Task complexity affects information use: a questionnaire study in city administration
Miamaria Saastamoinen, Sanna Kumpulainen, Pertti Vakkari and Kalervo Järvelin
School of Information Sciences, University of Tampere, Finland
Introduction
People perform various tasks both during their working and leisure time. Some of these tasks include information seeking and searching. In order to be able to explain variation in information seeking practices, it is important to understand the underlying task and its characteristics. The knowledge on the features of task performance contributes to the development of more suitable information systems and information seeking or working practices. Especially task complexity has proved to have a notable effect on information seeking practices: the more complex the task, the more complex information needs and information seeking. People also tend to underestimate the information seeking needed in complex tasks and overestimate it in simple tasks. (Byström 1999).
Task-based information seeking has been investigated using a range of approaches and methodologies. Vakkari (2003) has written a thorough review of task-based information seeking research and Ingwersen and Järvelin (2005) emphasise the need for a research programme that would combine the findings of information seeking and information retrieval into a task-based paradigm. The concept of task and task characteristics have been theoretically elaborated by, for example, Li and Belkin (2008), Byström and Hansen (2005) and Campbell (1988). Task-based information seeking and searching research has covered various domains, such as city administration (Byström 1999; Saastamoinen et al. 2012), patent domain (Hansen 2011), academic research (Wang et al.2007) and molecular medicine (Kumpulainen and Järvelin 2010), not to mention the research on students' task-based information activities (Kuhlthau 1993; Vakkari 2000; Vakkari et al. 2003).
While there are several studies on task-based information seeking, the domain still needs more research on different tasks in different domains. The present study focuses on tasks in administrative context. The studied tasks are real work tasks that are performed by real performers in real situations. The tasks are classified according to their complexity. Our study contributes to the knowledge on task-based information seeking in the administrative domain. It is a successor of Byström and Järvelin's (1995) and Byström's study (1999) because it was partly conducted in the same city administration context (Byström (1999) had two cities in inspection) and thus in the same working environment with a similar approach. However, they did not analyse the expected information needs. In our study, we compare expected and materialised information use. Naturally, administrative working settings have changed quite much especially regarding their information environment. In the 1990s, it was common that there were only a few independent. computerised information systems in use in an organization, and the Web was just emerging. Comparing the results of these two studies contributes to understanding the evolution of information seeking activities in organizational settings.
The specific research questions in the present paper are:
- What are the shares of internal and external information and how do they deviate across task complexity categories?
- What are the expected and materialised information types and how do their shares deviate across task complexity categories?
This study is a part of a larger research project, where the participants were shadowed, in addition to the questionnaires. The results based on the shadowing data are discussed in Saastamoinen et al. (2012).
The paper is structured as follows: first, we make a brief review of related studies. Secondly, we present the methods and data of the study. Then we discuss the findings, and finally, the research questions are answered in the conclusion.
Literature review
Byström and Hansen (2005), Li and Belkin (2008) and Vakkari (2003) discuss the concept of task and the different aspects task performance has in information studies literature. For example, in the information seeking model of Leckie et al. (1996), work roles affect tasks and tasks affect the nature of information needs. Moreover, Byström (1999) finds that the level of ambition is connected to task types.
Task complexity is one of the key features of tasks. The use of task complexity in informing sciences has been reviewed by Gill and Hicks (2006; see also Cohen 2009). Task complexity has been defined in many ways in research. According to Campbell (1988), these interpretations of task complexity can be divided into three major categories: task complexity is either a) mostly caused by the features of task performer; b) caused by objective features of the task; or c) a combination of these two.
Byström and Järvelin (1995) divide tasks into five complexity categories according to the degree of a priori determinability of task information, process and outcome. The extremes are automatic information processing tasks that could be fully automated and genuine decision tasks that are caused by completely unforeseeable upheavals. This classification is modified into three categories in Kumpulainen and Järvelin (2010), where each task session is assigned a complexity category depending on how many of the three task components (resources, process, outcome) are known to the task performer beforehand.
A priori determinability of a task is highly dependent on task performer if estimated by the performer for a task at hand. On the other hand, we can argue that if estimated for more abstract task types, this complexity definition becomes more objective. That is to say that some tasks are more complex, demanding or unclear than others regardless of the performer, as argued by Campbell (1988). The objectivity of task complexity should not be a question of right or wrong, however. In fact, Allen (1996) found that the participants' actual knowledge affected information seeking less than the knowledge they perceived to possess. Similarly, Saastamoinen et al. (2012) discovered that participants' perceived task complexity has clearer effects on information searching than their advance knowledge of the task.
