhD from the Department of Computer Science and Software Engineering, the University of Melbourne, Australia, in 2012. Her research interests include information retrieval heuristics, text indexing methods and ICT in learning. She is currently a Senior Lecturer at the Department of Information Systems, University of Malaya, Malaysia. She can be contacted at: sdevi@um.edu.my.
William Webber received his PhD at the University of Melbourne on measurement in information retrieval, and completed a post-doctoral research position in the e-discovery lab of the University of Maryland. He is currently an independent consultant on e-discovery and information retrieval. He can be contacted at: william@williamWebber.com.
Yun Sing Koh is a senior lecturer at the Department of Computer Science, The University of Auckland, New Zealand. She completed her PhD at the Department of Computer Science, University of Otago, New Zealand. Her research interest is in the area of data mining and machine learning, specifically data stream mining and pattern mining. She can be contacted at: ykoh@cs.auckland.ac.nz.
References
- Al Maskari, A. & Sanderson, M. (2011). The effect of user characteristics on search effectiveness in information retrieval. Information Processing & Management, 47(5), 719-729.
- Al Maskari, A., Sanderson, M. & Clough, P. (2008). Relevance judgments between TREC and non-trec assessors. In SIGIR '08: proceedings of the 31st Annual International ACM SIGIR Conference on Research and Development in Information Retrieval, , (pp. 683-684). New York, NY: ACM. Retrieved from http://eprints.whiterose.ac.uk/4510/1/pp908-almaskari.pdf (Archived by WebCite® at http://www.webcitation.org/6odPu4Iq3)
- Allahbakhsh, M., Benatallah, B., Ignjatovic, A., Motahari-Nezhad, H. R., Bertino, E. & Dustdar, S. (2013). Quality control in crowdsourcing systems: issues and directions. Internet Computing, IEEE, 17(2), 76-81. Retrieved from https://pdfs.semanticscholar.org/3982/559b5c155846ad90d4df58929eb3261d9a1f.pdf (Archived by WebCite® at http://www.webcitation.org/6odQTUmmt)
- Allen, B. (1992). Cognitive differences in end user searching of a CD-ROM index. In Proceedings of the 15th Annual International ACM SIGIR Conference on Research and Development in Information Retrieval, (pp. 298-309). New York, NY: ACM.
- Allen, B. & Allen, G. (1993). Cognitive abilities of academic librarians and their patrons. College & Research Libraries, 54(1), 67-73.
- Alonso, O. (2012). Implementing crowdsourcing-based relevance experimentation: an industrial perspective. Information Retrieval, 16(2), 101-120.
- Alonso, O. & Mizzaro, S. (2012). Using crowdsourcing for TREC relevance assessment. Information Processing & Management, 48(6), 1053-1066.
- Alonso, O., Rose, D. E. & Stewart, B. (2008). Crowdsourcing for relevance evaluation. ACM SIGIR Forum, 42(2), 9-15. Retrieved from http://www.sigir.org/files/forum/2008D/papers/2008d_sigirforum_alonso.pdf (Archived by WebCite® at http://www.webcitation.org/6odSGX70Q)
- Bailey, P., Craswell, N., Soboroff, I., Thomas, P., de Vries, A. P. & Yilmaz, E. (2008). Relevance assessment: are judges exchangeable and does it matter. In SIGIR '08: proceedings of the 31st Annual International ACM SIGIR Conference on Research And Development in Information Retrieval (pp. 667-674). New York, NY: ACM. Retrieved from http://es.csiro.au/pubs/bailey_sigir08.pdf (Archived by WebCite® at http://www.webcitation.org/6oe6xv0aK)
- Beaty, R. E., Silvia, P. J., Nusbaum, E. C., Jauk, E. & Benedek, M. (2014). The roles of associative and executive processes in creative cognition. Memory & Cognition, 42(7), 1186-1197.
- Behrend, T. S., Sharek, D. J., Meade, A. W. & Wiebe, E. N. (2011). The viability of crowdsourcing for survey research. Behavior Research Methods, 43(3), 800-813.