Li and Belkin (2008) base their theoretical task categorisation on earlier categorisations in information research literature. It is extensive but so multifaceted that it is difficult to exploit in empirical studies, though it may be applied when comparing the categorisations of different studies. In the categorisation, task complexity is divided in objective and subjective parts. Objectivity here means the number of paths between which the task performer has to choose during the task. They also have a different category called difficulty, which is said to be subjective. On the other hand, the researchers do not view a priori determinability either as part of difficulty or of complexity. (Li and Belkin 2008.)
The information seeking process is kindled by information needs. They can be described as anomalous states of knowledge (Belkin 1980) or as a gap to be crossed (Dervin 1983), for example. Further, Allen (1996) argues that information needs can only be observed indirectly, through information seeking activities. Case (2007) discusses different researchers' reasoning about information needs.
Eventually, an information seeking process ends up in information use. Kari (2010) discovers seven conceptions of information needs in information studies literature. According to Kari (2010), the conceptions vary from modifications in knowledge structures to consuming information instrumentally, or even producing new information. As a matter of fact, contemporary digital information environments enable almost simultaneous information seeking and information use as an information object can be gained, interpreted, modified, utilised and forwarded in a single session using a single computer, for instance (Blandford and Attfield 2010).
In the present paper, both information needs and information use are understood fairly instrumentally as parts of achieving the goal, the task outcome. The participants list potential information used in a task before commencing it; this can be interpreted as information needs. On the other hand, the listed information needs may as well be only on a prospective level; some information may not be even needed or used in the end, for various reasons. The list of used information in the end of the task obviously indicates information use of a sort but we cannot tell the nature of it. Nonetheless, the use is firmly connected to the benefits the information is expected to bring about.
Bearing in mind that information seeking is aimed at finding information, we study different information types and their relations to tasks of different complexity. Information types can be categorised on different levels of abstraction. Below, we will describe a few interesting categorisations used in research literature.
Byström (1999) categorises information into three categories based on its nature or ways of use: task information, domain information and task-solving information. Task information refers to information dealing with exclusively the task at hand. The information is typically in the form of facts (names, dates). The second information type, domain information, refers to general information dealing with the task subject. Thirdly, task-solving information indicates the means and methods to perform the task, e.g., information about what task and domain information is needed and what stages the task includes. In other words, task-solving information is methodological or procedural information. Additionally, a division between an organization's internal and external information sources is made. (Byström 1999.) We apply a similar internality division to information types.
Gorman's (1995) information types are closely related to Byström's (1999) though they are slightly more specific and named differently. Gorman (1995) outlines five types of information that physicians need in their work. Information needed may concern only one patient, statistics about patients in general, generic medical knowledge that can be easily extrapolated, procedural information (how to correctly perform one's own tasks) or social information (how others perform their tasks). (Gorman 1995.)
Morrison (1993) has similar information types to Gorman's (1995), although her categorisation focuses on the social aspects of work at the expense of substance matters of the tasks. Morrison's (1993) five information types concern procedural information, role expectations, expected behaviour both when performing the tasks and outside them, and performance feedback, that is, evaluative information about the task performance.
A typical way of classifying information searches is dividing them in known-item, factual and general searches (Ingwersen 1986, Toms 2011). We applied a similar classification to the information types in our data (see next section). This classification concerns clearly the form of the information, not its contents or expected uses and for this reason it can be easily applied to different environments and different tasks. Byström's (1999) task information resembles searching for facts (narrowly exploitable information) and domain information searching for general information (widely exploitable information), respectively.
In contrast to the examples above, Vakkari (2000) has two categorisations for information types in his study, namely types of information sought and contributing information types. This division resembles division between information needs and information use. Sought information has only three categories that describe how general the information is. By contrast, seven contributing information types represent more precisely the participating student group by categories such as theories and methods. (Vakkari 2000.)
Study design: participants, methods and data
The organization studied was the administration of a city of more than 200,000 inhabitants. The city arranges the statutory services based on the purchaser-provider model. The recruitment of the participants was taken care of by a contact person. After obtaining a name list we contacted the volunteers by e-mail and arranged a collective meeting with them to hear about their work and get them acquainted with the study. After that we sent them an orientation form to complete and began to agree on dates for data collection sessions by e-mail.
Our participants were five females and a male working in the purchasing sector. Two of them had subordinates. One half of the participants worked mainly in administrative duties, the other half in planning duties. The administrative duties included secretarial tasks such as preparing records and agendas, completing license applications and sending record excerpts. Planning duties included writing enclosures for calls for bids, replying to requests for account from other offices and untangling the effects of new residential areas on public services. The participants had working experience in same or similar tasks ranging from 1 year and 5 months to 25 years. Initially, we had seven participants in the study but unfortunately one of them had an insufficient number of tasks suitable for our study. We had to abandon data collection with this participant after a few sessions.
The questionnaire consisted of three electronic forms completed by task performers. Every participant completed an orientation form once, before the actual data collection phase. The questions concerned their work, tasks and information seeking. The purpose of this form was to provide the researcher with a preconception for the data collection (see Appendix 1). The task initiation (see Appendix 2) and task finishing (see Appendix 3) forms were completed at the beginning and in the end of every task. The questionnaire forms were founded on Byström's (1999) diary forms for ensuring the comparability of the results, and also because Byström's (1999) questionnaire was well tried. Only smaller revisions were made to the forms in order to better suit the present study and its specific research interests.
In the questionnaire forms, there were in all six questions concerning task complexity. In two of them, the task performer was requested to directly estimate the task complexity before beginning the task and after its completion. Three of the questions were about the task performer's own estimates of their knowing the task process, outcome and the information needed in the task beforehand. The more they knew, the simpler the task. In the final question, the participants were requested to estimate their expertise concerning each task. All these estimates were given in percentages. In the analysis phase, each task was assigned a composite complexity measure based on the questionnaire answers. The final complexity of a task is simply the mean of the five above mentioned complexity estimates; that is expertise, initial and final task complexity, the task performer's knowledge of task process and information needed. A priori knowledge of task outcome was omitted from the complexity measure. This was done because participants appeared to base their answers on different grounds; some understood 'outcome' as a content matter (such as the actual place where a school be established), some as the form of the outcome (such as the fact that the school will be placed somewhere).
Cronbach's alpha (1951) for the final composite measure of complexity was 0.79 (confidence interval 0.69-0.87), which is satisfactory. The tasks were divided in three complexity categories (simple, semi-complex and complex). Another way of calculating complexity from the same original data is demonstrated in Saastamoinen et al. (2012).
Mostly, we used task complexity categories in the calculations, but in some cases the exact complexity of each task was needed, such as when calculating Pearson's correlation coefficients. Categories were formed based on the size of each category so that each of them contained approximately equally many tasks. Above all the categories illustrate the tasks' relative complexity compared to other tasks in the data, as the object of the study is to compare information seeking in tasks of different complexities. Other categorisations were considered, but categories of different sizes could have caused distortions in the results because of the low number of tasks. Furthermore, the data did not seem to cluster in any natural complexity categories.
In the analysis phase, we compared tasks of different complexity categories regarding the expected and materialised use of information types. Expected information is information that participants expected to use during the task process (task initiation form) and materialised information is information they reported using after task completion (task finishing form). In the task finishing form, we also asked them whether the information was found and if it was adequate (on a scale of one to five). In addition to the expected and materialised information types, we calculated the distribution of dropped initial (not finally used in the task) information types, and unexpected new ones (not known to be used before the task), in each task complexity category.
We categorise information in two different ways: firstly, every piece of information mentioned in the forms is either internal or external to the organization regarding the place where it was produced. The second categorisation is between information types. The participants defined information (objects) in three quite distinguishable ways, which were 1) known items, such as a certain book or file without any explanation of what kind of information is desired from it; 2) facts, such as a name of a new manager; or 3) information aggregates, a subject or a bunch of facts needed.
In our main inspection all above mentioned information types are equally weighty and each piece of information is calculated once so that ten facts equal ten and an information aggregate equals one, for instance. In results section, we discuss both the mean shares and the absolute number of information types in each task complexity category. Mean share is the average proportion of an information type in a task complexity category, and mean count is the average number of an information type in a task complexity category, respectively.
We may argue that these information types can be arranged in order of growing complexity. Therefore we scored every information type according to its complexity. We scored them as follows: facts = 1, known items = 2 and information aggregates = 3. Information types in a task could obtain scores ranging from 1 to the maximum of 6, if all information types were needed. By comparison, we also changed this ordinal scale into the interval one and weighted the information types as follows: facts = 1, known items = 5 and information aggregates = 10. The information type complexity of a task could thus range from 1 to 16. These weighting factors are of course arbitrary but they provide further insight into the relationships of information types and task complexity. For the sake of comparison, we also counted the number of different information types in each task, ranging from 1 to 3.
Briefly, we ended up having three weighting schemes, namely 1-1-1, 1-2-3 and 1-5-10. In the weighting process, every information type was calculated only once so that for example one fact weighted as much as ten in a task. This decision had two reasons: firstly, the number of each information type used could already be seen in the unweighted measurements. Secondly, the complexity (i.e. diversity) of information types used does not increase whether there are for example several known items or just one. The complexity of information types is calculated both before and after task performance.