- Blanco, R., Halpin, H., Herzig, D. M., Mika, P., Pound, J., Thompson, H. S. & Tran Duc, T. (2011). Repeatable and reliable search system evaluation using crowdsourcing. In SIGIR '11: proceedings of the 34th Annual International ACM SIGIR Conference on Research and Development in Information Retrieval (pp. 923-932). New York, NY: ACM. Retrieved from http://livingknowledge.europarchive.org/images/publications/Sigir2011-crowd-search-evaluation.pdf (Archived by WebCite® at http://www.webcitation.org/6oe7KPwH2)
- Brennan, K., Kelly, D. & Arguello, J. (2014). The effect of cognitive abilities on information search for tasks of varying levels of complexity. In Proceedings of the 5th Information Interaction in Context Symposium (pp. 165-174). New York, NY: ACM. Retrieved from https://ils.unc.edu/~jarguell/BrennanIIIX14.pdf (Archived by WebCite® at http://www.webcitation.org/6oe7bY3Qs)
- Buckley, C. & Voorhees, E. M. (2000). Evaluating evaluation measure stability. In SIGIR '00: proceedings of the 23rd Annual International ACM SIGIR Conference on Research and Development in Information Retrieval (pp. 33-40). Retrieved from http://lipn.fr/~rozenknop/Cours/MICR_REI/articles-RI/BuckleyVoorhees2000.pdf (Archived by WebCite® at http://www.webcitation.org/6oe7kKz8h)
- Burgin, R. (1992). Variations in relevance judgments and the evaluation of retrieval performance. Information Processing & Management, 28(5), 619-627.
- Cleverdon, C. (1967). The Cranfield tests on index language devices. Aslib Proceedings, 19(6), 173-194. Retrieved from https://www.ischool.utexas.edu/~stratton/rdgs/Cleverdon.pdf (Archived by WebCite® at http://www.webcitation.org/6oe7rQf6t)
- Cleverdon, C. W. (1970). The effect of variations in relevance assessments in comparative experimental tests of index languages. Cranfield, UK: Cranfield Institute of Technology. (Cranfield Library Report No. 3).
- Clough, P., Sanderson, M., Tang, J., Gollins, T. & Warner, A. (2012). Examining the limits of crowdsourcing for relevance assessment. IEEE Internet Computing, 17(4), 32-38. Retrieved from http://marksanderson.org/publications/my_papers/IEEE-IC-2012.pdf (Archived by WebCite® at http://www.webcitation.org/6oe81sbzc)
- De Alfaro, L., Kulshreshtha, A., Pye, I. & Adler, B.T. (2011). Reputation systems for open collaboration. Communications of the ACM, 54(8), 81-87. Retrieved from https://users.soe.ucsc.edu/~luca/papers/11/cacm2010.pdf (Archived by WebCite® at http://www.webcitation.org/6oe8Bn2pO)
- Ekstrom, R.B., French, J.W., Harman, H.H. & Dermen, D. (1976). Manual for kit of factor-referenced cognitive tests. Princeton, NJ: Educational Testing Service.
- Gadiraju, U., Kawase, R., Dietze, S. & Demartini, G. (2015). Understanding malicious behavior in crowdsourcing platforms: the case of online surveys. In Proceedings of the 33rd Annual ACM Conference on Human Factors in Computing Systems (pp. 1631-1640). New York, NY: ACM. Retrieved from http://eprints.whiterose.ac.uk/95877/1/Understanding%20malicious%20behaviour.pdf (Archived by WebCite® at http://www.webcitation.org/6oe8Iv55O)
- Geary, D. C., Hoard, M. K., Nugent, L. & Bailey, D. H. (2013). Adolescents’ functional numeracy is predicted by their school entry number system knowledge. PloS one, 8(1), e54651. Retrieved from http://journals.plos.org/plosone/article?id=10.1371/journal.pone.0054651 (Archived by WebCite® at http://www.webcitation.org/6oe8Tyd99)
- Grady, C. & Lease, M. (2010). Crowdsourcing document relevance assessment with mechanical turk. In Proceedings of the NAACL HLT 2010 Workshop on Creating Speech and Language Data with Amazon's Mechanical Turk (pp. 172-179). Stroudsburg, PA: Association for Computational Linguistics. Retrieved from http://anthology.aclweb.org/W/W10/W10-07.pdf#page=186 (Archived by WebCite® at http://www.webcitation.org/6oe8c1h55)
- Hammon, L. & Hippner, H. (2012). Crowdsourcing. Business & Information Systems Engineering, 54(3), 165-168.