The distribution of the data and the number of, and the measuring level of, variables (nominal, ordinal etc.) set the preconditions in selecting suitable tests. The statistical tests applied and their significance levels are reported with the results.
Findings
Overview of tasks
The tasks in the data set were relatively simple. Task complexity as measured by a scale from 0 to 100 varied from 2% to 67.4%, the mean being 27%. Complex tasks were performed more seldom than simple ones: half of the simple tasks were performed weekly and 85% of complex tasks were performed every month or less frequently. Semi-complex tasks were performed quite evenly weekly, every month or more seldom. None of the tasks were reported to be performed on a daily basis.
The participants' work roles affected task complexity in quite a straightforward way: administrative staff performed most of the simple tasks and planners most of the complex ones (see Figure 1). The differences were statistically significant (Pearson Χ2, p=0.002).
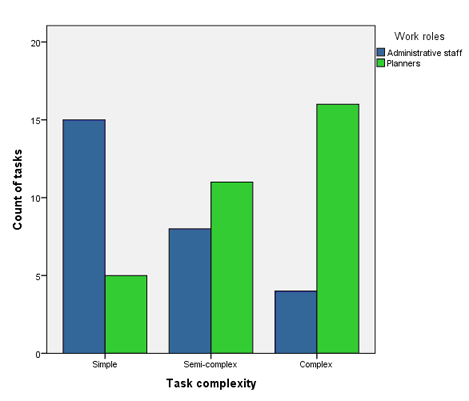
Figure 1: Work roles and task complexity.
Differences in perceived task complexity resembled what could be expected based on participants' positions. This finding seems to support the validity of our combined complexity measure. The planning tasks contain many wild cards whereas the nature of administrative tasks is to be quite routine-like. Nevertheless, planners had to perform administrative tasks as well (such as applying for a leave) and not all the tasks of the administration were so called routine but demanded context-sensitive reflection (such as appraising the competence of deputy candidates).
In the task initiation forms the participants were enquired if their aim was mainly to get the task quickly out of the way, to get it well performed or if they are only satisfied with an excellent result. In the task finishing forms they were enquired if they were satisfied with the result (on a four point scale). These two were associated in an interesting way: the more ambitious the goal, the more satisfied the participants were with the result (Pearson Χ2, p=0.009). On the other hand, task complexity did not have an effect on either the goal or the satisfaction. Some participants tended to be more satisfied with their task outcomes than others but the goals were independent of the task performer.
Information internality
Across all complexity categories, internal information was more popular than external. In total, only 20 % of expected information and 16 % of materialised information was external. The differences between the use of internal and external information, both in terms of expected and materialised use, are statistically significant at all task complexity levels (Wilcoxon, p=0.000-0.006). The participants expected that they would use less internal information (both absolutely and proportionally) in simple than in complex tasks (see Figure 2). The use that materialised was quite the opposite: the share of internal information was bigger in simple than in complex tasks. That is, internal information was insufficient to assuage their information needs in complex tasks unlike they expected. Deviating from that, the participants predict the share of the need for internal and external information accurately in semi-complex tasks. Interestingly, semi-complex tasks have a peak of both expected and materialised use of internal information being over 90 % on average (see Figure 2).
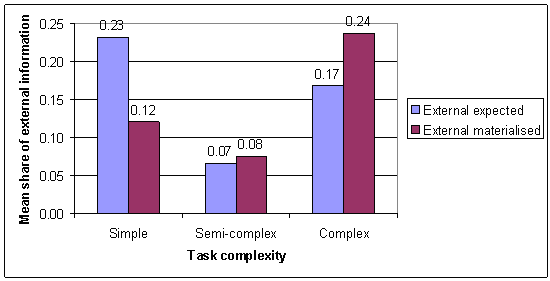
Figure 2: The mean shares of expected and materialised external information use in different task complexity categories.
In addition, the differences between the frequency of expected and materialised internal information use are statistically significant in simple tasks (t-test, p=0.021). That is, the expectations of usage of internal information differ most in simple tasks, which is quite surprising as simple tasks should be easily predictable by definition. Figure 3 illustrates the differences between the frequency of internal and external resources.
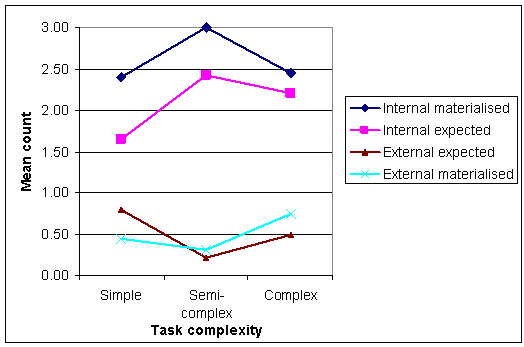
Figure 3: The mean count of expected and materialised internal and external resources.
In spite of the clear distinctions between the use of internal and external information, task complexity in itself does not appear to affect the internality substantially. It only affects the share of internality of materialised information use but the Pearson correlation of task complexity and information internality is only -0.26 (p=0.043). Consequently, the share of external information used increases a little with growing task complexity.
Internality of abandoned initial information. The more complex the task, the greater the amount of internal information that is abandoned during the task process, and the less abandoned external information, respectively. This means that, in simple tasks, less than 60% of abandoned information is internal, whereas in semi-complex and complex tasks over 90% of abandoned information is internal. The difference between the internal and external abandoned information (both absolutely and proportionally) is statistically significant in semi-complex and complex tasks (Wilcoxon signed ranks, p=0.004-0.020). The Pearson correlation between the share of internality of abandoned information and task complexity is notable (0.59) and statistically significant (p=0.004).
Internality of new, unexpected information. There tends to be a larger number of new external information needs in complex tasks than in simpler tasks. However, no such a clear linear trend holds for the number of new internal information. Proportionally, 83% of newcomers in simple tasks and 70 % of newcomers in complex tasks are internal, whereas 89% of newcomers are internal in semi-complex tasks. The difference between the internal and external newcomers (both absolutely and proportionally) is statistically significant in simple and semi-complex tasks (Wilcoxon signed ranks, p=0.002-0.021), but not in complex tasks.
Information types
Expected information types. Every information type is needed in tasks of every complexity category but the differences between different information types are really clear. Based on the participants' expectations, they would need most frequently facts in simple tasks and by far mostly information aggregates in complex tasks (see Figure 4). The differences between information types are statistically significant in complex tasks (Friedman, p=0.001), but not in other groups. Task complexity correlates significantly with the number of expected facts (Pearson's r -0.35, p=0.007) and information aggregates (Pearson's r 0.32, p=0.014). Task complexity also correlates with the share of facts (Pearson's r -0.41, p=0.001) and the share of information aggregates (Pearson's r 0.29, p=0.026). Subsequently, the more complex the task, the less facts and the more information aggregates are expected. Nonetheless, the differences between the use of known items in different complexity categories are not significant (Kruskal Wallis, p=0.186). Known items are most used in semi-complex tasks, and in complex and simple tasks they are used less but quite equally.
Dropped initial information types. Visually, it appears that the more complex the task, the smaller the share of dropped initial facts compared to all dropped information types (see Figure 4, right side). Nevertheless, neither the differences between information types nor between task complexity categories are significant. The expected use of information types and dropped initial information types are summarised in Figure 4/
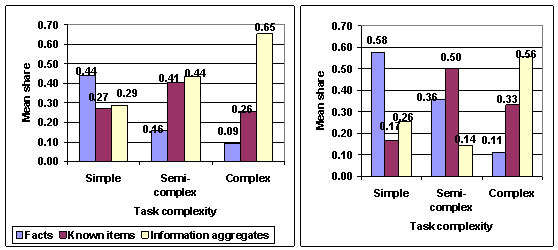
Figure 4: The share of expected use of information types (left) and dropped, initial information types (right).
Materialised information types. The materialised use of different information types looks in outline similar to the expectations of usage. In simple tasks, facts are the most used information type and they are used more than expected. In semi-complex tasks, known items have the greatest mean share of information types. As expected, information aggregates are the most used information type in complex tasks both proportionally and absolutely. The differences between information types are statistically significant in simple (Friedman, p=0.013) and complex (Friedman, p=0.012) tasks. As already seen in the usage expectations, task complexity correlates significantly with the number of facts (Pearson's r -0.37, p=0.004) and information aggregates (Pearson's r 0.30, p=0.022) used and with their shares of all information types used in an average task, respectively (Pearson's r for facts -0.47, p=0.000, and for information aggregates 0.37, p=0.005). Hence, the more complex the task, the more information aggregates and the less facts are used.
New, unexpected information types. Task complexity also correlates with the share of new facts (Pearson's r -0.44, p=0.006) and new information aggregates (Pearson's r 0.39, p=0.018) that are needed during the task process but not expected in the beginning of the task. Accordingly, the more complex the task, the less new factual needs emerge as growing task complexity indicates smaller need for facts on the whole. On the other hand, in complex tasks all information aggregates needed cannot be accurately listed before performing the task. The materialised use of information types and unexpected information types are summarised in Figure 5.