- Heer, J. & Bostock, M. (2010). Crowdsourcing graphical perception: using mechanical turk to assess visualization design. In Proceedings of the 28th International Conference on Human Factors in Computing Systems (pp. 203-212). New York, NY: ACM. Retrieved from http://www.cs.kent.edu/~javed/class-P2P12F/papers-2012/PAPER2012-2010-MTurk-CHI.pdf (Archived by WebCite® at http://www.webcitation.org/6oe8tfKYS)
- Howe, J. (2006). The rise of crowdsourcing. Wired Magazine, 14(6), 1-4. Retrieved from http://sistemas-humano-computacionais.wdfiles.com/local--files/capitulo%3Aredes-sociais/Howe_The_Rise_of_Crowdsourcing.pdf (Archived by WebCite® at http://www.webcitation.org/6oe98KuLJ)
- Ipeirotis, P. (2010). Demographics of mechanical turk. XRDS: Crossroads, The ACM Magazine for Students: Comp-YOU-Ter 17,(2), 16-21. Retrieved from https://archive.nyu.edu/bitstream/2451/29585/2/CeDER-10-01.pdf (Archived by WebCite® at http://www.webcitation.org/6oe9LK7g7)
- John, O., Naumann, L. & Soto, C. (2008). Paradigm shift to the integrative Big Five trait taxonomy. In O.P. John, R.W. Robins & L.A. Pervin (Eds.), Handbook of personality: theory and research (3rd. ed., pp. 114-158). New York, NY: Guilford Press.
- Kazai, G. (2011). In search of quality in crowdsourcing for search engine evaluation. Advances in Information Retrieval: proceedings of the 33rd European Conference on IR Research, ECIR 2011, Dublin, Ireland, April 18-21, 2011 (pp. 165-176). Berlin: Springer. Retrieved from http://ai2-s2-pdfs.s3.amazonaws.com/5480/a8f3f6d89f3019aa373e91220e19f2d3a506.pdf (Archived by WebCite® at http://www.webcitation.org/6oe9VxEjE)
- Kazai, G., Kamps, J., Koolen, M. & Milic-Frayling, N. (2011). Crowdsourcing for book search evaluation: impact of hit design on comparative system ranking. In SIGIR '11: Proceedings of the 34th International ACM SIGIR Conference on Research and Development in Information Retrieval (pp. 205-214). New York, NY: ACM. Retrieved from http://humanities.uva.nl/~kamps/webart/publications/2011/kaza:crow11.pdf (Archived by WebCite® at http://www.webcitation.org/6oe9hptN0)
- Kazai, G., Kamps, J. & Milic-Frayling, N. (2011). Worker types and personality traits in crowdsourcing relevance labels. In CIKM '11: proceedings of the 20th ACM International Conference on Information and Knowledge Management (pp. 1941-1944). New York, NY: ACM. Retrieved from https://humanities.uva.nl/~kamps/readme/publications/2011/kaza:work11.pdf (Archived by WebCite® at http://www.webcitation.org/6oe9rjKf3)
- Kazai, G., Kamps, J. & Milic-Frayling, N. (2012). The face of quality in crowdsourcing relevance labels: demographics, personality and labeling accuracy. In CIKM '12: proceedings of the 21st ACM International Conference on Information and Knowledge Management (pp. 2583-2586). New York, NY: ACM. Retrieved from http://www.e.humanities.uva.nl/publications/2012/kaza:face12.pdf (Archived by WebCite® at http://www.webcitation.org/6oeA6Y1TT)
- Kazai, G., Kamps, J. & Milic-Frayling, N. (2013). An analysis of human factors and label accuracy in crowdsourcing relevance judgments. Information Retrieval, 16(2), 138-178. Retrieved from http://e.humanities.uva.nl/publications/2013/kaza:anal13.pdf (Archived by WebCite® at http://www.webcitation.org/6oeACQo1s)
- Kazhdan, T. (1979). Effects of subjective expert evaluation of relevance on the performance parameters of document-based information retrieval system. Nauchno-Tekhnicheskaya Informatsiya, Seriya, 2(13), 21-24.