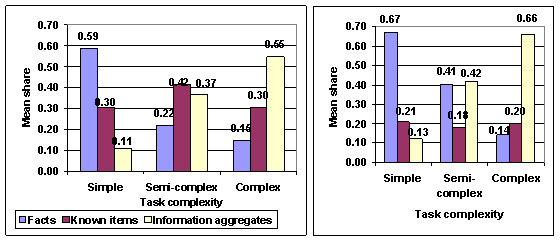
Figure 5: The share of materialised information types (left) and the share of new, unexpected information types (right).
Information type complexity. We found that task complexity correlated with information type complexity both before and especially after task performance (Table 1). It follows that the more complex the task, the more complex the information needed.
Weighting of facts, known items, and aggregates | ||||||
---|---|---|---|---|---|---|
1-1-1 | 1-2-3 | 1-5-10 | ||||
Task complexity measure | Expected | Materialised | Expected | Materialised | Expected | Materialised |
Continuous | 0.09 P | 0.21 P | 0.30* S | 0.48** S | 0.34** P | 0.50** P |
Categorical | 0.12 S | 0.26* S | 0.31* S | 0.51** S | 0.33* S | 0.54** S |
*=correlation is significant at the 0.05 level; **=correlation is significant at the 0.01 level; P=Pearson correlation; S=Spearman correlation. |
Task complexity hardly affects the number of different information types needed in a task. Instead, task complexity is obviously connected to the ascending information type complexity on ordinal scale. If known items and information aggregates are weighted heavily in comparison to facts, the correlation is even stronger.
Accessibility and sufficiency. Information needs were not as well satisfied in complex as in simpler tasks. This is demonstrated by the differences between task complexity categories that were statistically significant both concerning accessibility (Kruskal Wallis, p=0.031) and sufficiency (one-way ANOVA, p=0.042) of information. On the whole, information was both easily achievable and sufficient but the differences between information types were significant (Pearson Χ2, p=0.000). In other words, information aggregates were a little more difficult to find, and it was not so easy to obtain satisfactory information aggregates, either. On the other hand, facts were sufficient and found easily. Furthermore, the accessibility and sufficiency of known items were in between these two other information types.
Discussion
Tasks and their complexity
Participants' work roles affected task complexity: planners had more complex tasks than administrative personnel. The fact that work role did affect complexity demonstrated that the combined complexity variable was even objectively quite reliable as it handled complexity from many different viewpoints. Our results support what Leckie, Pettigrew and Sylvain (1996) state in their information seeking model: work role is a key factor affecting work tasks. They do not, however, explicitly refer to task complexity in their model. (Leckie et al. 1996).
Comparing tasks of different complexity across studies is challenging because of varying complexity criteria. Bystöm's (1999) categorisation is based on careful, qualitative analysis of several task features by the researcher; the complexity estimates of the participants played the major role but the final decision was on the researcher herself. These kinds of complexity estimates may be accurate but they are hard to repeat. Reading the representative tasks Byström (1999) gives, we can conclude that our simple tasks are mainly comparable to her information processing tasks and our complex tasks to her decision tasks, respectively. Hence, simple tasks are quick to perform and recurrent, and more complex tasks require creativity and they are longer-term projects. Though our classification is more influenced by the task performer, its simplicity makes it easy to appraise and apply.
Campbell (1988) emphasises the features of tasks at the expense of task performer's characteristics or opinion. He argues that the task performers' complexity estimates are at least indirectly influenced by task features, thus making the objective task traits more important. While we do not question such an influence, our classification is entirely founded on the task performers' views and it does not try to analyse the reasons for them. Either manner can be validated. However, if task features are to be evaluated by the researchers, they have to have a deeper insight into the substance of the tasks studied in order to classify them accurately. Additionally, Campbell's (1988) categorisation is not purely hierarchical. His complexity categorisation incorporates different sources of complexity and different task types producing a three dimensional classification that is not easily comparable to one-dimensional task categories.
Kumpulainen and Järvelin (2010) have a slightly different view of tasks, as they actually estimate complexity (the amount of prior knowledge) for data collection sessions that approximately equal tasks. This approach is practical and avoids the problem of defining the boundaries of tasks. Session complexity is determined by the researcher on the spot and therefore Kumpulainen and Järvelin's (2010) complex tasks may differ from ours although the knowledge to be estimated is partly the same. Their complexity estimates are also rigid in the sense that the participants either have the prior knowledge concerning the task or not, as judged by the researcher. We measured knowledge and complexity on a sliding scale and only afterwards applied a suitable classification scheme. This course of action had the advantage of both using the unclassified complexity estimates and enabling reclassification if needed.
Information internality
In our data, participants used mostly internal information despite of task complexity. Byström (1999) studied the same organizational setting and she found out that source internality depends on both task complexity and source type: the more complex the task, the more probable that internal people are used as sources and on the other hand, slightly more external documentary sources are used in complex than in simple tasks. We instead found that more use of internal information is expected in complex than in simple tasks, while more external information use is materialised in complex than in simple tasks. Herewith the expectations were the opposite of materialised use in terms of information internality.
One explanation could be that in simple tasks, the participants can easily name beforehand the prospective pieces of information, including external information whether needed in the performance of the actual, single task or not. As a case in point, a participant informed needing the administrative law in a routine task. It proved that she did not consult this law because she knew it already and thus did not report using it in the end of the task, either. Yet in case of complex tasks, especially the prospective external information is difficult to know beforehand and the participants itemise the familiar, i.e. internal information. We could even argue that the complexity of information seeking and vague information needs affect task complexity.
Information types and task complexity
Our categorisation for information types was grounded in the data. We did not apply any ready categories on information types used in a task. For this reason, comparing Byström's (1999) empirical results on information types to ours is only indicative. Our information types (facts, known items and information aggregates) are based on the extent or technicalities of information, whereas Byström's (1999) types (task, domain and task-solving information) are more based on the contents of the information object. With caution and to a limited extent we could argue that task information is similar to facts and domain information similar to information aggregates, because facts are often needed only in a limited task context and information aggregates can more easily be applied in a broader one, as well. Task-solving information is not applicable to our classification.
Information type use is clearly dependent on task complexity. The more complex the task, the more information aggregates and the less facts are needed. Equally, Byström (1999) found that information types are used in a certain order of importance when task complexity increases. Only narrow information is needed in simple tasks, additionally broader information in more complex tasks and even task-solving information in the most complex tasks (Byström 1999).
In our data, known items were used evenly in all task complexity categories. This may be due to the nature of known items; they are used for many different purposes from searching facts to understanding a topic (assumed to be found in a certain known-item) or from reading software manuals to delivering official records. A known item may be needed for example if all information in it may be useful (e.g., if a participant mentions a certain book title but does not refer to the purpose of use), or when a certain piece of information must be forwarded or handled regardless of the contents (e.g., filing records). A known item can be even both: a person may be told to (a) find a document (known item) to share as a copy (e.g., by e-mail) to some group of people (e.g., meeting attendees) and (b) then study its contents carefully, as well, in order to chair a meeting, for example.
The above example demonstrates that participants may refer to a known item which can actually have diverse meanings that cannot be sorted out in a short questionnaire form, for example. This may be one reason for the need for known items not being affected by task complexity. It could be argued that a participant frames and names a piece of information or an information type so that it reflects her understanding of its most important parts or uses. It goes without saying that she does not use the same conceptualisations as researchers normally do. Known items were analysed as far as possible in this study but as we focused on the conceptualisations of the participants, we desired to avoid excessive reading between the lines. It should be noted here that known items fell into known item category because they could not be put elsewhere on good grounds. This happens because people may demonstrate their information use vaguely in a questionnaire.
We found that the more complex the task, the smaller the share of dropped initial and new, unexpected facts. We can argue that to some extent, there are so many initially needed facts especially in simple tasks that it is obvious that some of them are dropped during the task process, because a fact is such a small a unit that it is more difficult to predict accurately than information aggregates. On the other hand, in complex tasks, almost 60% of dropped initial information types are information aggregates. This notion is more difficult to explain. For some reason, information aggregates tend to get switched in complex tasks as easily as facts in simple tasks, as information aggregates are also by far the most frequent new, unexpected information type in complex tasks.
Another important finding was that the more complex the task, the more complex the information needed. This result is consistent with those of Bystöm (1999). However, our study has been unable to demonstrate that the more complex the task, the more various information types needed, which was one of Byström's (1999) main findings. This is because we had different information type categories. It seems evident that people may use both task information and task solving information in a non-routine task (Byström 1999) whereas it is unclear to what extent separate facts are needed in complex tasks in addition to information aggregates. Of course they might be needed but it is not a special feature of complex tasks compared to more simple ones.
We also analysed how easily different information types were found and whether they were sufficient to satisfy the participants' information need. Differences between information types were statistically significant. Facts were well accessible and satisfactory, whereas information aggregates were more taxing to find and less satisfactory. Known items fell in between these two on both dimensions. These findings are quite obvious if we think about the nature of these two information types. Facts are easily definable and may be one or two words long; information aggregates are substantial units of information and their boundaries more difficult to delimit.