- Kendall, M. G. (1938). A new measure of rank correlation. Biometrika, 30(1/2), 81-93.
- Kinney, K.A., Huffman, S.B. & Zhai, J. (2008). How evaluator domain expertise affects search result relevance judgments. In CIKM ;08: proceedings of the 17th ACM Conference on Information and Knowledge Management (pp. 591-598). New York, NY: ACM. )
- Landis, J. R. & Koch, G. G. (1977). The measurement of observer agreement for categorical data. Biometrics, 33(1), 159-174. Retrieved from http://www.dentalage.co.uk/wp-content/uploads/2014/09/landis_jr__koch_gg_1977_kappa_and_observer_agreement.pdf (Archived by WebCite® at http://www.webcitation.org/6oep0UoRZ)
- Lesk, M. E. & Salton, G. (1968). Relevance assessments and retrieval system evaluation. Information Storage and Retrieval, 4(4), 343-359.
- Mason, W. & Suri, S. (2012). Conducting behavioral research on Amazon’s mechanical turk. Behavior Research Methods, 44(1), 1-23. Retrieved from http://smash.psych.nyu.edu/courses/spring12/lhc/readings/SSRN-id1691163.pdf (Archived by WebCite® at http://www.webcitation.org/6oepgeFkc)
- Mason, W. & Watts, D. J. (2010). Financial incentives and the performance of crowds. ACM SigKDD Explorations Newsletter, 11(2), 100-108. Retrieved from https://pdfs.semanticscholar.org/1ae6/228dccf569d6990c7afc31282c40f9da23bc.pdf (Archived by WebCite® at http://www.webcitation.org/6oepFAYsh)
- Pallant, J. (2001). SPSS survival manual: a step by step guide to data analysis using SPSS for Windows (Versions 10 and 11): SPSS Student Version 11.0 for Windows. Milton Keynes, UK: Open University Press.
- Paolacci, G., Chandler, J. & Ipeirotis, P. (2010). Running experiments on Amazon mechanical turk. Judgment and Decision Making, 5 (5), 411-419. Retrieved from http://sjdm.cybermango.org/journal/10/10630a/jdm10630a.pdf (Archived by WebCite® at http://www.webcitation.org/6oepuCG6W)
- Potthast, M., Stein, B., Barrón-Cedeño, A. & Rosso, P. (2010). An evaluation framework for plagiarism detection. In COLING '10: proceedings of the 23rd International Conference on Computational Linguistics: Posters (pp. 997-1005). Stroudsburg, PA: Association for Computational Linguistics. Retrieved from http://www.anthology.aclweb.org/C/C10/C10-2115.pdf (Archived by WebCite® at http://www.webcitation.org/6of20pGwM)
- Quinn, A. J. & Bederson, B. B. (2011). Human computation: a survey and taxonomy of a growing field. In CHI '11: proceedings of the 2011 Annual Conference on Human Factors in Computing Systems (pp. 1403-1412). New York, NY: ACM. Retrieved from http://crowdsourcing-class.org/readings/downloads/intro/QuinnAndBederson.pdf (Archived by WebCite® at http://www.webcitation.org/6oeq34Xpn)
- Reis, H. T. & Judd, C. M. (2000). Handbook of research methods in social and personality psychology. Cambridge: Cambridge University Press.
- Ross, J., Irani, L., Silberman, M. S., Zaldivar, A. & Tomlinson, B. (2010). Who are the crowdworkers?: shifting demographics in mechanical turk. In CHI EA '10: Chi '!) Extended abstracts on Human Factors in Computing Systems (pp. 2863-2872). New York, NY: ACM. Retrieved from http://bit.ly/2mnIQPJ (Archived by WebCite® at http://www.webcitation.org/6oeqH7RMl)
- Salthouse, T. A. (2014). Frequent assessments may obscure cognitive decline. Psychological Assessment, 26(4), 1063-1069. Retrieved from https://pdfs.semanticscholar.org/f4aa/c97f2e1057df1b70944270c102ead4c55430.pdf (Archived by WebCite® at http://www.webcitation.org/6oeqPHmJ5)
- Scholer, F., Kelly, D., Wu, W.-C., Lee, H. S. & Webber, W. (2013). The effect of threshold priming and need for cognition on relevance calibration and assessment. Proceedings of the 36th International ACM SIGIR Conference on Research and Development in Information Retrieval (pp. 623-632). Retrieved from http://williamwebber.com/research/papers/skwlw13sigir.pdf (Archived by WebCite® at http://www.webcitation.org/6oeqTu1Mc)
- Scholer, F., Turpin, A. & Sanderson, M. (2011). Quantifying test collection quality based on the consistency of relevance judgements. In SIGIR '11: proceedings of the 34th International ACM SIGIR Conference on Research and Development in Information Retrieval (pp. 1063-1072). New York, NY: ACM. Retrieved from http://marksanderson.org/publications/my_papers/sigir2011-scholer.pdf (Archived by WebCite® at http://www.webcitation.org/6oerOz1UU)
- Soboroff, I., Nicholas, C. & Cahan, P. (2001). Ranking retrieval systems without relevance judgments. In SIGIR '01: proceedings of the 24th International ACM SIGIR Conference on Research and Development in Information Retrieval (pp. 66-73). New York, NY: ACM. )
- Trotman, A. & Jenkinson, D. (2007). IR evaluation using multiple assessors per topic. In Proceedings of the 12th Australasian Document Computing Symposium, Melbourne, Vic. (pp. 9-16). Retrieved from http://ai2-s2-pdfs.s3.amazonaws.com/76ea/86f077094ef5aa9a1b1bea38c2c64ddee7bd.pdf (Archived by WebCite® at http://www.webcitation.org/6oerhJJ6i))
- Voorhees, E. M. (2000). Variations in relevance judgments and the measurement of retrieval effectiveness. Information Processing and Management, 36(5), 697-716. Retrieved from http://ai2-s2-pdfs.s3.amazonaws.com/162c/68e07814704109122d61771c1ce067e95b86.pdf (Archived by WebCite® at http://www.webcitation.org/6oertv5SJ)
- Voorhees, E. M. & Harman, D. K. (Eds.)(2005). TREC: experiment and evaluation in information retrieval. Cambridge, MA: MIT Press.
- Zhao, Y. & Zhu, Q. (2012). Evaluation on crowdsourcing research: current status and future direction. Information Systems Frontiers, 1-18. Retrieved from http://bit.ly/2mZSdSV (Archived by WebCite® at http://www.webcitation.org/6oewb7IQp)
- Zhu, D. & Carterette, B. (2010). An analysis of assessor behavior in crowdsourced preference judgments. SIGIR 2010 Workshop on Crowdsourcing for Search Evaluation (pp. 17-20). New York, NY: ACM. Retrieved from http://ir.ischool.utexas.edu/cse2010/materials/zhucarterette.pdf (Archived by WebCite® at http://www.webcitation.org/6oL7bz6uk)