Methodological discussion
Once we had got the research permission from the city, we were not able to affect the way the participants were selected. Hence, no statistical sampling methods were used, whatsoever. The participation was voluntary and gratuitous on behalf of the participants. Arranging the sessions in concert with the participants was a necessary precaution for successful data collection as the work situations were authentic. Therefore the participants were allowed to decide the dates of data collection sessions. Accordingly, we had a convenience sample of fifty-nine tasks. This may have affected the features of the tasks. For example, the tasks may have been unusually simple because the participants wanted to manage well their work observed in the study. On the other hand, we had several, hours long sessions with each participant and thus it is unlikely that the participants were able to play some role the whole time or to select all their tasks.
The participants completed an electronic questionnaire form in the beginning and end of each task performance. This method worked well: the participants commenced to remember to complete the forms without request quite quickly and we believe that they did it carefully because they knew the researchers. This would not have been possible if we only used a questionnaire to obtain several hundreds of participants. We also saw that information seeking is such diverse a phenomenon that a single questionnaire cannot yield a thorough overview.
The limitations in the present study include the small data set. We had only fifty-nine task initiation and end form pairs but this small amount was due the fact that the data had been initially collected to be used in combination with the shadowing data (see Saastamoinen et al. 2012). We did not aim at statistical generalisability because our data set was too small and the study was not designed for that, either. However, as the participants committed themselves to shadowing and got acquainted with the researcher, it is probable that they were more motivated in completing the forms carefully than if the researcher had been anonymous or vaguely known.
Unfortunately, some of our questions were formulated ambiguously and thus the answers could not be reliably compared. However, it was more common that there was insufficient variation in the answers to compare the task complexity categories.
Conclusions
The notion of context has grown increasingly important in information studies. Tasks and their features have proved a useful context especially for work related information seeking as work usually consists of several tasks of different topics and complexity. This research examined information use related to the work tasks of a city administration. This study revealed the quantitative differences between information type use in simple, semi-complex and complex tasks and between expected and materialised use.
The results of this study indicate that a maximum of approximately a fourth of information types used are external. External information is used the least in semi-complex tasks where its share is only under 10 %. Surprisingly, participants were found to expect using more external information in simple than in complex tasks though they actually used more external information in complex tasks.
On the question of divergent information types, this study found that participants used three separate information types, namely facts, known-items and information aggregates. The use of facts clearly declined with growing task complexity, whereas the use of information aggregates increased. The use of known-items seemed independent of task complexity. Use expectations were well met in materialised use.
These findings have potentially important implications for developing information (retrieval) systems to support various work tasks. Well performing information systems can assist in any task but it is especially significant to understand the differing information needs in simple, semi-complex and complex tasks. Present systems are often designed for mainly factual information needs or otherwise simple task processes. This study confirms that the more complex the task, the more complex the information need and information use; thus flexible repositories and information systems are also needed to reinforce performing complex tasks.
Though the tasks and their features analysed in the study may not be a representative sample, we offered a simple task categorisation method that is easy to apply and that proved useful in the present setting. This kind of rough classification based on mere numerical estimations may miss some positive effects gained from qualitative task analysis but it facilitates comparing the tasks and findings across studies.
Our data must be interpreted with caution because we had a relatively small data set collected through questionnaires in a limited context and time frame. Thus more research on this topic needs to be undertaken. In addition to the information types, it is important to study the sources where information is searched for and/or found in different task complexity categories. Using several data collection methods concurrently (triangulation) and conjoining the data for analysis should improve the reliability and generalisability of results in the future.
Acknowledgements
This research was partly supported by Academy of Finland (project number 133021).
About the authors
Miamaria Saastamoinen is a postgraduate student and researcher in information studies at the School of Information Sciences, University of Tampere, Finland. Her main research interest is task-based information searching. She can be contacted at: miamaria.saastamoinen@uta.fi.
Sanna Kumpulainen is a PhD Candidate in the School of Information Sciences at University of Tampere, Finland. She received her Master in Social Sciences from the same University. Her current research interests are task-based information access and user modelling. She can be contacted at: sanna.kumpulainen@uta.fi.
Pertti Vakkari is Professor of Information Studies at the School of Information Sciences, University of Tampere, Finland. He is a member of the editorial board of journals Journal of Documentation and Information Processing & Management. His research interests include task-based information searching and use, the use of digital libraries, the evaluation of information search systems, fiction retrieval, and perceived outcomes of public libraries. His publications include several monographs and readers and over 100 papers. He has received ASIS&T SIGUSE Award for Outstanding Contributions to Information Behavior. He can be contacted at: pertti.vakkari@uta.fi.
Kalervo Järvelin, is Professor and Vice Chair at the School of Information Sciences, University of Tampere, Finland. He holds a PhD in Information Studies (1987) from the same university. His research covers information seeking and retrieval, linguistic and conceptual methods in information retrieval, information retrieval evaluation, and database management. He can be contacted at: kalervo.jarvelin@uta.fi.